ParaGraph: Mapping Wikidata Tail Entities to Wikipedia Paragraphs.
Big Data(2022)
摘要
Bridging unstructured data with knowledge bases is an essential task in many problems related to natural language understanding. Traditionally, this task is considered in one direction only: linking entity mentions in a text to their counterpart in a knowledge base (also known as entity linking). In this paper, we propose to tackle this problem from a different angle: linking entities from a knowledge base to paragraphs describing those entities. We argue that such a new perspective can be beneficial to several applications, including information retrieval, knowledge base population, and joint entity and word embedding. We present a transformer-based model, ParaGraph, which, given a Wikidata entity as input, retrieves its corresponding Wikipedia section. To perform this task, ParaGraph first generates an entity summary and compares it to sections to select an initial set of candidates. The candidates are then ranked using additional information from the entity’s textual description and contextual information. Our experimental results show that ParaGraph achieves 87% Hits@10 when ranking Wikipedia sections given a Wikidata entity as input. The obtained results show that ParaGraph can reduce the information gap between Wikipedia-based entities and tail entities and demonstrate the effectiveness of our proposed approach towards linking knowledge graph entities to their text counterparts.
更多查看译文
关键词
mapping wikidata tail entities
AI 理解论文
溯源树
样例
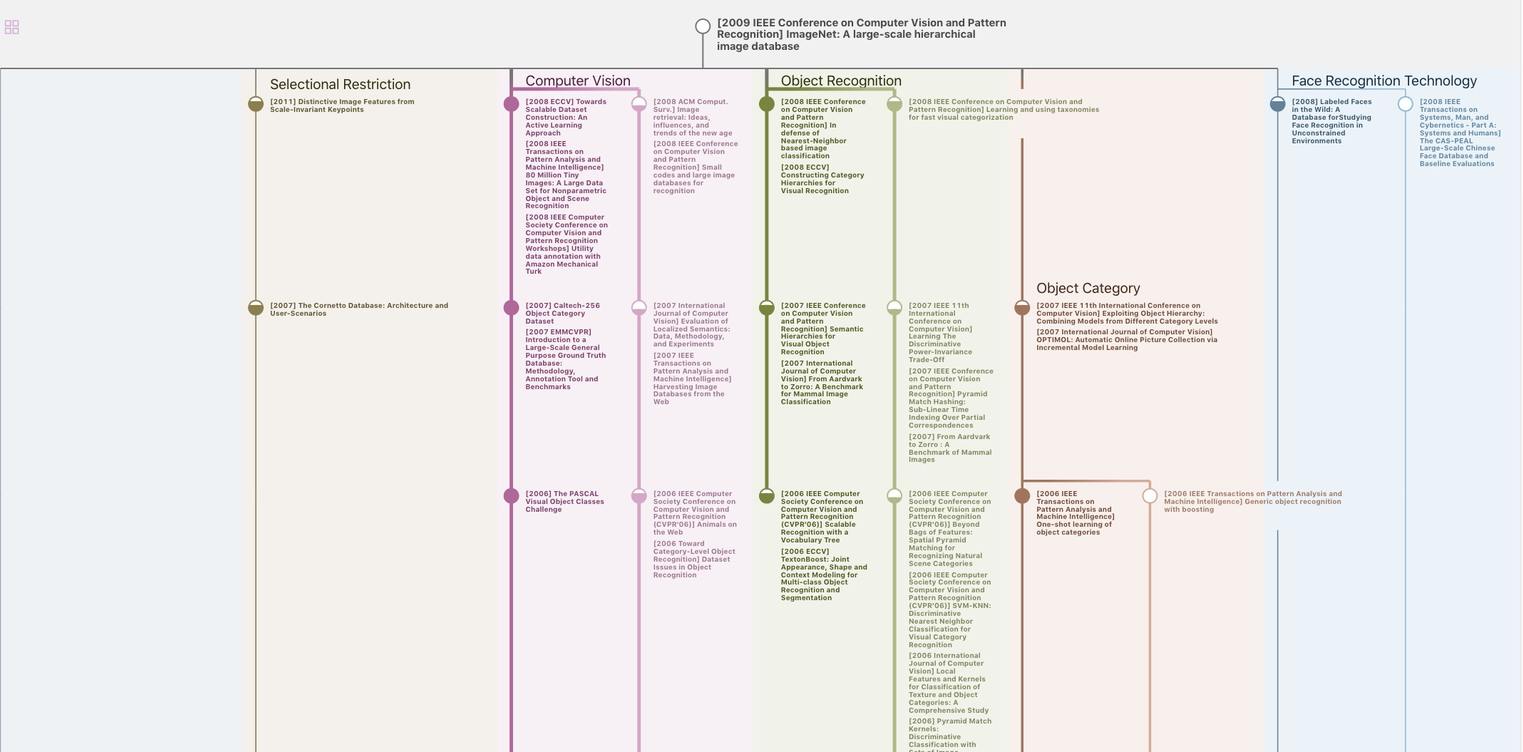
生成溯源树,研究论文发展脉络
Chat Paper
正在生成论文摘要