Asymptotically Unbiased Off-Policy Policy Evaluation when Reusing Old Data in Nonstationary Environments
arxiv(2023)
摘要
In this work, we consider the off-policy policy evaluation problem for contextual bandits and finite horizon reinforcement learning in the nonstationary setting. Reusing old data is critical for policy evaluation, but existing estimators that reuse old data introduce large bias such that we can not obtain a valid confidence interval. Inspired from a related field called survey sampling, we introduce a variant of the doubly robust (DR) estimator, called the regression-assisted DR estimator, that can incorporate the past data without introducing a large bias. The estimator unifies several existing off-policy policy evaluation methods and improves on them with the use of auxiliary information and a regression approach. We prove that the new estimator is asymptotically unbiased, and provide a consistent variance estimator to a construct a large sample confidence interval. Finally, we empirically show that the new estimator improves estimation for the current and future policy values, and provides a tight and valid interval estimation in several nonstationary recommendation environments.
更多查看译文
关键词
reusing old
AI 理解论文
溯源树
样例
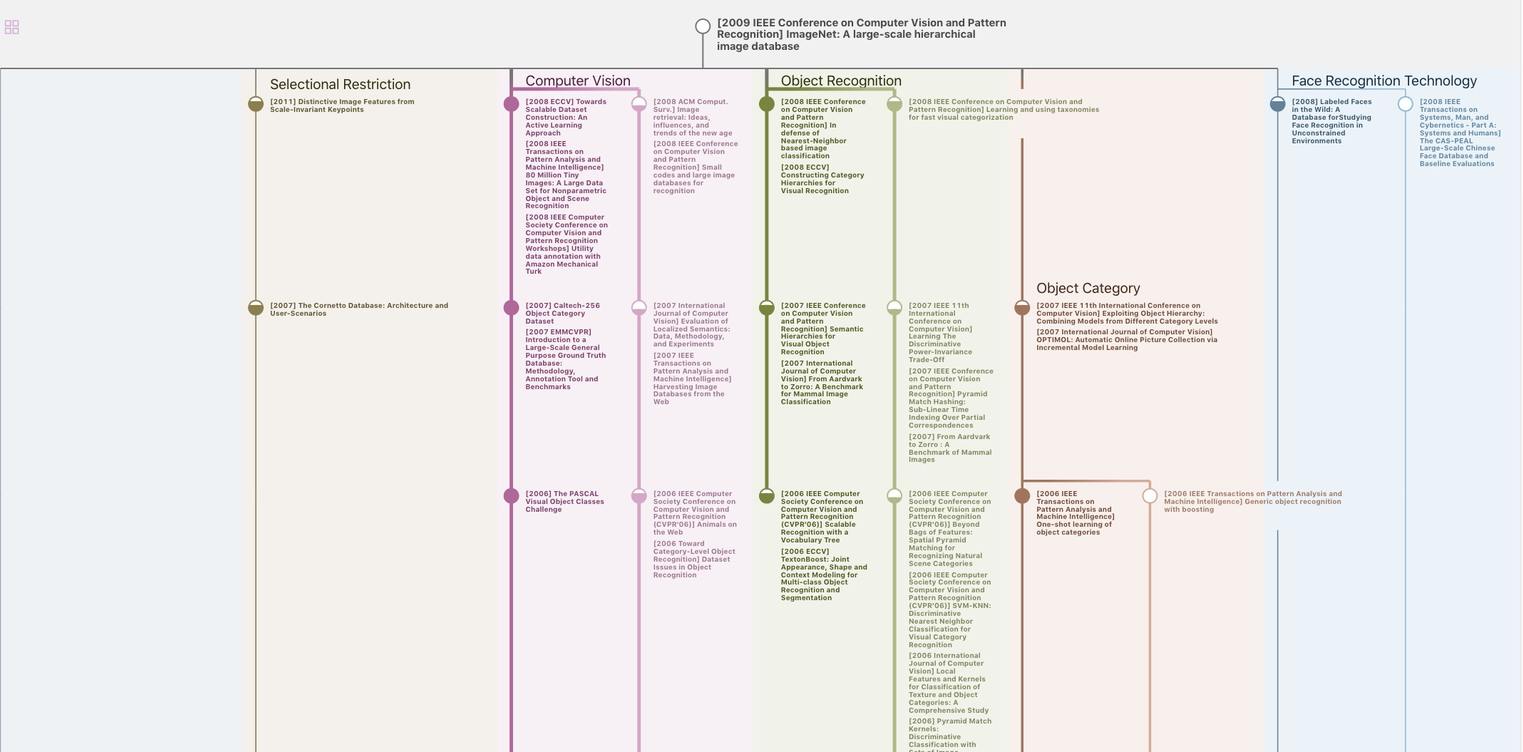
生成溯源树,研究论文发展脉络
Chat Paper
正在生成论文摘要