TraVL: Transferring Pre-trained Visual-Linguistic Models for Cross-Lingual Image Captioning.
APWeb/WAIM (2)(2022)
摘要
Visual-Linguistic (VL) pre-training is gaining increasing interest due to its ability to learn generic VL representations that can be used for downstream cross-modal tasks. However, the lack of large-scale and high-quality parallel corpora makes VL pre-training impractical for low-resource languages. Therefore, it is desirable to leverage existing well-trained English VL models for cross-modal tasks in other languages. But a basic approach suffers from its inability to capture the semantic correlation between different modalities and insufficient utilization of the hierarchical representations of VL models. In this work, we propose TraVL, a novel framework for transferring pre-trained VL models for cross-lingual image captioning. To enforce the semantic alignment during modality fusion, TraVL employs joint attention that constructs the key-value pair by concatenating the visual and linguistic representations. To fully exploit the hierarchical visual information, we develop an adjacent layer-fusion mechanism that allows each decoder layer to attend to the encoder’s multilayer representations with similar semantics. Experiments on a Chinese image-text dataset show that TraVL outperforms state-of-the-art captioning models and other transfer learning methods.
更多查看译文
关键词
pre-trained,visual-linguistic,cross-lingual
AI 理解论文
溯源树
样例
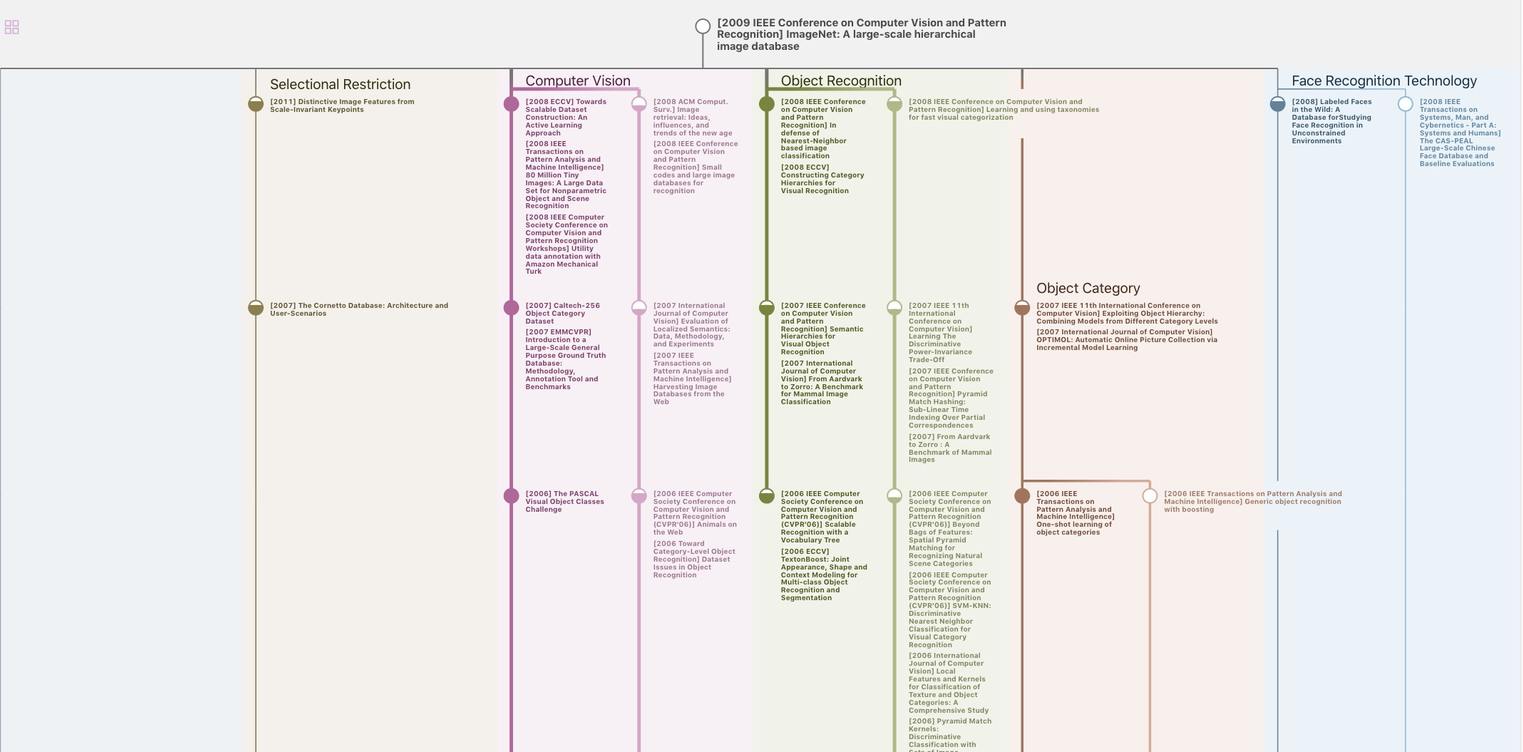
生成溯源树,研究论文发展脉络
Chat Paper
正在生成论文摘要