On Differentially Private Online Predictions
arxiv(2023)
摘要
In this work we introduce an interactive variant of joint differential privacy towards handling online processes in which existing privacy definitions seem too restrictive. We study basic properties of this definition and demonstrate that it satisfies (suitable variants) of group privacy, composition, and post processing. We then study the cost of interactive joint privacy in the basic setting of online classification. We show that any (possibly non-private) learning rule can be effectively transformed to a private learning rule with only a polynomial overhead in the mistake bound. This demonstrates a stark difference with more restrictive notions of privacy such as the one studied by Golowich and Livni (2021), where only a double exponential overhead on the mistake bound is known (via an information theoretic upper bound).
更多查看译文
关键词
private online predictions
AI 理解论文
溯源树
样例
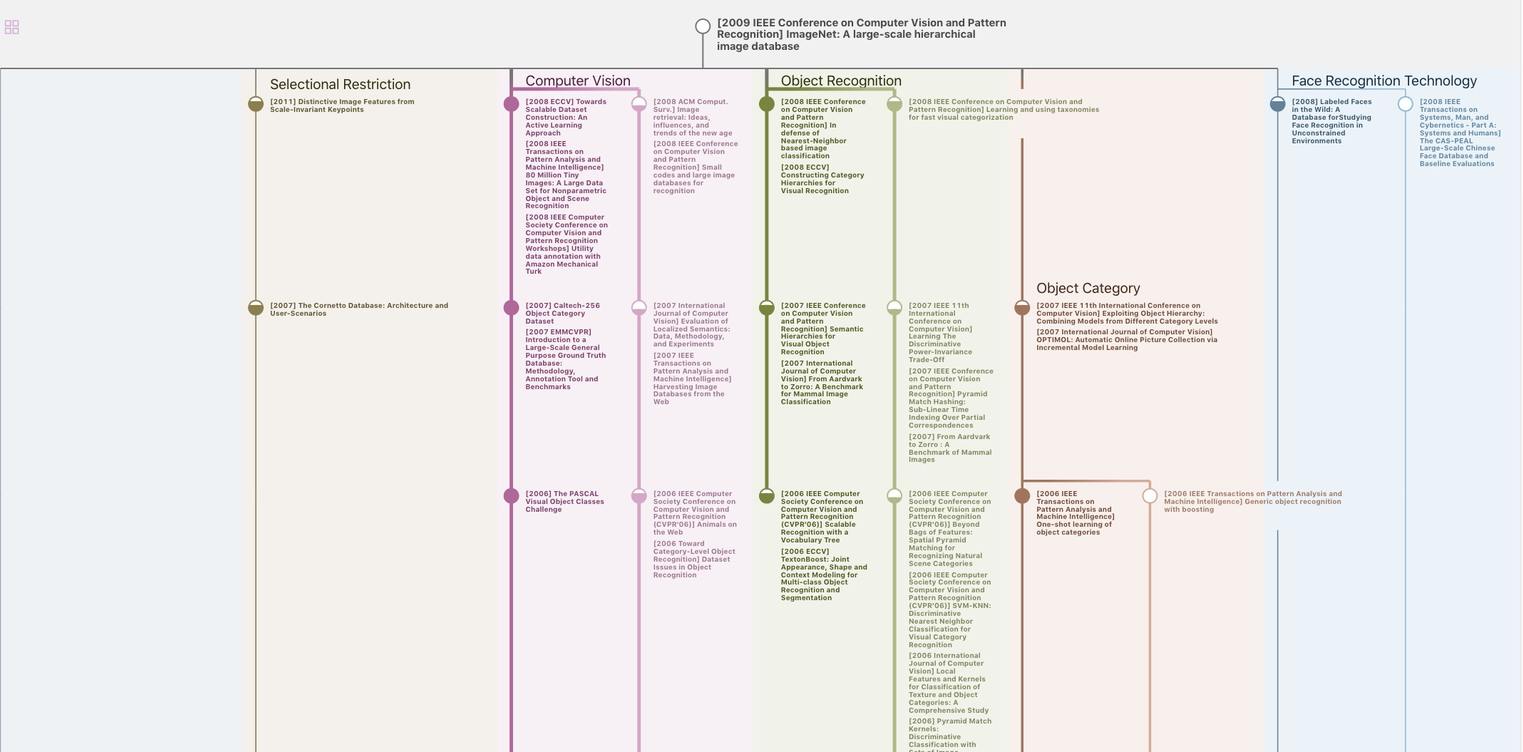
生成溯源树,研究论文发展脉络
Chat Paper
正在生成论文摘要