Warping the Space: Weight Space Rotation for Class-Incremental Few-Shot Learning
ICLR 2023(2023)
摘要
Class-incremental few-shot learning, where new sets of classes are provided sequentially with only a few training samples, presents a great challenge due to catastrophic forgetting of old knowledge and overfitting caused by lack of data. During finetuning on new classes, the performance on previous classes deteriorates quickly even when only a small fraction of parameters are updated, since the previous knowledge is broadly associated with most of the model parameters in the original parameter space. In this paper, we introduce WaRP, the \textit{weight space rotation process}, which transforms the original parameter space into a new space so that we can push most of the previous knowledge compactly into only a few important parameters. By properly identifying and freezing these key parameters in the new weight space, we can finetune the remaining parameters without affecting the knowledge of previous classes. As a result, WaRP provides an additional room for the model to effectively learn new classes in future incremental sessions. Experimental results confirm the effectiveness of our solution and show the improved performance over the state-of-the-art methods.
更多查看译文
关键词
incremental few-shot learning,catastrophic forgetting,parameter space,weight space rotation
AI 理解论文
溯源树
样例
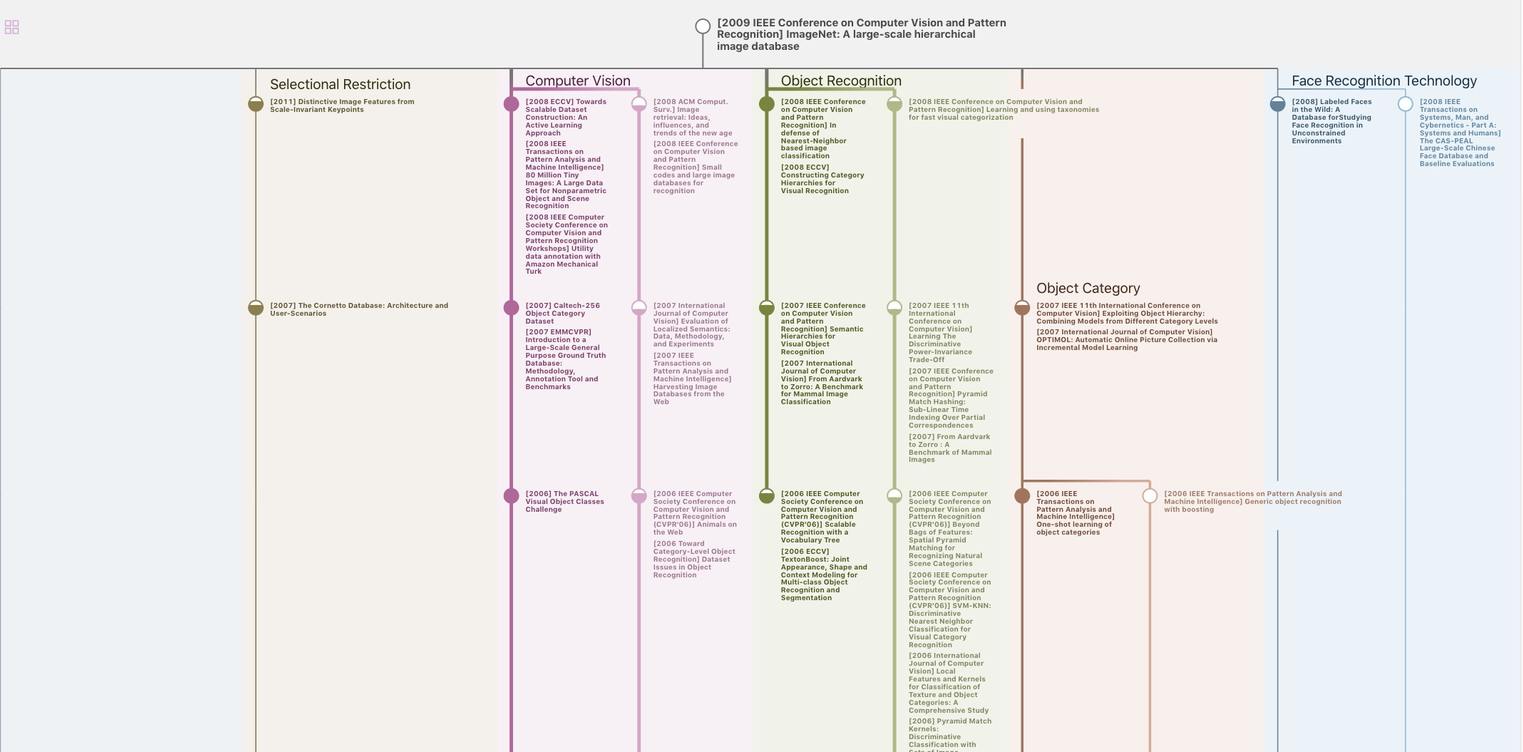
生成溯源树,研究论文发展脉络
Chat Paper
正在生成论文摘要