Epileptic EEG Identification Based on Dual Q -Factor Signal Decomposition (DQSD), Fast and Adaptive Multivariate Empirical Mode Decomposition (FA-MVEMD) and Neural Networks
CIRCUITS SYSTEMS AND SIGNAL PROCESSING(2023)
摘要
Epileptic seizure is a chronic central nervous system disorder which is characterized by transient event of abnormal and excessive neuronal discharge in the brain. Electroencephalogram (EEG) records the neurological activity in the brain which is commonly used for the identification of epilepsy. However, visual screening of EEG recordings is time consuming, tedious and subjective. Hence, automatic seizure detection becomes urgent in clinical application. Relevant technique in this filed has been developed rapidly with the employment of machine learning and signal processing tools. In this study, we propose a novel method for automatic epileptic EEG identification based upon dual Q -factor signal decomposition derived from tunable Q -factor wavelet transform (TQWT), fast and adaptive multivariate empirical mode decomposition (FA-MVEMD) and neural networks. First, EEG signals are decomposed into high- and low-resonance components using both high-Q-factor and low-Q-factor TQWT jointly. Second, FA-MVEMD decomposes the high- and low- Q -factor components into scale-aligned intrinsic mode components (IMFs). The first two IMFs of the high- and low- Q -factor components are extracted, which contain most of the EEG signals’ energy and are considered to be the predominant IMFs. The properties associated with the nonlinear EEG system dynamics are preserved in the predominant IMFs which demonstrate significant difference in EEG system dynamics between normal (healthy), interictal and ictal EEG signals. Third, neural networks are then used to model, identify and classify EEG system dynamics with the predominant IMFs as the input. Finally, experiments on Bonn EEG database are included to assess the effectiveness of the proposed method. The overall average accuracy for binary classification, ternary classification and five-class classification is reported to be 98.54% , 99.18% and 97.84% , respectively, by using tenfold cross-validation. In comparison with other state-of-the-art methods, our algorithm illustrates superior performance, which can serve as a potential candidate for the automatic EEG seizure detection in the clinical application.
更多查看译文
关键词
Dual Q -factor signal decomposition (DQSD),Tunable Q -factor wavelet transform (TQWT)
AI 理解论文
溯源树
样例
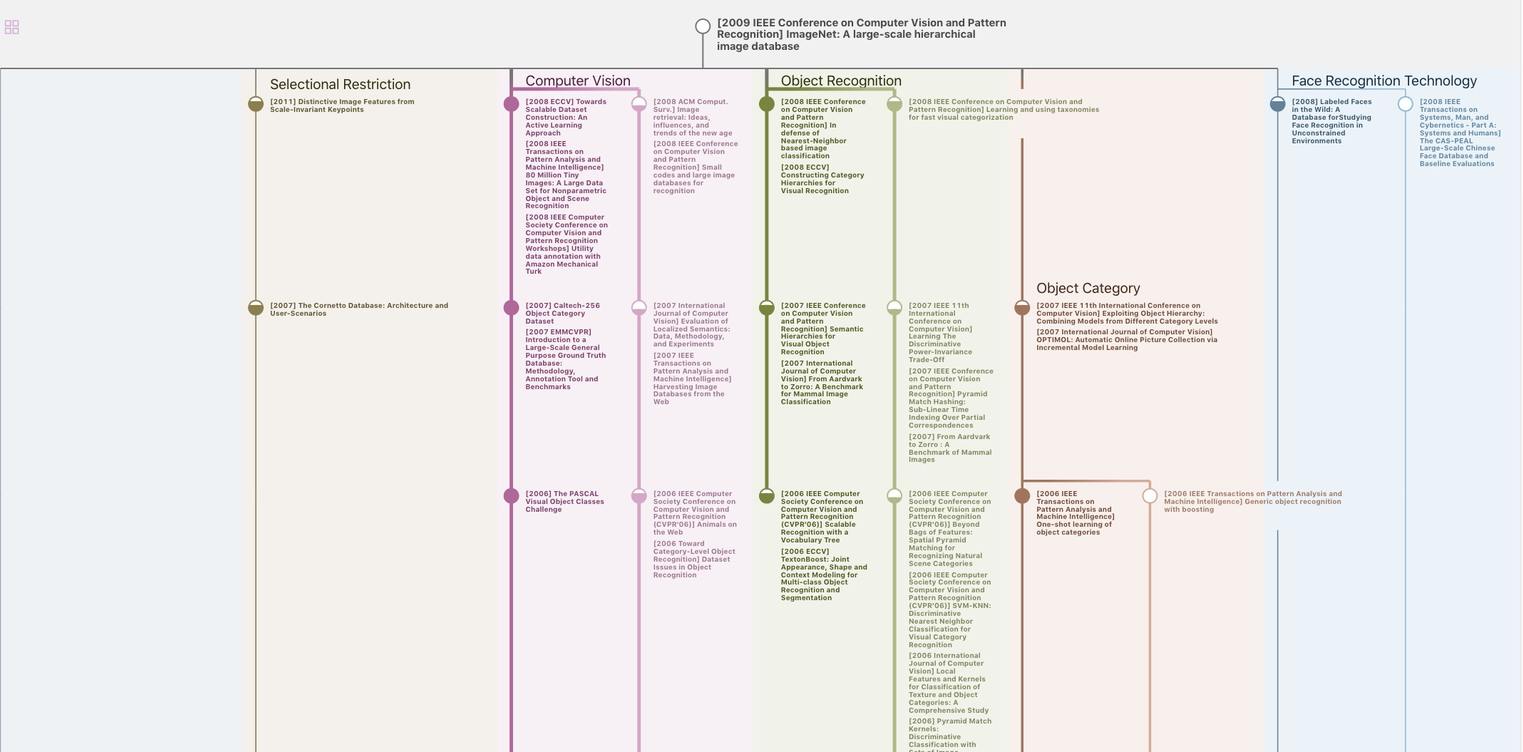
生成溯源树,研究论文发展脉络
Chat Paper
正在生成论文摘要