RePReL: a unified framework for integrating relational planning and reinforcement learning for effective abstraction in discrete and continuous domains
NEURAL COMPUTING & APPLICATIONS(2022)
摘要
We propose a hybrid planner-(deep)reinforcement learning (RL) architecture, RePReL, that leverages a relational planner to efficiently provide useful state abstractions. State abstractions have a tremendous advantage for better generalization and transfer in RL. Our framework takes an important step toward constructing these abstractions. Specifically, the framework enables multi-level abstractions by leveraging a high-level planner to communicate with a low-level (deep) reinforcement learner. Our empirical results demonstrate the generalization and transfer capabilities of the framework in both discrete and continuous domains with rich structures (objects and relations between these objects). A key aspect of RePReL is that it can be seen as a plug-and-play framework where different planners can be used in combination with different (deep) RL agents.
更多查看译文
关键词
Planning,Reinforcement learning,Abstractions
AI 理解论文
溯源树
样例
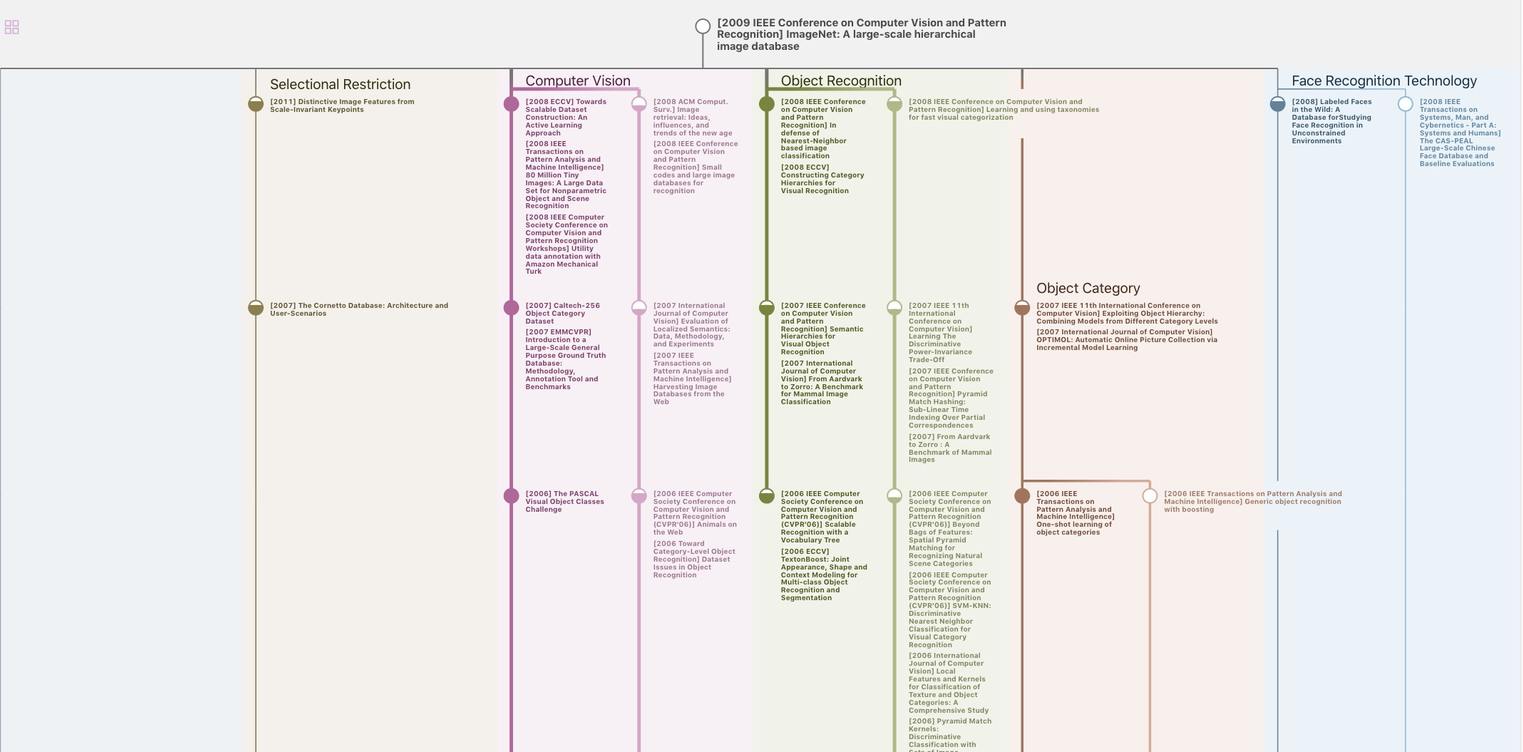
生成溯源树,研究论文发展脉络
Chat Paper
正在生成论文摘要