Where to Diffuse, How to Diffuse and How to get back: Learning in Multivariate Diffusions
ICLR 2023(2023)
摘要
Diffusion-based generative models (DBGMs) perturb data to a target noise distribution and reverse this inference process to generate samples. The choice of inference diffusion affects both likelihoods and sample quality as it is tied to the generative model. Recent work in DBGMs has applied the principle of improving generative models with the use of auxiliary variables, leading to improved sample quality. While there are many such multivariate diffusions to explore, each new one requires significant model-specific analysis, hindering rapid prototyping and evaluation. In this work, we study linear Multivariate Diffusion Models (MDMs). First, for any number of auxiliary variables, we provide a recipe for maximizing a lower-bound on the MDM likelihood, without requiring any model-specific analysis. Next, we demonstrate how to parameterize the diffusion for a specified target noise distribution; these two points together enable optimizing the inference diffusion process. Optimizing the diffusion expands easy experimentation from just a few well-known processes to an automatic search over the set of linear diffusions. To demonstrate these ideas, we introduce two new specific diffusions as well as learn a diffusion process on the MNIST and CIFAR10 datasets. We achieve improved bits-per-dim bounds using the new diffusion, compared to the existing likelihood-trained VPSDE. We additionally connect the existing CLD objective to the likelihood lower-bound.
更多查看译文
关键词
Diffusion models,score based generative model,generative models,variational inference
AI 理解论文
溯源树
样例
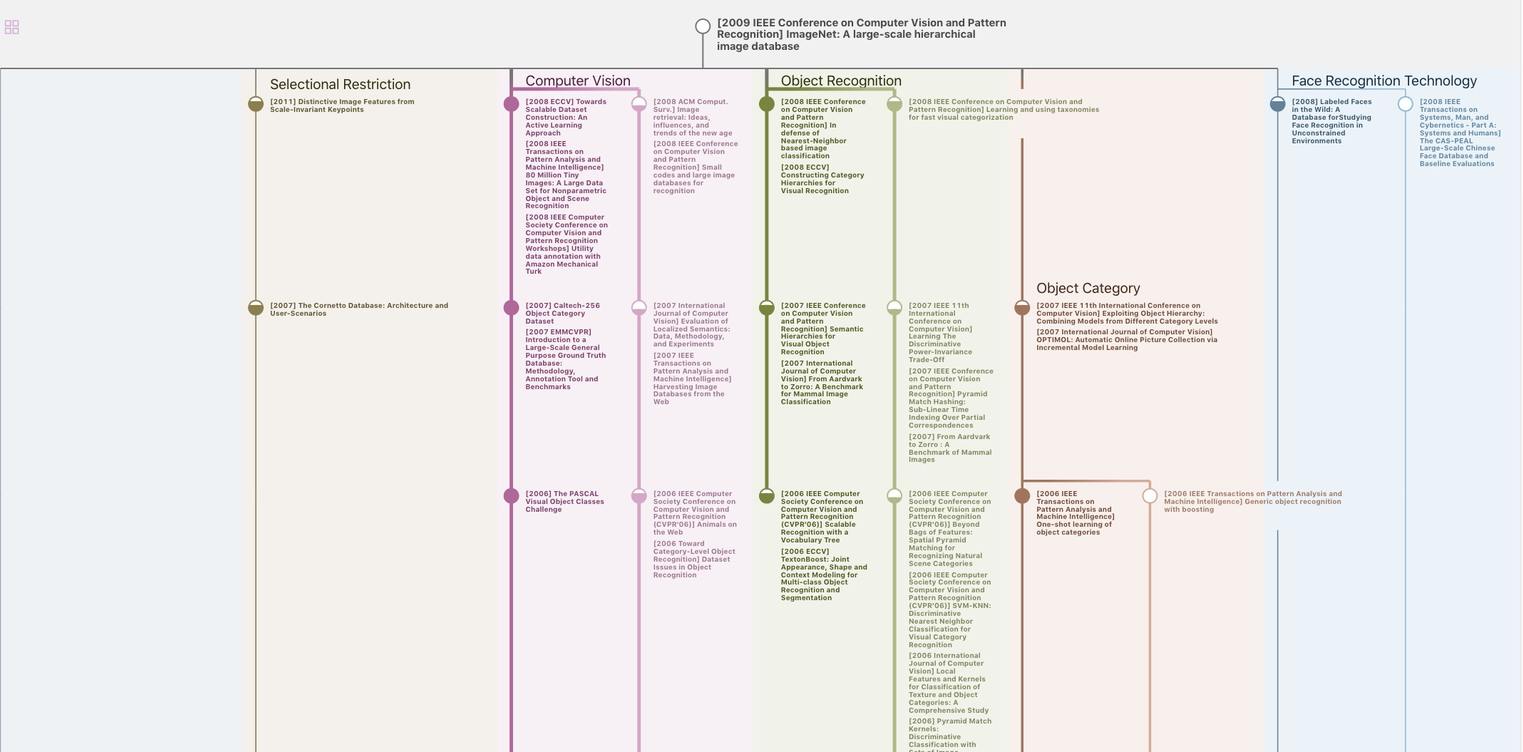
生成溯源树,研究论文发展脉络
Chat Paper
正在生成论文摘要