Learning from Multiple Sources for Data-to-Text and Text-to-Data
arxiv(2023)
摘要
Data-to-text (D2T) and text-to-data (T2D) are dual tasks that convert structured data, such as graphs or tables into fluent text, and vice versa. These tasks are usually handled separately and use corpora extracted from a single source. Current systems leverage pre-trained language models fine-tuned on D2T or T2D tasks. This approach has two main limitations: first, a separate system has to be tuned for each task and source; second, learning is limited by the scarcity of available corpora. This paper considers a more general scenario where data are available from multiple heterogeneous sources. Each source, with its specific data format and semantic domain, provides a non-parallel corpus of text and structured data. We introduce a variational auto-encoder model with disentangled style and content variables that allows us to represent the diversity that stems from multiple sources of text and data. Our model is designed to handle the tasks of D2T and T2D jointly. We evaluate our model on several datasets, and show that by learning from multiple sources, our model closes the performance gap with its supervised single-source counterpart and outperforms it in some cases.
更多查看译文
关键词
multiple sources,learning,data-to-text,text-to-data
AI 理解论文
溯源树
样例
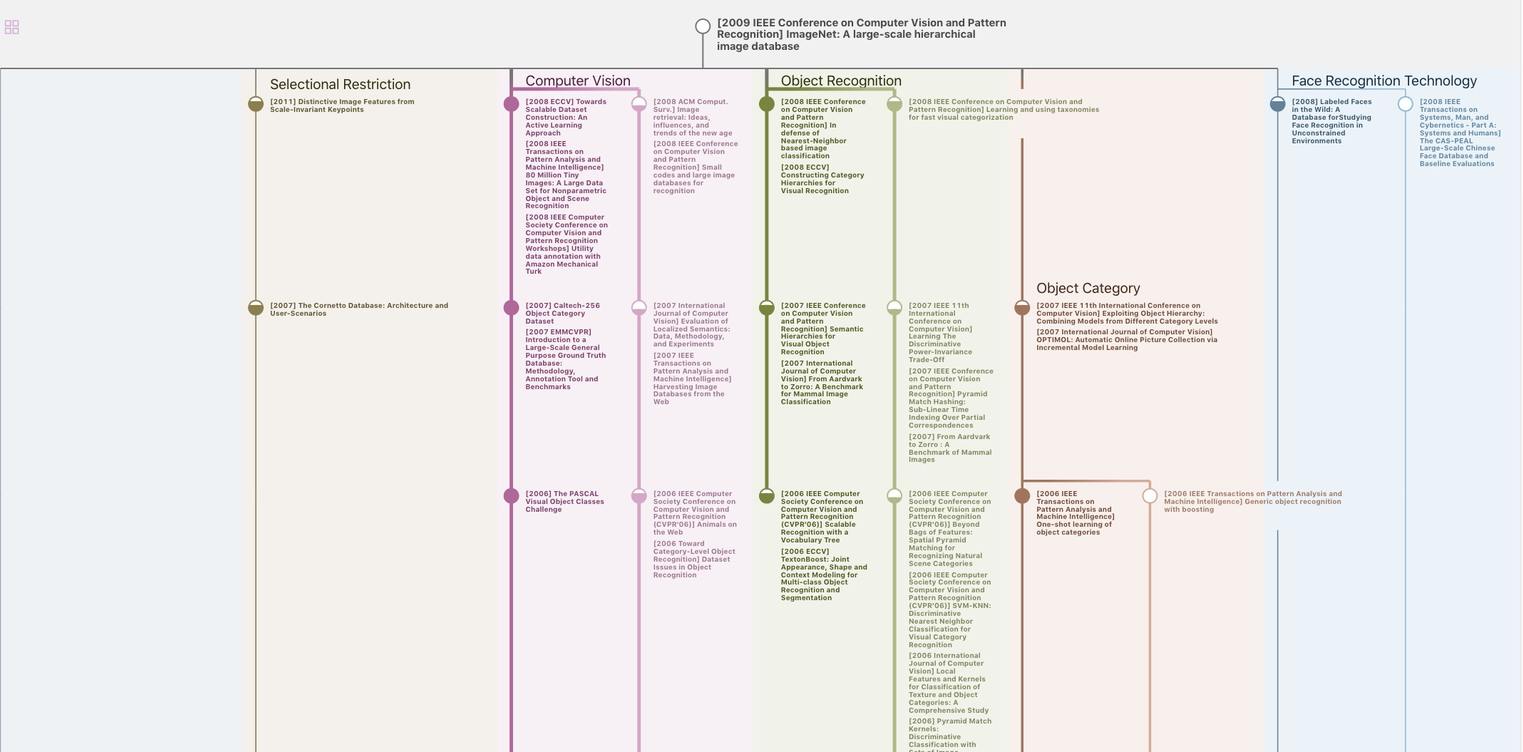
生成溯源树,研究论文发展脉络
Chat Paper
正在生成论文摘要