FederatedTrust: A solution for trustworthy federated learning
FUTURE GENERATION COMPUTER SYSTEMS-THE INTERNATIONAL JOURNAL OF ESCIENCE(2024)
摘要
The rapid expansion of the Internet of Things (IoT) and Edge Computing has presented challenges for centralized Machine and Deep Learning (ML/DL) methods due to the presence of distributed data silos that hold sensitive information. To address concerns regarding data privacy, collaborative and privacy-preserving ML/DL techniques like Federated Learning (FL) have emerged. FL ensures data privacy by design, as the local data of participants remains undisclosed during the creation of a global and collaborative model. However, data privacy and performance are insufficient since a growing need demands trust in model predictions. Existing literature has proposed various approaches dealing with trustworthy ML/DL (excluding data privacy), identifying robustness, fairness, explainability, and accountability as important pillars. Nevertheless, further research is required to identify trustworthiness pillars and evaluation metrics specifically relevant to FL models, as well as to develop solutions that can compute the trustworthiness level of FL models. This work examines the existing requirements for evaluating trustworthiness in FL and introduces a comprehensive taxonomy consisting of six pillars (privacy, robustness, fairness, explainability, accountability, and federation), along with over 30 metrics for computing the trustworthiness of FL models. Subsequently, an algorithm named FederatedTrust is designed based on the pillars and metrics identified in the taxonomy to compute the trustworthiness score of FL models. A prototype of FederatedTrust is implemented and integrated into the learning process of FederatedScope, a well-established FL framework. Finally, five experiments are conducted using different configurations of FederatedScope (with different participants, selection rates, training rounds, and differential privacy) to demonstrate the utility of FederatedTrust in computing the trustworthiness of FL models. Three experiments employ the FEMNIST dataset, and two utilize the N-BaIoT dataset, considering a real-world IoT security use case.
更多查看译文
关键词
Trustworthy federated learning,Trust assessment,AI governance,Privacy,Robustness,Fairness,Explainability,Accountability
AI 理解论文
溯源树
样例
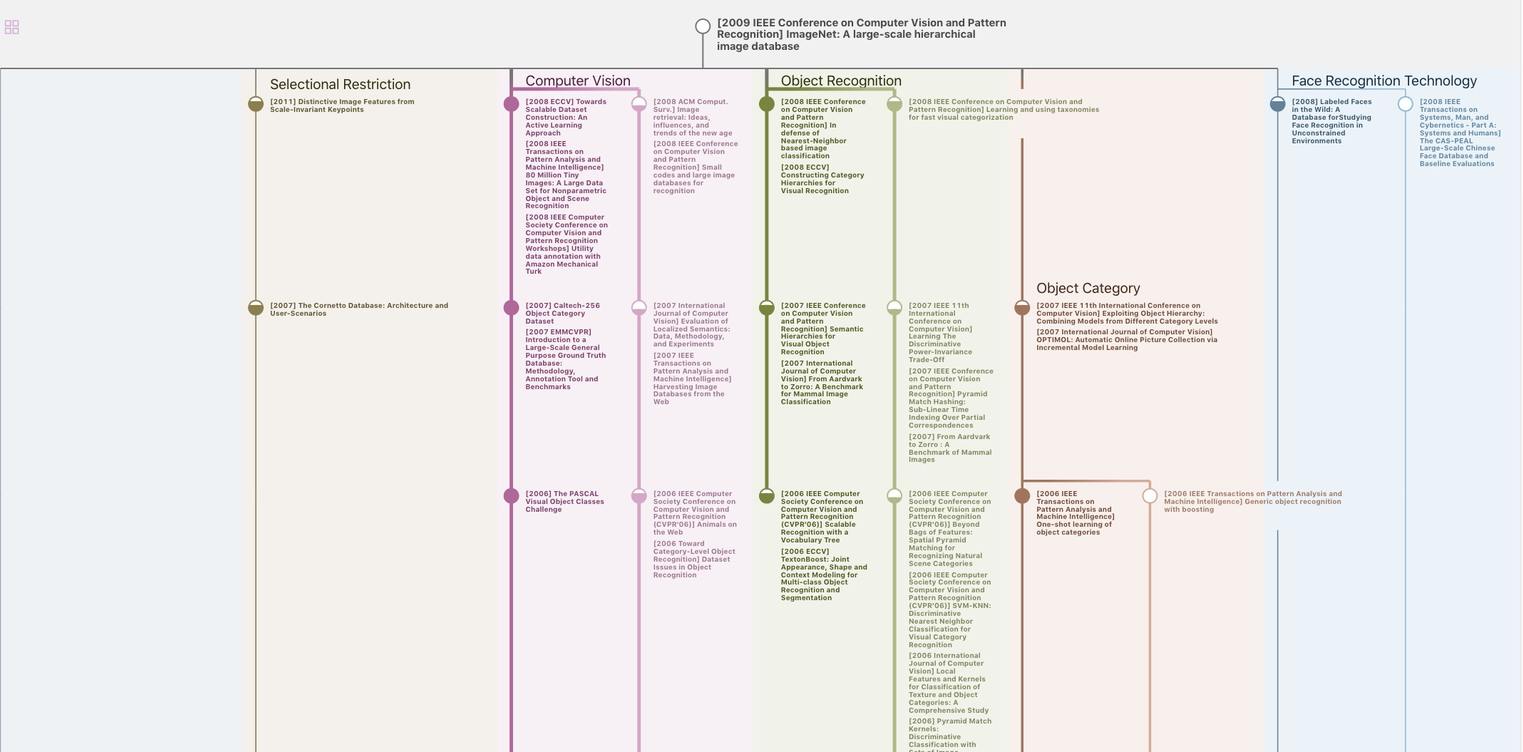
生成溯源树,研究论文发展脉络
Chat Paper
正在生成论文摘要