Chance-Constrained Trajectory Optimization for High-DOF Robots in Uncertain Environments
arxiv(2023)
摘要
Many practical applications of robotics require systems that can operate safely despite uncertainty. In the context of motion planning, two types of uncertainty are particularly important when planning safe robot trajectories. The first is environmental uncertainty -- uncertainty in the locations of nearby obstacles, stemming from sensor noise or (in the case of obstacles' future locations) prediction error. The second class of uncertainty is uncertainty in the robots own state, typically caused by tracking or estimation error. To achieve high levels of safety, it is necessary for robots to consider both of these sources of uncertainty. In this paper, we propose a risk-bounded trajectory optimization algorithm, known as Sequential Convex Optimization with Risk Optimization (SCORA), to solve chance-constrained motion planning problems despite both environmental uncertainty and tracking error. Through experiments in simulation, we demonstrate that SCORA significantly outperforms state-of-the-art risk-aware motion planners both in planning time and in the safety of the resulting trajectories.
更多查看译文
关键词
uncertain environments,optimization,robots,chance-constrained,high-dof
AI 理解论文
溯源树
样例
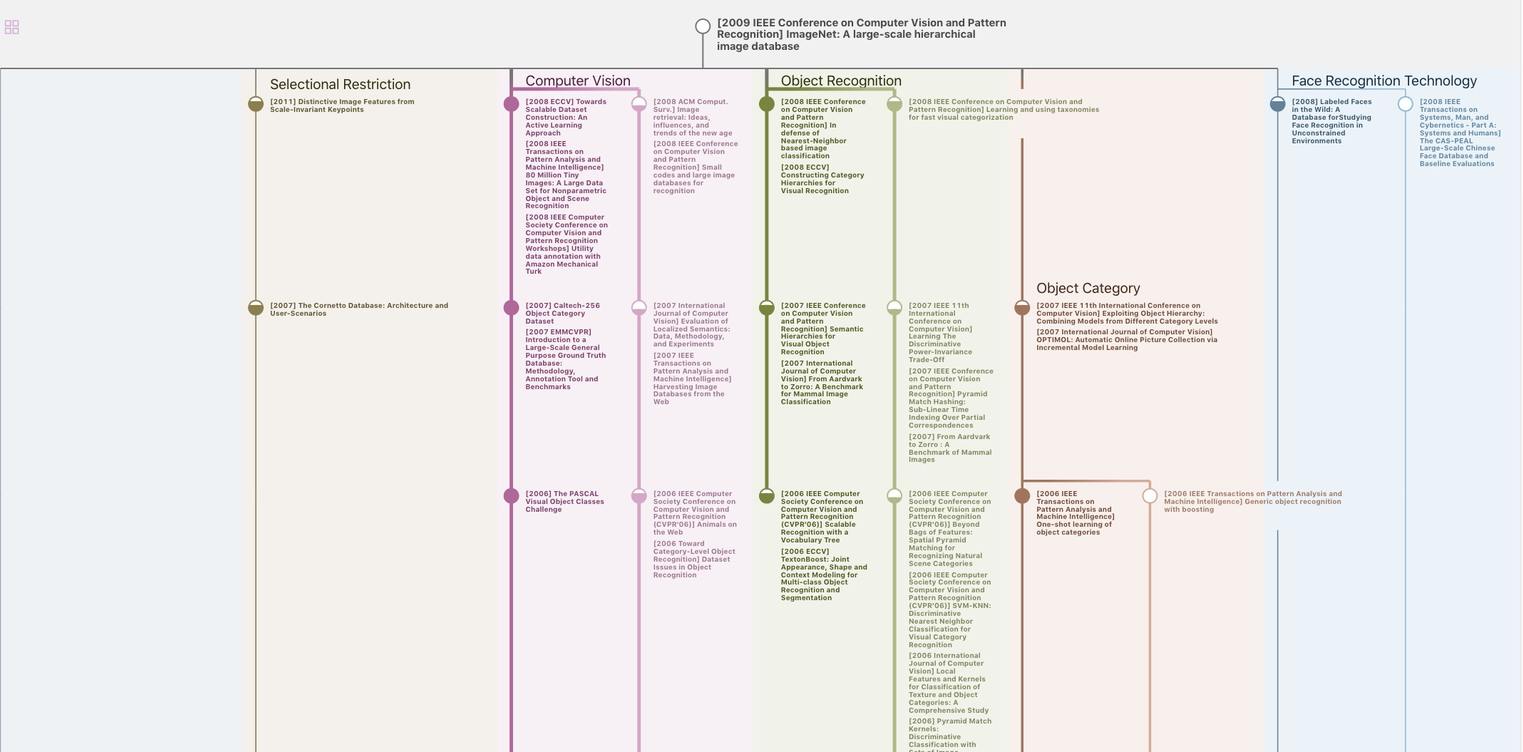
生成溯源树,研究论文发展脉络
Chat Paper
正在生成论文摘要