Virtual Tasks but Real Gains: Improving Multi-Task Learning.
ICPR(2022)
摘要
In supervised multi-task learning, the choice of auxiliary tasks is usually decided manually. This immediately raises the issue of how to choose suitable tasks, which in turn requires the need to measure the similarity between tasks. In this paper, we propose a task-similarity metric that depends solely on the task labels, not on the machine learning models. We show that auxiliary tasks may be synthetically generated from the main tasks with any desired similarity. Labels for these virtual tasks are also generated. Finally, we show that learning these virtual tasks along with the main task leads to real performance gains.
更多查看译文
关键词
virtual tasks,learning,real gains,multi-task
AI 理解论文
溯源树
样例
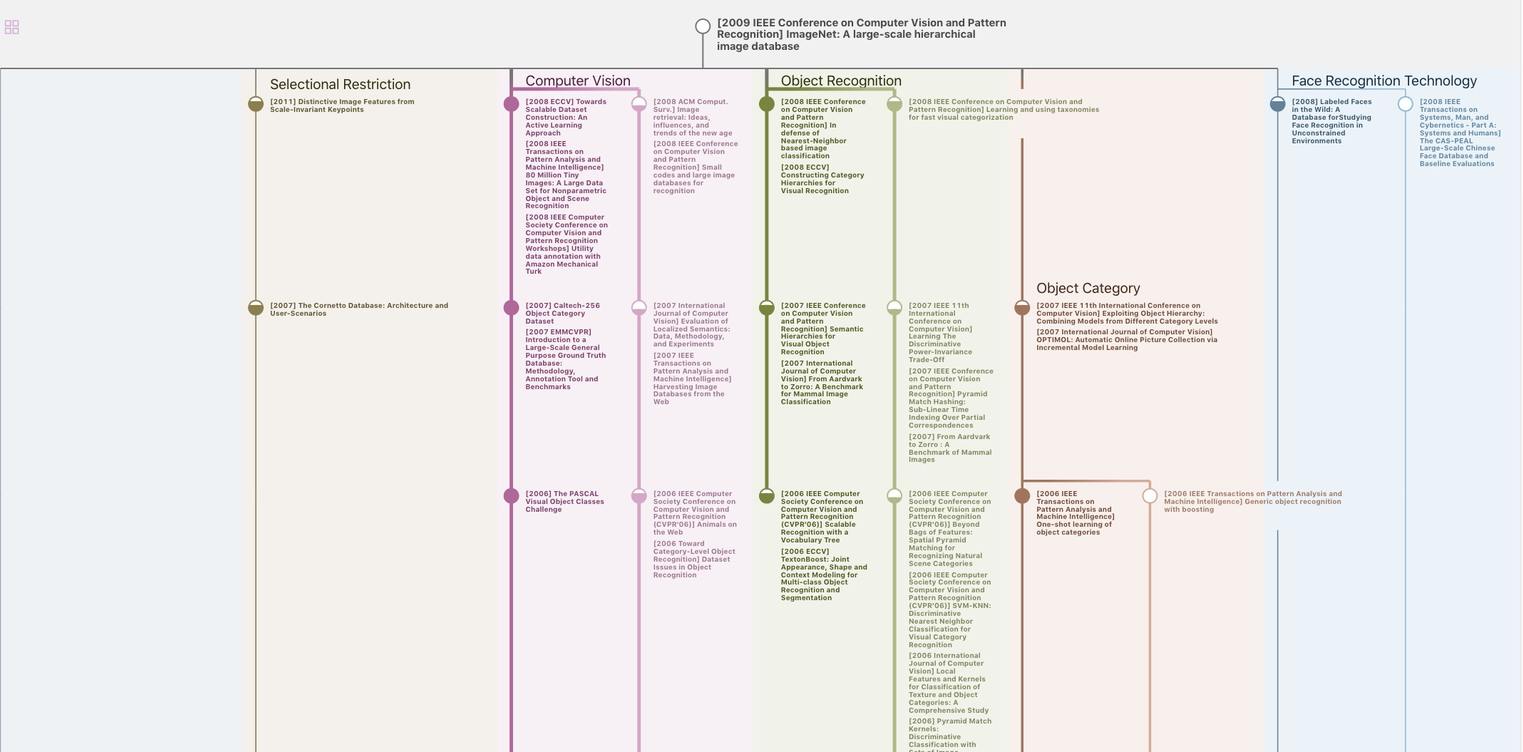
生成溯源树,研究论文发展脉络
Chat Paper
正在生成论文摘要