Visual Analytics of Neuron Vulnerability to Adversarial Attacks on Convolutional Neural Networks
ACM TRANSACTIONS ON INTERACTIVE INTELLIGENT SYSTEMS(2023)
摘要
Adversarial attacks on a convolutional neural network (CNN)-injecting human-imperceptible perturbations into an input image-could fool a high-performance CNN into making incorrect predictions. The success of adversarial attacks raises serious concerns about the robustness of CNNs, and prevents them from being used in safety-critical applications, such asmedical diagnosis and autonomous driving. Ourwork introduces a visual analytics approach to understanding adversarial attacks by answering two questions: (1) Which neurons are more vulnerable to attacks? and (2) Which image features do these vulnerable neurons capture during the prediction? For the first question, we introduce multiple perturbation-based measures to break down the attacking magnitude into individual CNN neurons and rank the neurons by their vulnerability levels. For the second, we identify image features (e.g., cat ears) that highly stimulate a user-selected neuron to augment and validate the neuron's responsibility. Furthermore, we support an interactive exploration of a large number of neurons by aiding with hierarchical clustering based on the neurons' roles in the prediction. To this end, a visual analytics system is designed to incorporate visual reasoning for interpreting adversarial attacks. We validate the effectiveness of our system through multiple case studies aswell as feedback from domain experts.
更多查看译文
关键词
Convolutional neural networks,adversarial attack,explainable machine learning
AI 理解论文
溯源树
样例
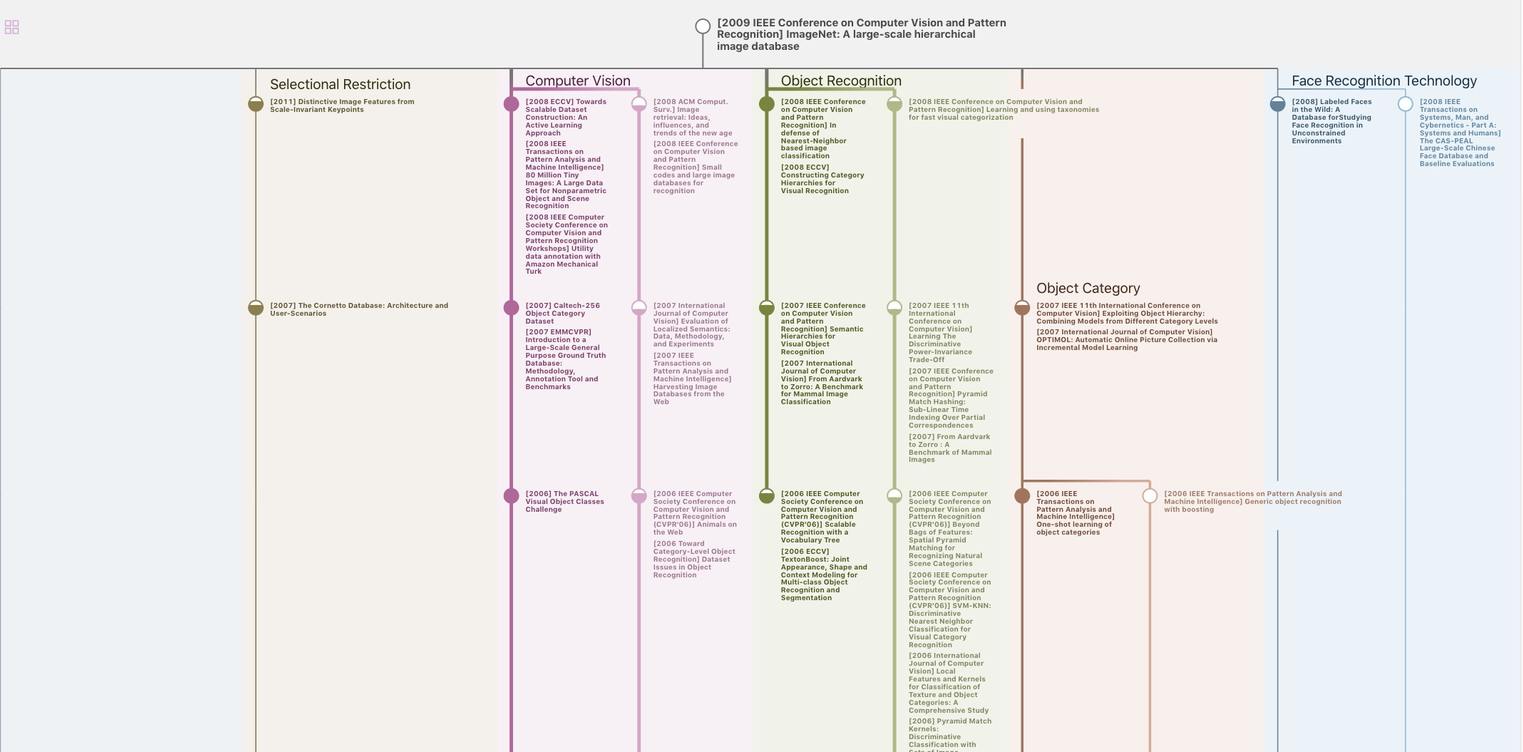
生成溯源树,研究论文发展脉络
Chat Paper
正在生成论文摘要