Enhancing User Personalization in Conversational Recommenders
WWW 2023(2023)
摘要
Conversational recommenders are emerging as a powerful tool to personalize a user's recommendation experience. Through a back-and-forth dialogue, users can quickly hone in on just the right items. Many approaches to conversational recommendation, however, only partially explore the user preference space and make limiting assumptions about how user feedback can be best incorporated, resulting in long dialogues and poor recommendation performance. In this paper, we propose a novel conversational recommendation framework with two unique features: (i) a greedy NDCG attribute selector, to enhance user personalization in the interactive preference elicitation process by prioritizing attributes that most effectively represent the actual preference space of the user; and (ii) a user representation refiner, to effectively fuse together the user preferences collected from the interactive elicitation process to obtain a more personalized understanding of the user. Through extensive experiments on four frequently used datasets, we find the proposed framework not only outperforms all the state-of-the-art conversational recommenders (in terms of both recommendation performance and conversation efficiency), but also provides a more personalized experience for the user under the proposed multi-groundtruth multi-round conversational recommendation setting.
更多查看译文
关键词
conversational recommenders,user personalization
AI 理解论文
溯源树
样例
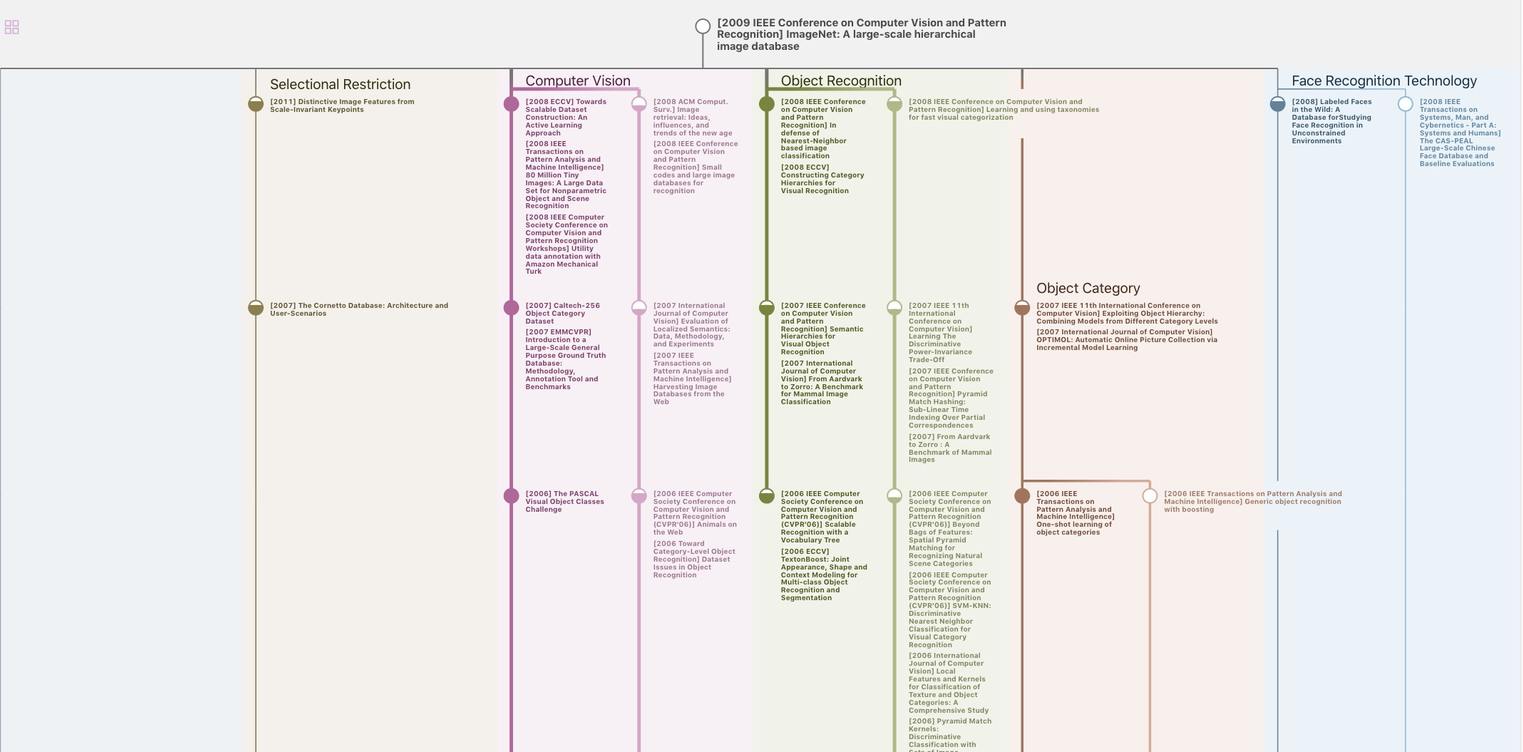
生成溯源树,研究论文发展脉络
Chat Paper
正在生成论文摘要