PolyFormer: Referring Image Segmentation As Sequential Polygon Generation
CVPR 2023(2023)
摘要
In this work, instead of directly predicting the pixel-level segmentation masks, the problem of referring image segmentation is formulated as sequential polygon generation, and the predicted polygons can be later converted into segmentation masks. This is enabled by a new sequence-to-sequence framework, Polygon Transformer (PolyFormer), which takes a sequence of image patches and text query tokens as input, and outputs a sequence of polygon vertices autoregressively. For more accurate geometric localization, we propose a regression-based decoder, which predicts the precise floating-point coordinates directly, without any coordinate quantization error. In the experiments, PolyFormer outperforms the prior art by a clear margin, e.g., 5.40% and 4.52% absolute improvements on the challenging RefCOCO+ and RefCOCOg datasets. It also shows strong generalization ability when evaluated on the referring video segmentation task without fine-tuning, e.g., achieving competitive 61.5% J&F on the Ref-DAVIS17 dataset.
更多查看译文
关键词
image segmentation,sequential polygon
AI 理解论文
溯源树
样例
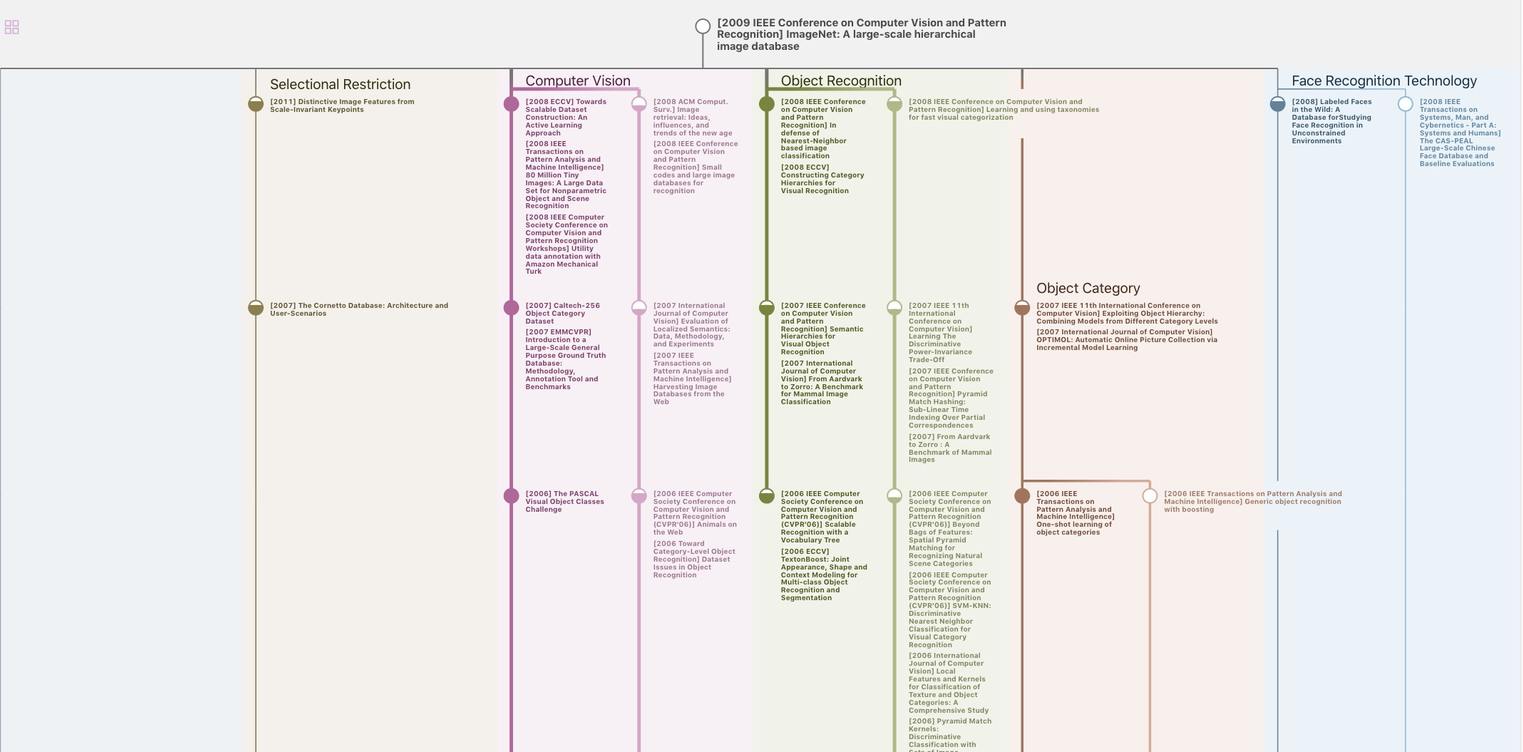
生成溯源树,研究论文发展脉络
Chat Paper
正在生成论文摘要