DeepAccident: A Motion and Accident Prediction Benchmark for V2X Autonomous Driving
AAAI 2024(2024)
摘要
Safety is the primary priority of autonomous driving. Nevertheless, no published dataset currently supports the direct and explainable safety evaluation for autonomous driving. In this work, we propose DeepAccident, a large-scale dataset generated via a realistic simulator containing diverse accident scenarios that frequently occur in real-world driving. The proposed DeepAccident dataset includes 57K annotated frames and 285K annotated samples, approximately 7 times more than the large-scale nuScenes dataset with 40k annotated samples. In addition, we propose a new task, end-to-end motion and accident prediction, which can be used to directly evaluate the accident prediction ability for different autonomous driving algorithms. Furthermore, for each scenario, we set four vehicles along with one infrastructure to record data, thus providing diverse viewpoints for accident scenarios and enabling V2X (vehicle-to-everything) research on perception and prediction tasks. Finally, we present a baseline V2X model named V2XFormer that demonstrates superior performance for motion and accident prediction and 3D object detection compared to the single-vehicle model.
更多查看译文
关键词
CV: Vision for Robotics & Autonomous Driving,CV: 3D Computer Vision,CV: Adversarial Attacks & Robustness,CV: Motion & Tracking,ROB: Multimodal Perception & Sensor Fusion,ROB: Multi-Robot Systems
AI 理解论文
溯源树
样例
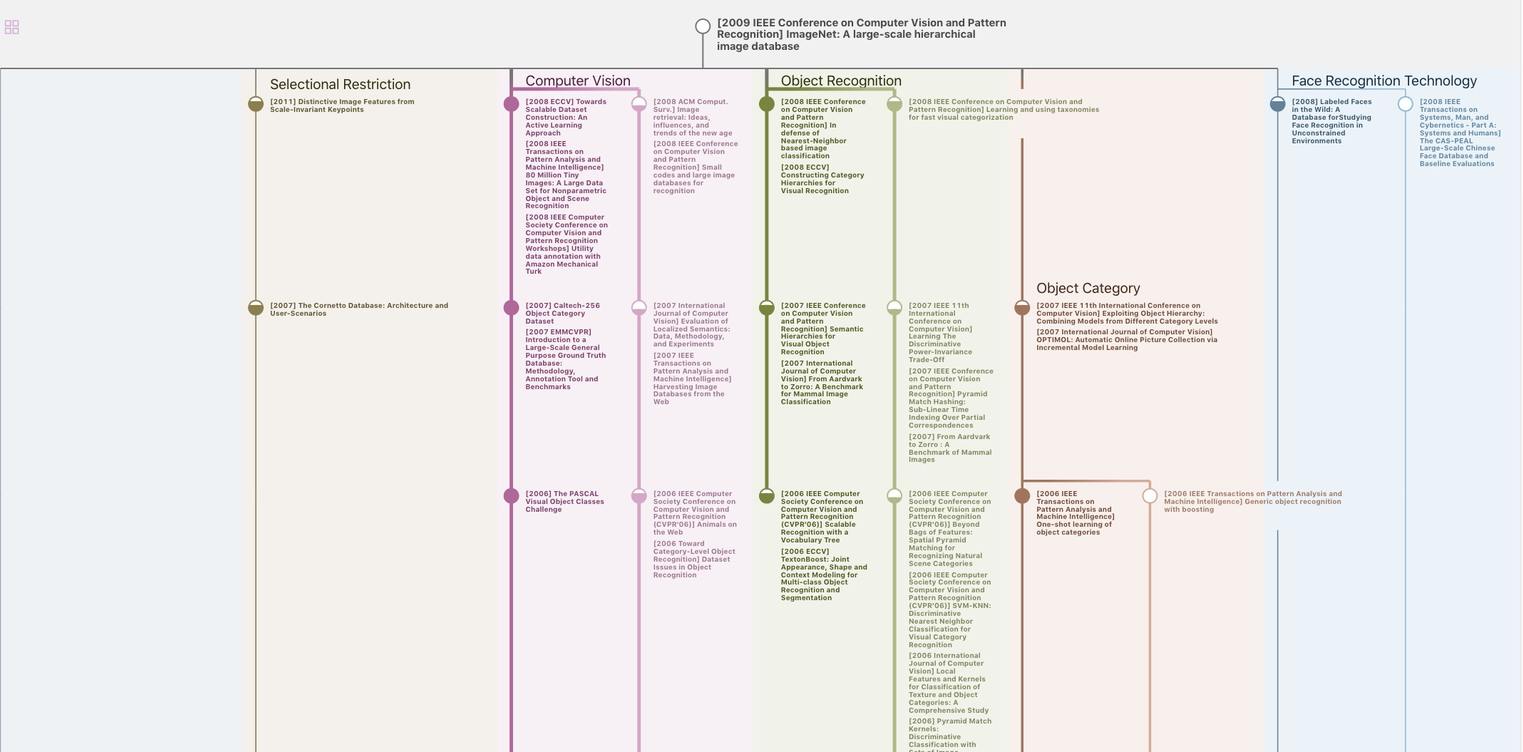
生成溯源树,研究论文发展脉络
Chat Paper
正在生成论文摘要