ViTA: A Vision Transformer Inference Accelerator for Edge Applications
arxiv(2023)
摘要
Vision Transformer models, such as ViT, Swin Transformer, and Transformer-in-Transformer, have recently gained significant traction in computer vision tasks due to their ability to capture the global relation between features which leads to superior performance. However, they are compute-heavy and difficult to deploy in resource-constrained edge devices. Existing hardware accelerators, including those for the closely-related BERT transformer models, do not target highly resource-constrained environments. In this paper, we address this gap and propose ViTA - a configurable hardware accelerator for inference of vision transformer models, targeting resource-constrained edge computing devices and avoiding repeated off-chip memory accesses. We employ a head-level pipeline and inter-layer MLP optimizations, and can support several commonly used vision transformer models with changes solely in our control logic. We achieve nearly 90% hardware utilization efficiency on most vision transformer models, report a power of 0.88W when synthesised with a clock of 150 MHz, and get reasonable frame rates - all of which makes ViTA suitable for edge applications.
更多查看译文
关键词
Vision Transformer, Swin Transformer, Hardware, Accelerator, Computer Vision, Edge Computing, FPGA
AI 理解论文
溯源树
样例
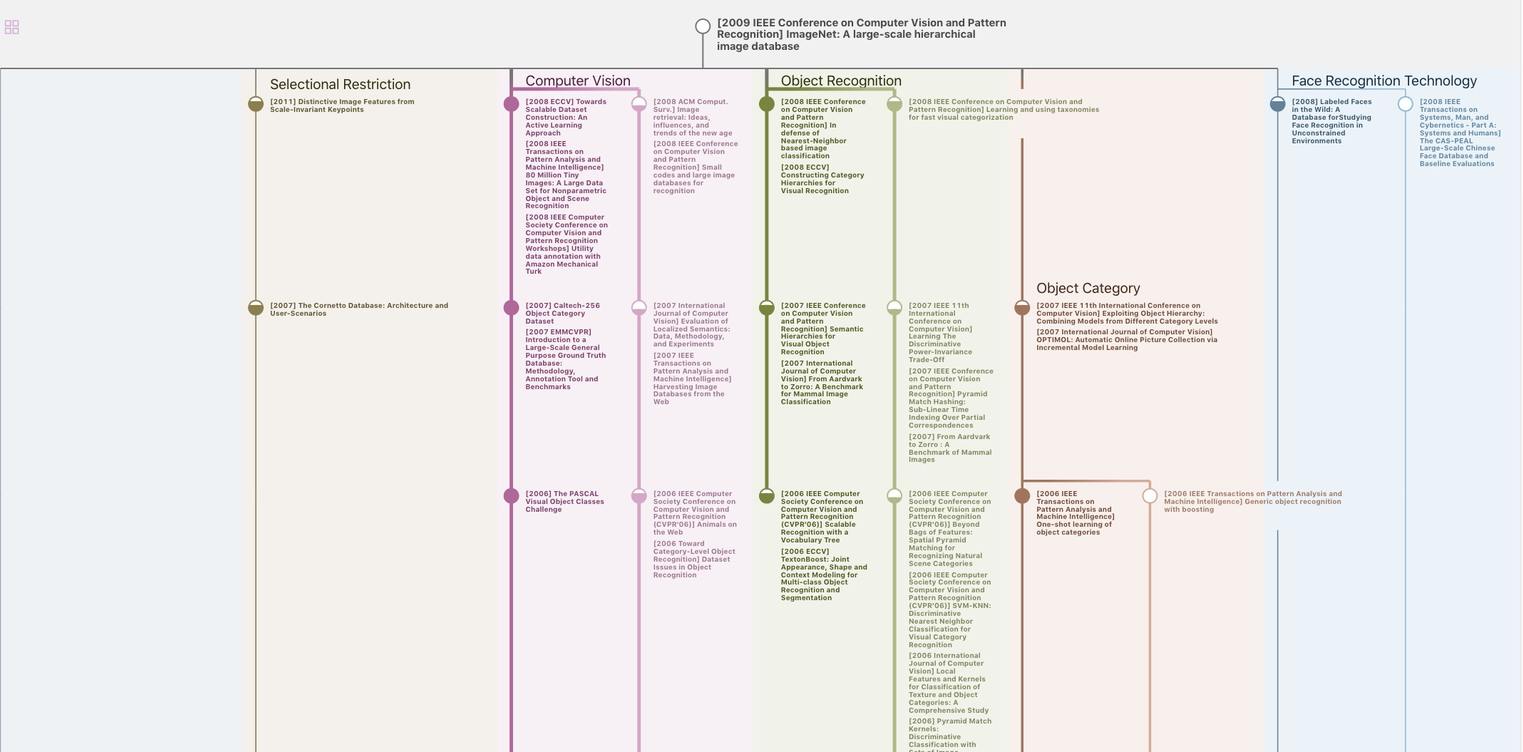
生成溯源树,研究论文发展脉络
Chat Paper
正在生成论文摘要