NatUKE: A Benchmark for Natural Product Knowledge Extraction from Academic Literature.
ICSC(2023)
摘要
This work introduces a benchmark for natural product knowledge extraction from academic literature and evaluates different, state-of-the-art unsupervised embedding generation methods for this task. We show that it can automatically extract chemical compound characteristics from academic literature with an unsupervised pipeline based on graph embedding methods. We evaluated Four methods (DeepWalk, Node2Vec, Metapath2Vec, and EPHEN) in a similarity-based graph completion evaluation scenario. EPHEN achieves reasonable hits@k performance at bioactivity and isolation type extraction with 0.64 when k = 5 and 0.75 when k = 1, respectively. Meanwhile, Metapath2Vec was the best performer, but with underwhelming results, when extracting compound name and specie with 0.20 and 0.44 when k = 50, respectively. These results show that using text data and previously extracted knowledge from the knowledge graph provides the most stable performance. They also show us that some characteristics from these papers are more challenging to extract than others, and using the knowledge graph topology as context data helps in these scenarios.
更多查看译文
关键词
knowledge extraction,natural products,knowledge graphs
AI 理解论文
溯源树
样例
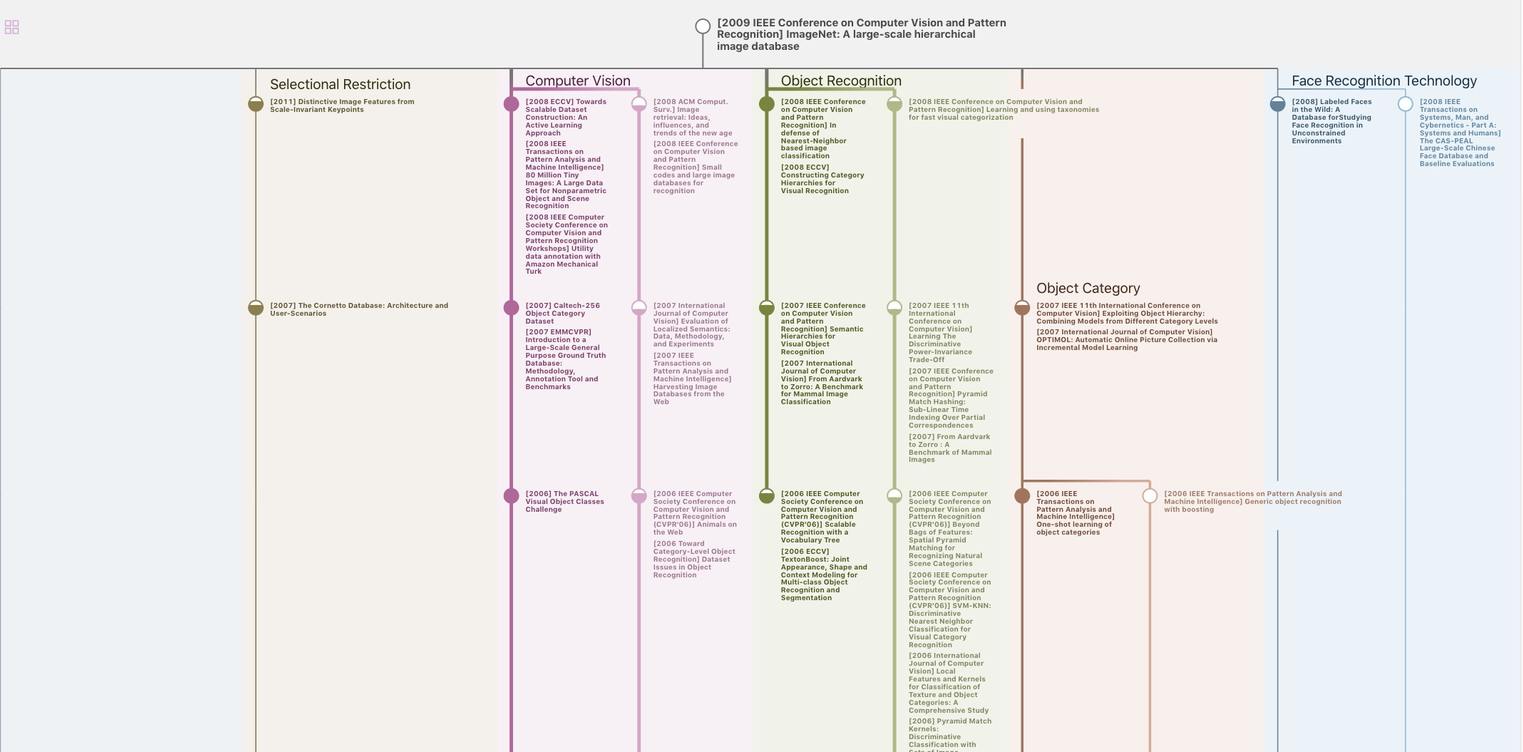
生成溯源树,研究论文发展脉络
Chat Paper
正在生成论文摘要