Safe Reinforcement Learning for LiDAR-based Navigation via Control Barrier Function.
ICMLA(2022)
摘要
Reinforcement learning has shown encouraging results in decision-making tasks such as those found in gaming and robotics. However, when applied in safety-critical applications, like navigation, reinforcement learning can cause serious safety problems due to its trial-and-error nature. In this paper, we propose a safe reinforcement learning framework for LiDAR-based navigation. The proposed framework incorporates control barrier function theory with reinforcement learning to mitigate safety risks while learning a navigation strategy. Furthermore, by designing an online learning control barrier function, the proposed method can adapt to different obstacle settings through learning from past experiences. As a map-less solution, our approach uses only LiDAR as the perception system and requires no additional localization information. We conduct simulated experiments in ROS Gazebo under different indoor environments. The results show that our method outperforms other approaches by achieving up to twice the reward and five times fewer collisions when compared to the runner-up.
更多查看译文
关键词
Automatous Navigation,Deep Reinforcement Learning,LiDAR based,Control Barrier Function
AI 理解论文
溯源树
样例
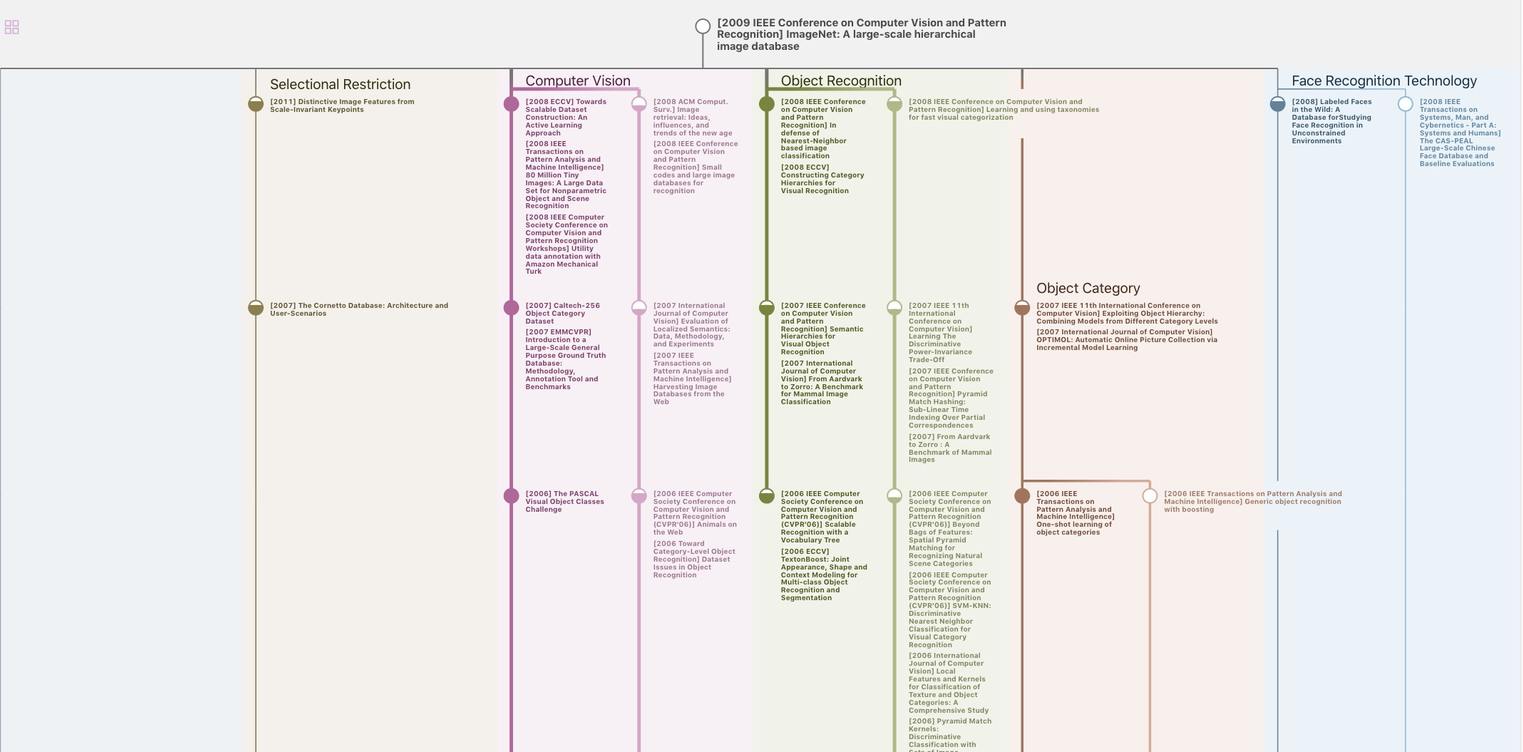
生成溯源树,研究论文发展脉络
Chat Paper
正在生成论文摘要