Uncertainty Calibration for Counterfactual Propensity Estimation in Recommendation
arxiv(2023)
摘要
In recommendation systems, a large portion of the ratings are missing due to the selection biases, which is known as Missing Not At Random. The counterfactual inverse propensity scoring (IPS) was used to weight the imputation error of every observed rating. Although effective in multiple scenarios, we argue that the performance of IPS estimation is limited due to the uncertainty miscalibration of propensity estimation. In this paper, we propose the uncertainty calibration for the propensity estimation in recommendation systems with multiple representative uncertainty calibration techniques. Theoretical analysis on the bias and generalization bound shows the superiority of the calibrated IPS estimator over the uncalibrated one. Experimental results on the coat and yahoo datasets shows that the uncertainty calibration is improved and hence brings the better recommendation results.
更多查看译文
关键词
counterfactual propensity estimation,uncertainty calibration,recommendation
AI 理解论文
溯源树
样例
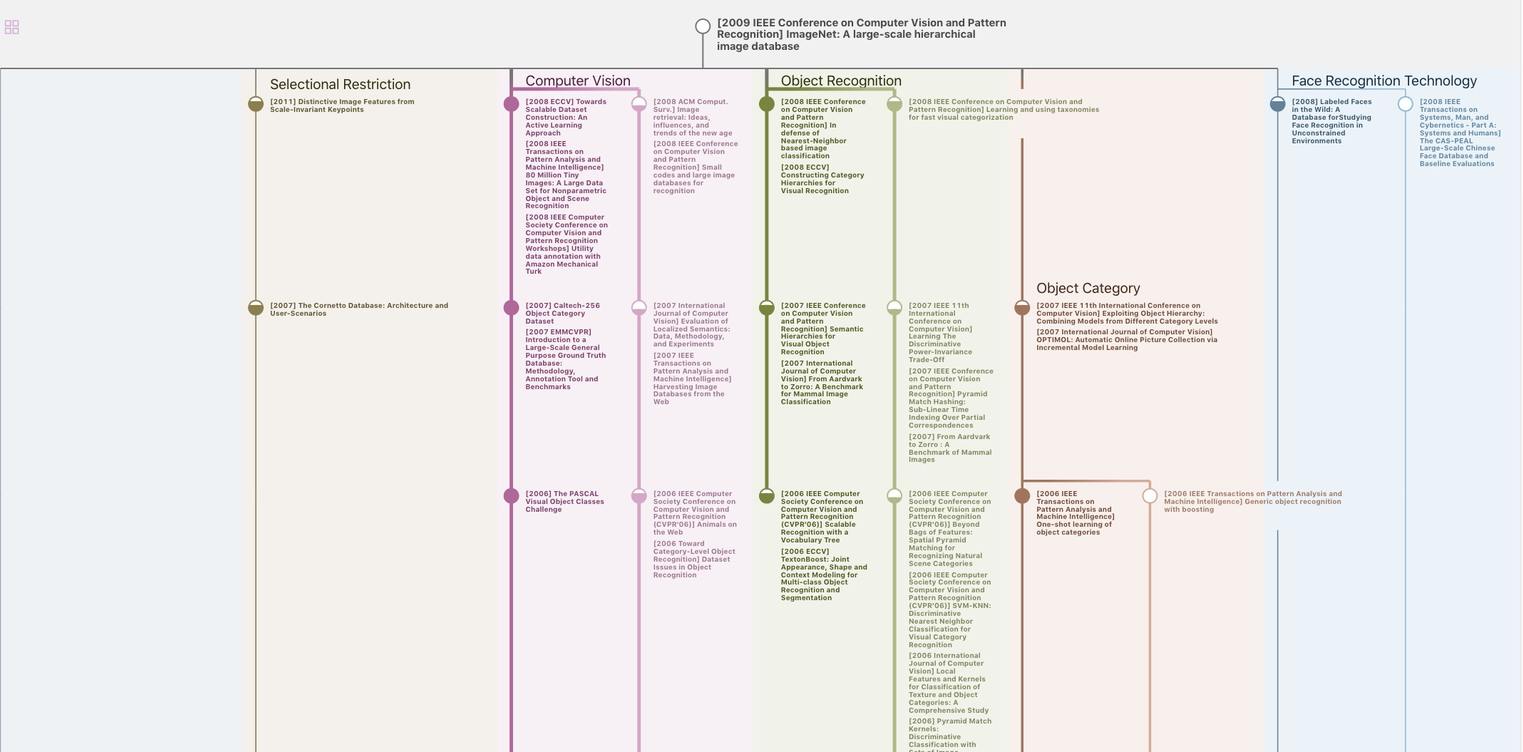
生成溯源树,研究论文发展脉络
Chat Paper
正在生成论文摘要