Over-parameterised Shallow Neural Networks with Asymmetrical Node Scaling: Global Convergence Guarantees and Feature Learning
arxiv(2023)
摘要
We consider the optimisation of large and shallow neural networks via gradient flow, where the output of each hidden node is scaled by some positive parameter. We focus on the case where the node scalings are non-identical, differing from the classical Neural Tangent Kernel (NTK) parameterisation. We prove that, for large neural networks, with high probability, gradient flow converges to a global minimum AND can learn features, unlike in the NTK regime. We also provide experiments on synthetic and real-world datasets illustrating our theoretical results and showing the benefit of such scaling in terms of pruning and transfer learning.
更多查看译文
关键词
shallow neural networks,neural networks,scaling,feature learning,over-parameterised
AI 理解论文
溯源树
样例
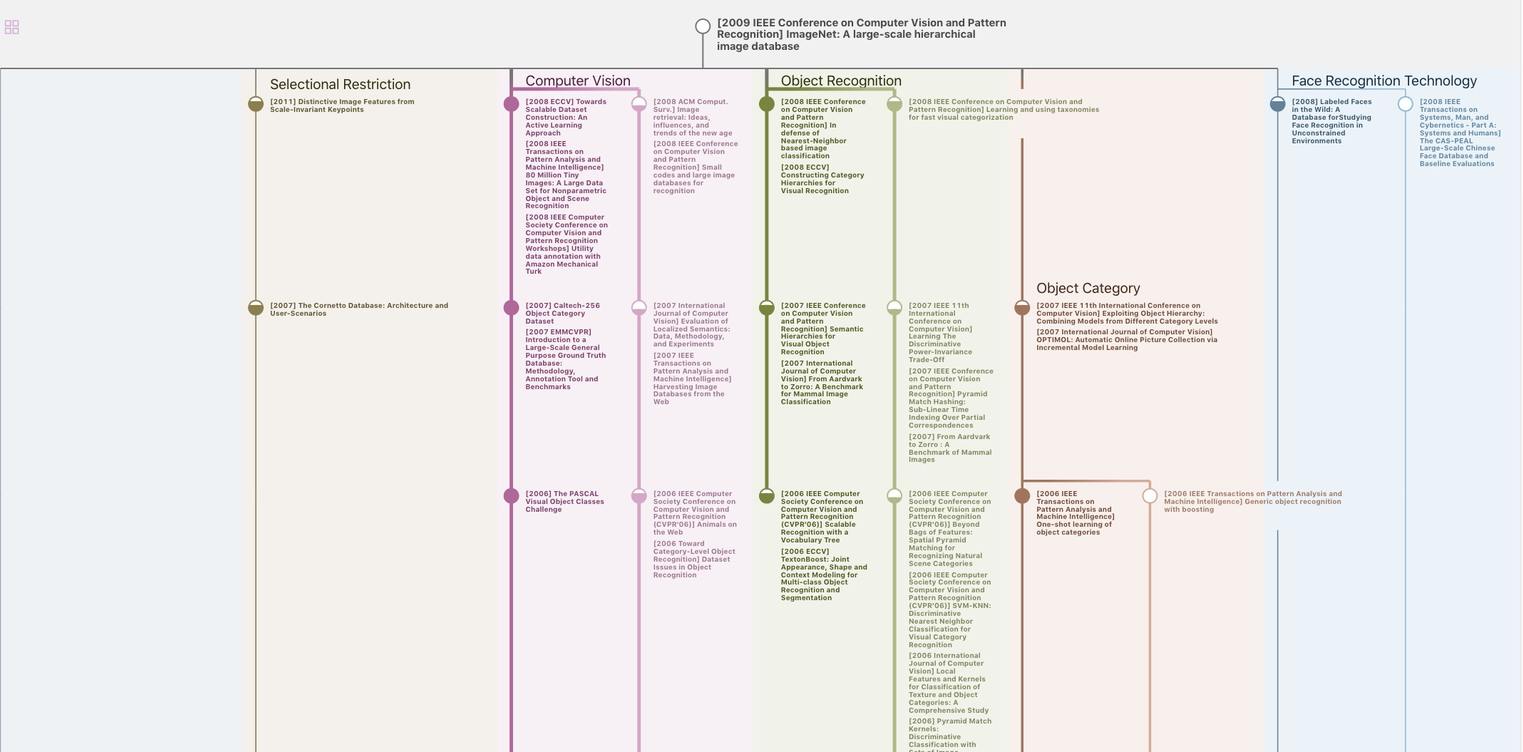
生成溯源树,研究论文发展脉络
Chat Paper
正在生成论文摘要