Learning Exploration Strategies to Solve Real-World Marble Runs
arxiv(2023)
摘要
Tasks involving locally unstable or discontinuous dynamics (such as bifurcations and collisions) remain challenging in robotics, because small variations in the environment can have a significant impact on task outcomes. For such tasks, learning a robust deterministic policy is difficult. We focus on structuring exploration with multiple stochastic policies based on a mixture of experts (MoE) policy representation that can be efficiently adapted. The MoE policy is composed of stochastic sub-policies that allow exploration of multiple distinct regions of the action space (or strategies) and a high-level selection policy to guide exploration towards the most promising regions. We develop a robot system to evaluate our approach in a real-world physical problem solving domain. After training the MoE policy in simulation, online learning in the real world demonstrates efficient adaptation within just a few dozen attempts, with a minimal sim2real gap. Our results confirm that representing multiple strategies promotes efficient adaptation in new environments and strategies learned under different dynamics can still provide useful information about where to look for good strategies.
更多查看译文
关键词
exploration,strategies,learning,real-world
AI 理解论文
溯源树
样例
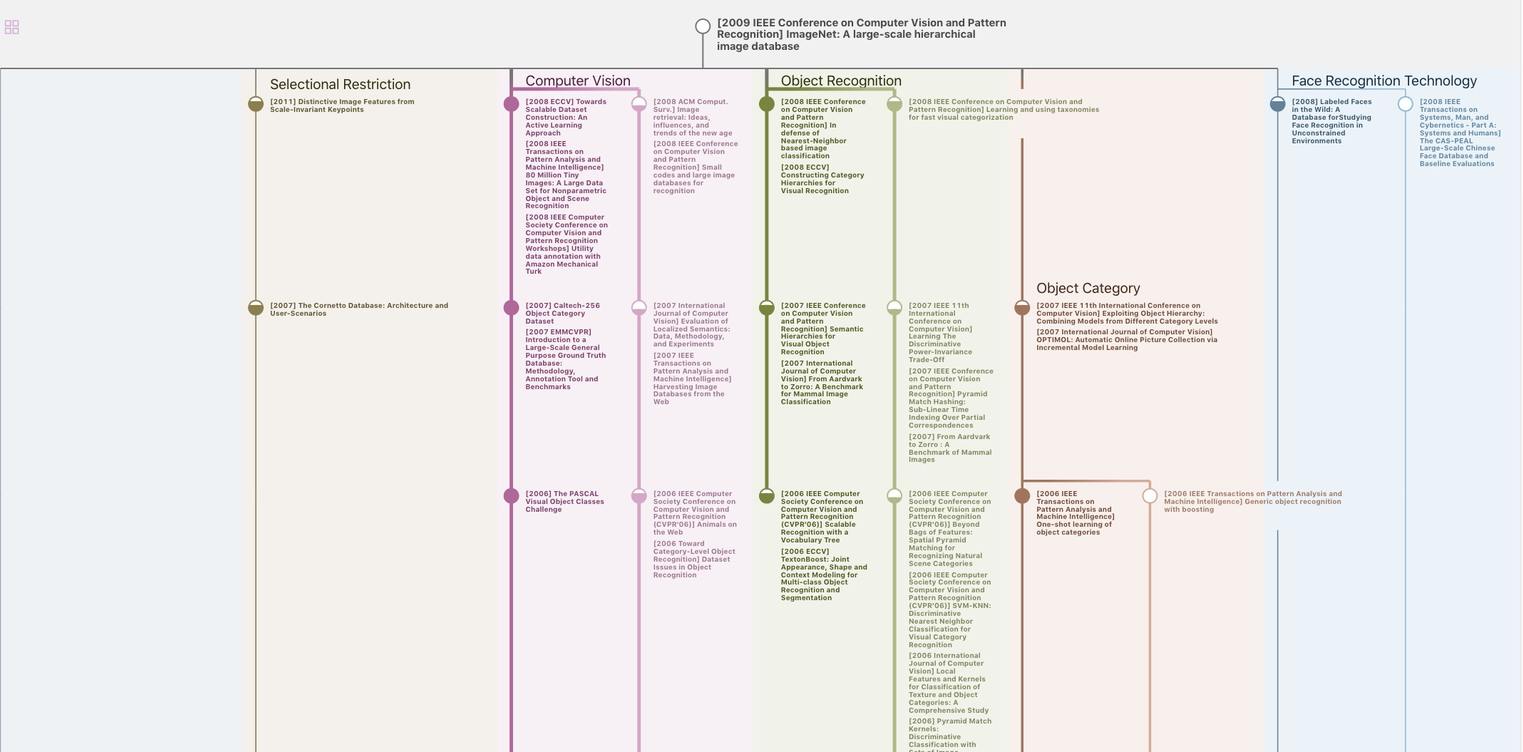
生成溯源树,研究论文发展脉络
Chat Paper
正在生成论文摘要