Uncertainty-Aware Off-Policy Learning
arxiv(2023)
摘要
Off-policy learning, referring to the procedure of policy optimization with access only to logged feedback data, has shown importance in various real-world applications, such as search engines, recommender systems, and etc. While the ground-truth logging policy, which generates the logged data, is usually unknown, previous work simply takes its estimated value in off-policy learning, ignoring both high bias and high variance resulted from such an estimator, especially on samples with small and inaccurately estimated logging probabilities. In this work, we explicitly model the uncertainty in the estimated logging policy and propose a Uncertainty-aware Inverse Propensity Score estimator (UIPS) for improved off-policy learning. Experiment results on synthetic and three real-world recommendation datasets demonstrate the advantageous sample efficiency of the proposed UIPS estimator against an extensive list of state-of-the-art baselines.
更多查看译文
关键词
off-policy learning,uncertainty
AI 理解论文
溯源树
样例
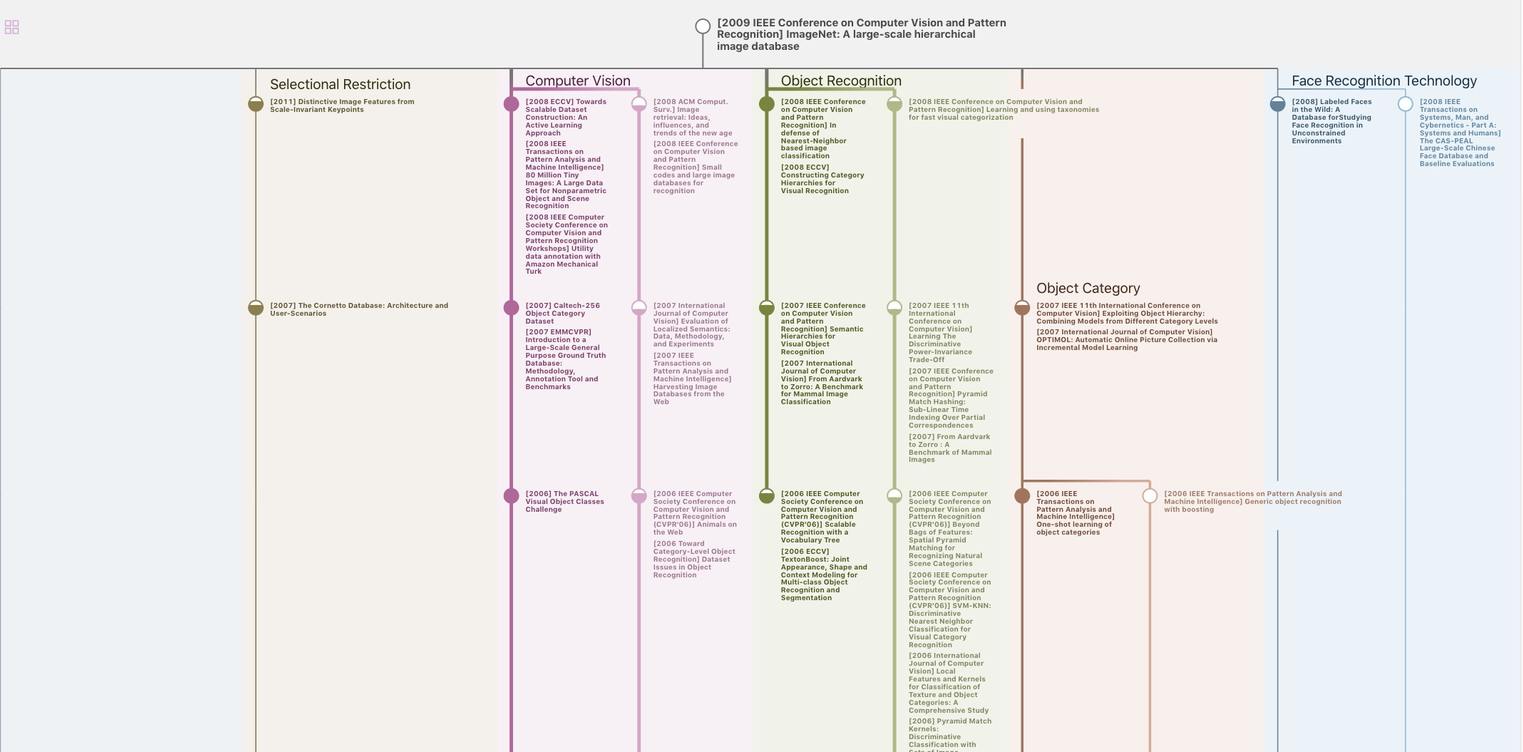
生成溯源树,研究论文发展脉络
Chat Paper
正在生成论文摘要