Cross-Scale KNN Image Transformer for Image Restoration.
IEEE Access(2023)
摘要
Numerous image restoration approaches have been proposed based on attention mechanism, achieving superior performance to convolutional neural networks (CNNs) based counterparts. However, they do not leverage the attention model in a form fully suited to the image restoration tasks. In this paper, we propose an image restoration network with a novel attention mechanism, called cross-scale $k$ -NN image Transformer (CS-KiT), that effectively considers several factors such as locality, non-locality, and cross-scale aggregation, which are essential to image restoration. To achieve locality and non-locality, the CS-KiT builds $k$ -nearest neighbor relation of local patches and aggregates similar patches through local attention. To induce cross-scale aggregation, we ensure that each local patch embraces different scale information with scale-aware patch embedding (SPE) which predicts an input patch scale through a combination of multi-scale convolution branches. We show the effectiveness of the CS-KiT with experimental results, outperforming state-of-the-art restoration approaches on image denoising, deblurring, and deraining benchmarks.
更多查看译文
关键词
Image restoration,Transformers,Noise reduction,Complexity theory,Computer vision,Convolutional neural networks,Feature extraction,denoising,deblurring,deraining,transformer,self-attention,k-nn search,low-level vision
AI 理解论文
溯源树
样例
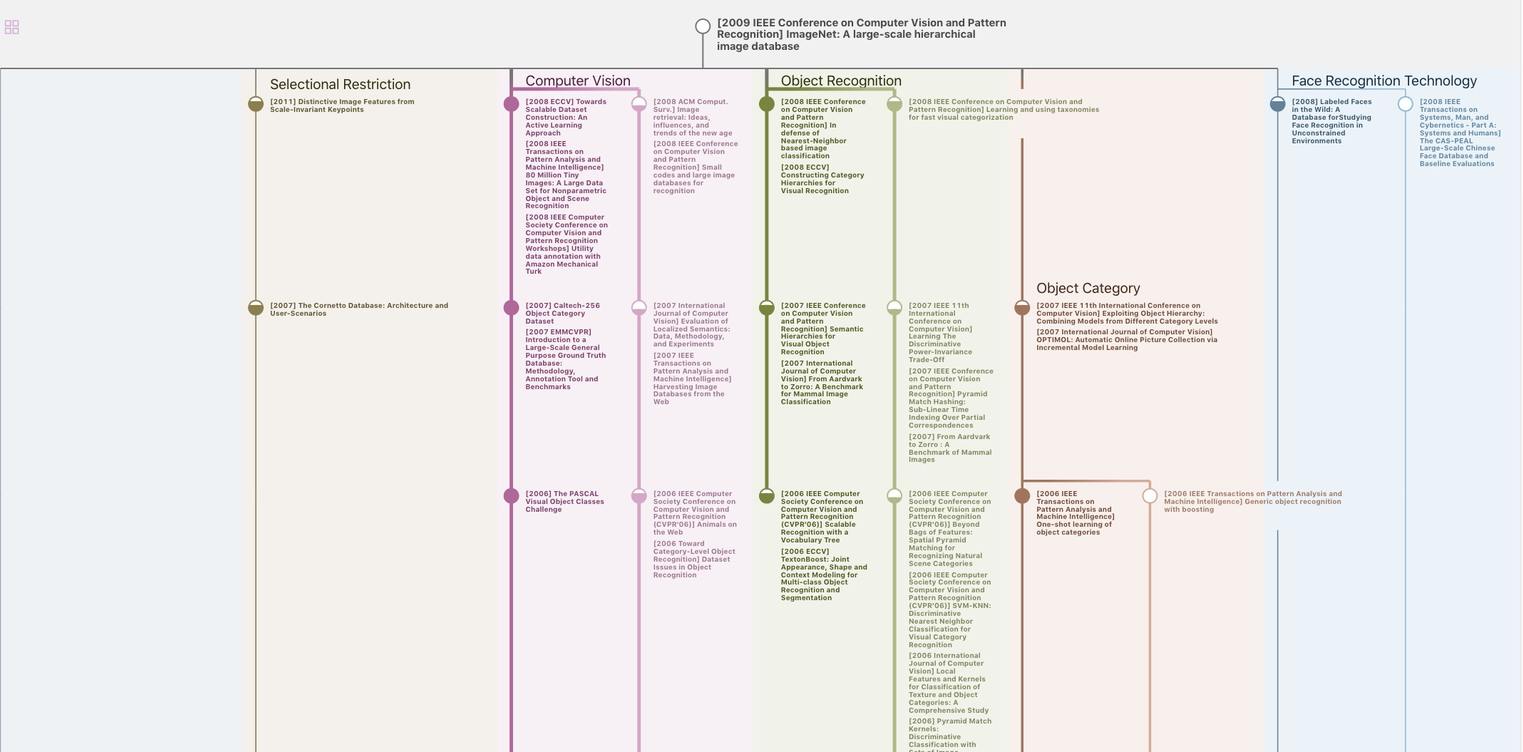
生成溯源树,研究论文发展脉络
Chat Paper
正在生成论文摘要