Learning Accurate Label-Specific Features From Partially Multilabeled Data
IEEE TRANSACTIONS ON NEURAL NETWORKS AND LEARNING SYSTEMS(2023)
摘要
Feature selection is an effective dimensionality reduction technique, which can speed up an algorithm and improve model performance such as predictive accuracy and result comprehensibility. The study of selecting label-specific features for each class label has attracted considerable attention since each class label might be determined by some inherent characteristics, where precise label information is required to guide label-specific feature selection. However, obtaining noise-free labels is quite difficult and impractical. In reality, each instance is often annotated by a candidate label set that comprises multiple ground-truth labels and other false-positive labels, termed partial multilabel (PML) learning scenario. Here, false-positive labels concealed in a candidate label set might induce the selection of false label-specific features while masking the intrinsic label correlations, which misleads the selection of relevant features and compromises the selection performance. To address this issue, a novel two-stage partial multilabel feature selection (PMLFS) approach is proposed, which elicits credible labels to guide accurate label-specific feature selection. First, the label confidence matrix is learned to help elicit ground-truth labels from the candidate label set via the label structure reconstruction strategy, each element of which indicates how likely a class label is ground truth. After that, based on distilled credible labels, a joint selection model, including label-specific feature learner and common feature learner, is designed to learn accurate label-specific features to each class label and common features for all class labels. Besides, label correlations are fused into the features selection process to facilitate the generation of an optimal feature subset. Extensive experimental results clearly validate the superiority of the proposed approach.
更多查看译文
关键词
Feature extraction,Correlation,Task analysis,Noise measurement,Sports,Indexes,Data mining,Feature selection,label correlations,label disambiguation,label-specific features,partial multilabel (PML) learning
AI 理解论文
溯源树
样例
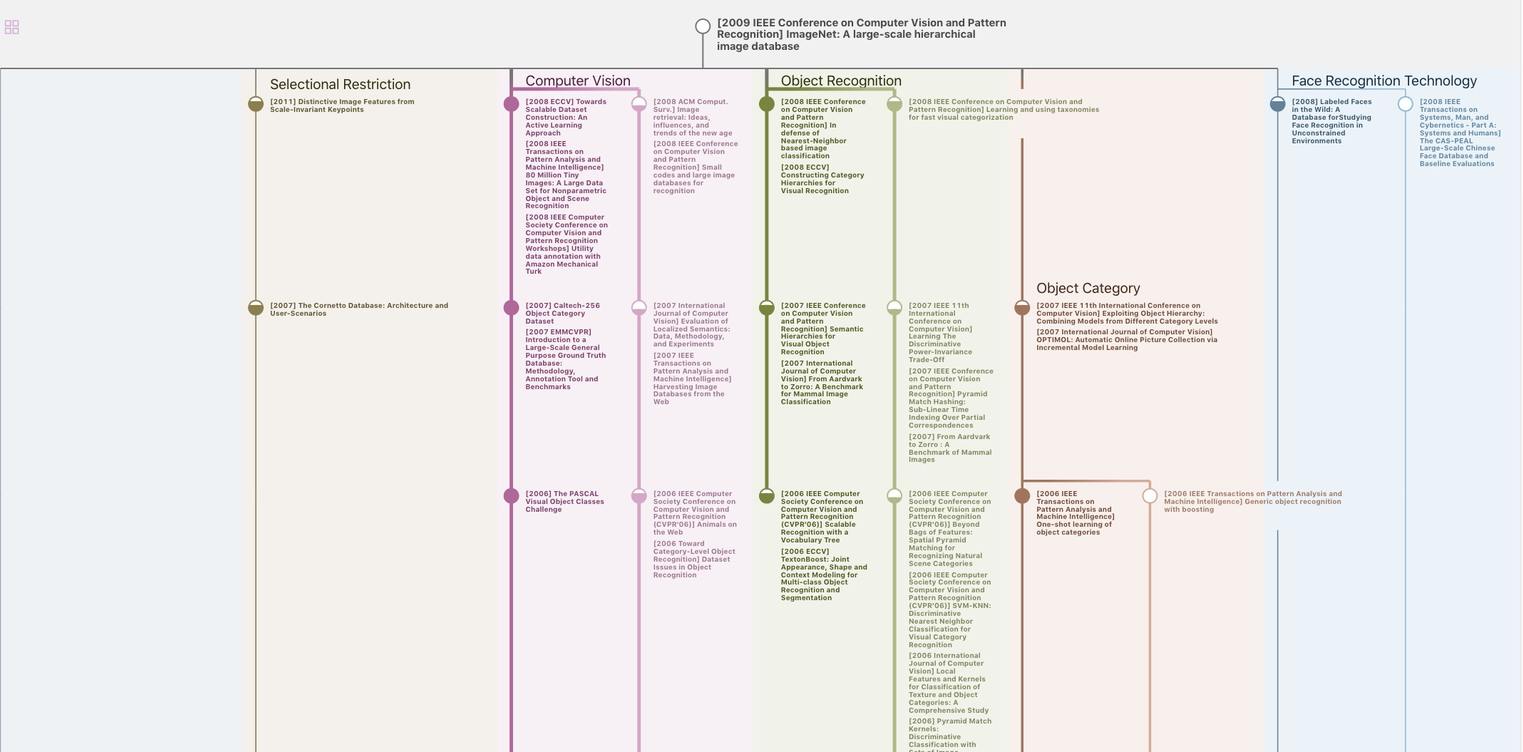
生成溯源树,研究论文发展脉络
Chat Paper
正在生成论文摘要