High Probability Convergence of Clipped-SGD Under Heavy-tailed Noise
arxiv(2023)
摘要
While the convergence behaviors of stochastic gradient methods are well understood \emph{in expectation}, there still exist many gaps in the understanding of their convergence with \emph{high probability}, where the convergence rate has a logarithmic dependency on the desired success probability parameter. In the \emph{heavy-tailed noise} setting, where the stochastic gradient noise only has bounded $p$-th moments for some $p\in(1,2]$, existing works could only show bounds \emph{in expectation} for a variant of stochastic gradient descent (SGD) with clipped gradients, or high probability bounds in special cases (such as $p=2$) or with extra assumptions (such as the stochastic gradients having bounded non-central moments). In this work, using a novel analysis framework, we present new and time-optimal (up to logarithmic factors) \emph{high probability} convergence bounds for SGD with clipping under heavy-tailed noise for both convex and non-convex smooth objectives using only minimal assumptions.
更多查看译文
关键词
high probability convergence,noise,clipped-sgd,heavy-tailed
AI 理解论文
溯源树
样例
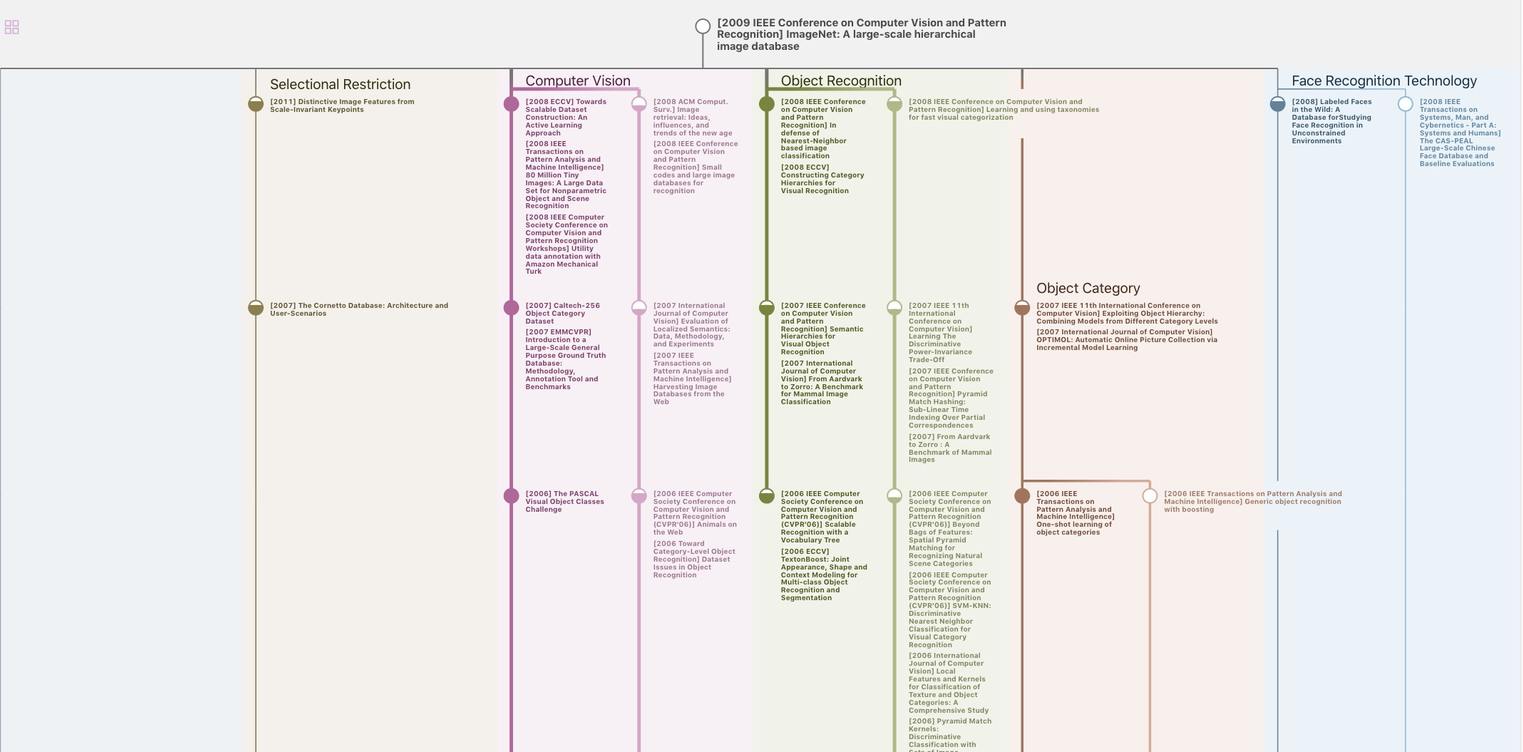
生成溯源树,研究论文发展脉络
Chat Paper
正在生成论文摘要