Sterling: Synergistic Representation Learning on Bipartite Graphs
AAAI 2024(2024)
摘要
A fundamental challenge of bipartite graph representation learning is how to extract informative node embeddings. Self-Supervised Learning (SSL) is a promising paradigm to address this challenge. Most recent bipartite graph SSL methods are based on contrastive learning which learns embeddings by discriminating positive and negative node pairs. Contrastive learning usually requires a large number of negative node pairs, which could lead to computational burden and semantic errors. In this paper, we introduce a novel synergistic representation learning model (STERLING) to learn node embeddings without negative node pairs. STERLING preserves the unique local and global synergies in bipartite graphs. The local synergies are captured by maximizing the similarity of the inter-type and intra-type positive node pairs, and the global synergies are captured by maximizing the mutual information of co-clusters. Theoretical analysis demonstrates that STERLING could improve the connectivity between different node types in the embedding space. Extensive empirical evaluation on various benchmark datasets and tasks demonstrates the effectiveness of STERLING for extracting node embeddings.
更多查看译文
关键词
ML: Graph-based Machine Learning,DMKM: Graph Mining, Social Network Analysis & Community
AI 理解论文
溯源树
样例
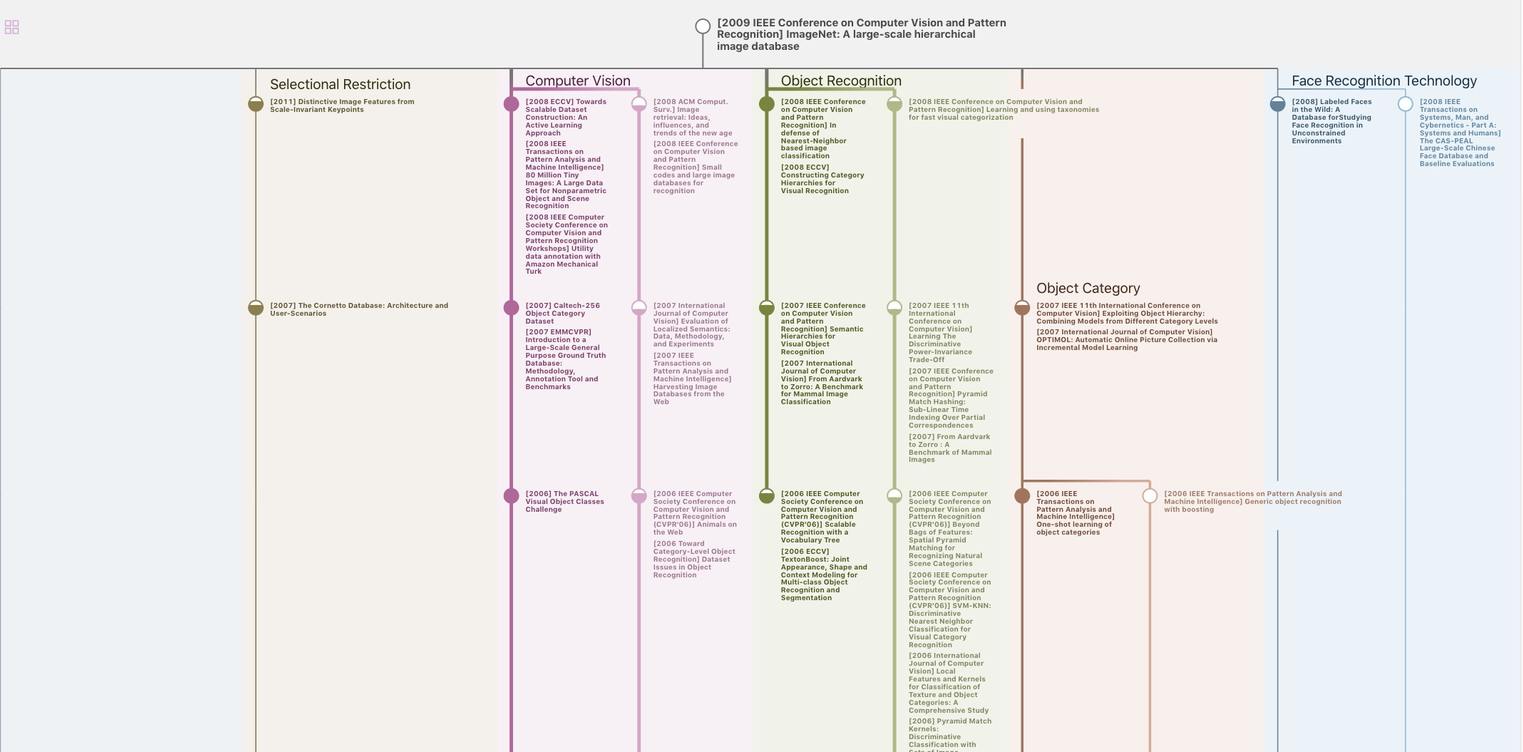
生成溯源树,研究论文发展脉络
Chat Paper
正在生成论文摘要