Contextual Search in the Presence of Adversarial Corruptions
OPERATIONS RESEARCH(2022)
摘要
We study contextual search, a generalization of binary search in higher dimensions, which captures settings such as feature-based dynamic pricing. Standard formulations of this problem assume that agents act in accordance with a specific homogeneous response model. In practice, however, some responses may be adversarially corrupted. Existing algorithms heavily depend on the assumed response model being (approximately) accurate for all agents and have poor performance in the presence of even a few such arbitrary misspecifications. We initiate the study of contextual search when some of the agents can behave in ways inconsistent with the underlying response model. In particular, we provide two algorithms, one based on multidimensional binary search methods and one based on gradient descent. We show that these algorithms attain near-optimal regret in the absence of adversarial corruptions and their performance degrades gracefully with the number of such agents, providing the first results for contextual search in any adversarial noise model. Our techniques draw inspiration from learning theory, game theory, high dimensional geometry, and convex analysis.
更多查看译文
关键词
contextual online decision making,dynamic pricing,learning from revealed preferences
AI 理解论文
溯源树
样例
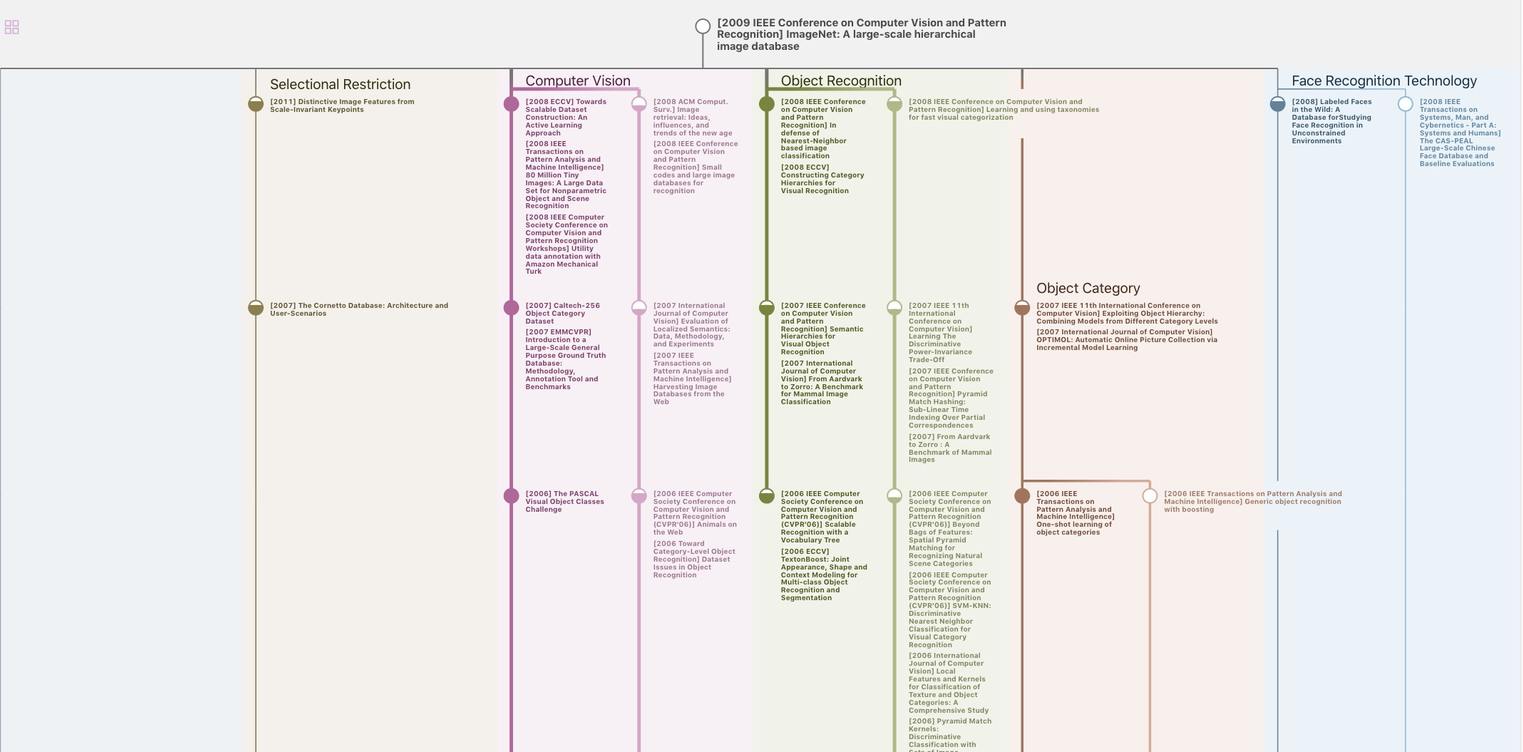
生成溯源树,研究论文发展脉络
Chat Paper
正在生成论文摘要