Deep Reinforcement Learning for Online Error Detection in Cyber-Physical Systems
arxiv(2023)
摘要
Reliability is one of the major design criteria in Cyber-Physical Systems (CPSs). This is because of the existence of some critical applications in CPSs and their failure is catastrophic. Therefore, employing strong error detection and correction mechanisms in CPSs is inevitable. CPSs are composed of a variety of units, including sensors, networks, and microcontrollers. Each of these units is probable to be in a faulty state at any time and the occurred fault can result in erroneous output. The fault may cause the units of CPS to malfunction and eventually crash. Traditional fault-tolerant approaches include redundancy time, hardware, information, and/or software. However, these approaches impose significant overheads besides their low error coverage, which limits their applicability. In addition, the interval between error occurrence and detection is too long in these approaches. In this paper, based on Deep Reinforcement Learning (DRL), a new error detection approach is proposed that not only detects errors with high accuracy but also can perform error detection at the moment due to very low inference time. The proposed approach can categorize different types of errors from normal data and predict whether the system will fail. The evaluation results illustrate that the proposed approach has improved more than 2x in terms of accuracy and more than 5x in terms of inference time compared to other approaches.
更多查看译文
关键词
online error detection,reinforcement learning,deep reinforcement,cyber-physical
AI 理解论文
溯源树
样例
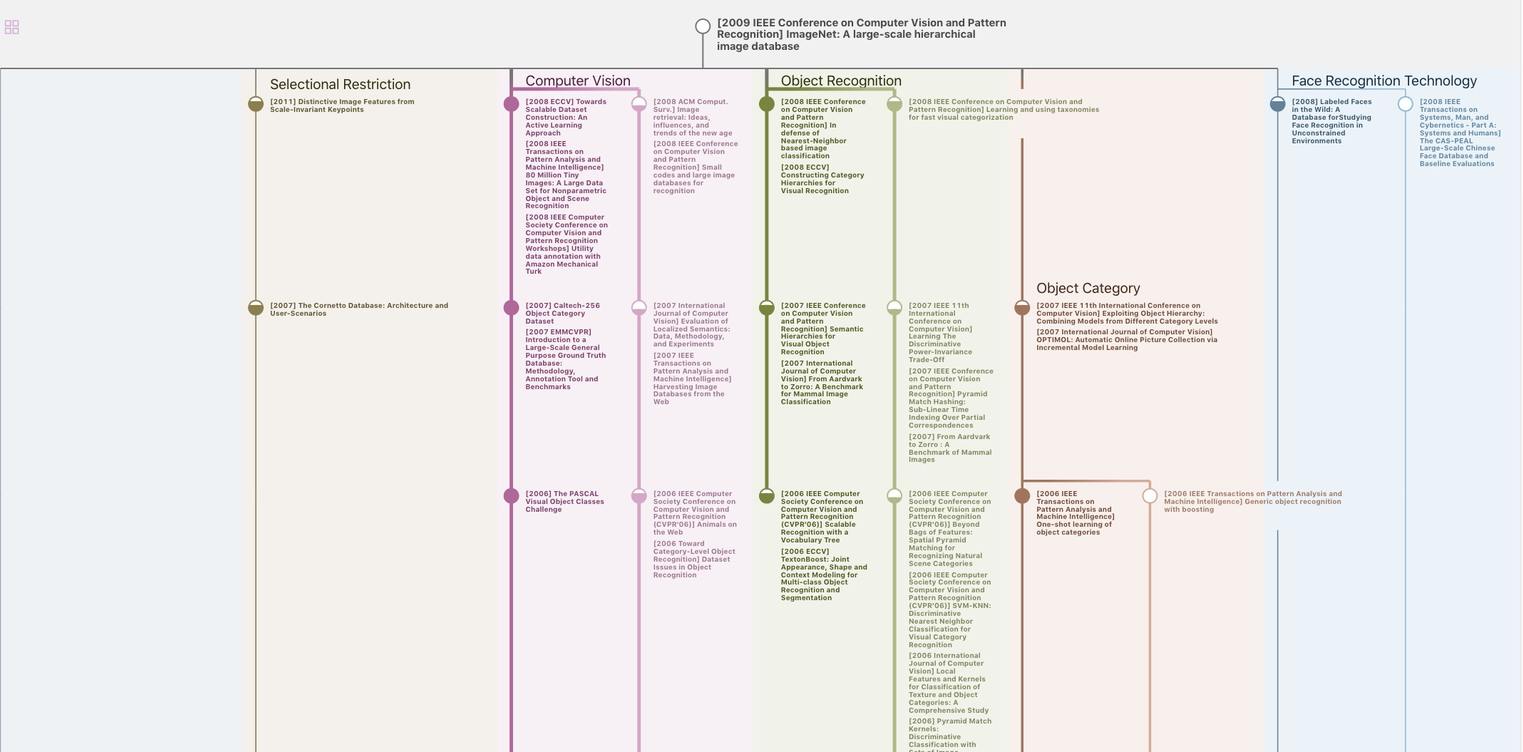
生成溯源树,研究论文发展脉络
Chat Paper
正在生成论文摘要