Gaussian Process-Gated Hierarchical Mixtures of Experts.
IEEE transactions on pattern analysis and machine intelligence(2024)
摘要
In this paper, we propose novel Gaussian process-gated hierarchical mixtures of experts (GPHMEs). Unlike other mixtures of experts with gating models linear in the input, our model employs gating functions built with Gaussian processes (GPs). These processes are based on random features that are non-linear functions of the inputs. Furthermore, the experts in our model are also constructed with GPs. The optimization of the GPHMEs is performed by variational inference. The proposed GPHMEs have several advantages. They outperform tree-based HME benchmarks that partition the data in the input space, and they achieve good performance with reduced complexity. Another advantage is the interpretability they provide for deep GPs, and more generally, for deep Bayesian neural networks. Our GPHMEs demonstrate excellent performance for large-scale data sets, even with quite modest sizes.
更多查看译文
关键词
And random features,gaussian processes,mixtures of experts,soft decision trees
AI 理解论文
溯源树
样例
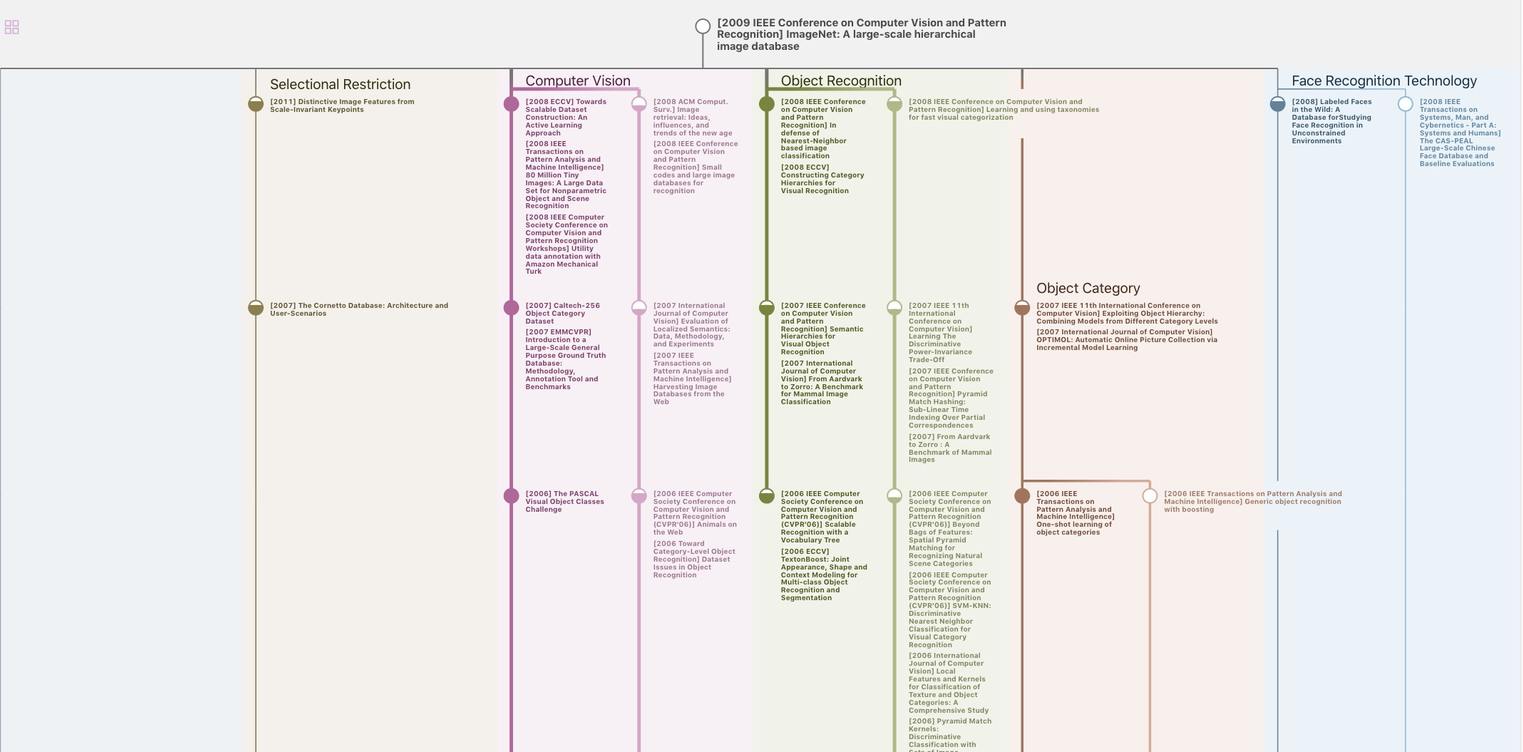
生成溯源树,研究论文发展脉络
Chat Paper
正在生成论文摘要