Stochastic and Dual Adversarial GAN-Boosted Zero-Shot Knowledge Graph.
CICAI (3)(2022)
摘要
Zero-shot knowledge graph (KG) has gained much research attention in recent years. Due to its excellent performance in approximating data distribution, generative adversarial network (GAN) has been used in zero-shot learning for KG completion. However, existing works on GAN-based zero-shot KG completion all use traditional simple architecture without randomness in generator, which greatly limits the ability of GAN mining knowledge on complex datasets. Moreover, the discriminator not only needs to distinguish true data from generated data but also needs to classify generated data correctly at the same time, which affects the optimization process of the generator. In this work, we propose a novel zero-shot KG framework based on stochastic and dual adversarial GAN (SDA) to better mine the association between semantic information and extracted features. Specifically, we introduce a stochastic generator and an additional classifier to improve the model's ability of approximating features and classifying unseen tasks. The experiments on NELL-ZS and Wiki-ZS datasets show that the proposed SDA outperforms the classic methods in zero-shot KG completion task. In particular, the proposed SDA receives a 0.6% and 0.7% increase on mean reciprocal ranking (MRR) for NELL-ZS and Wiki-ZS datasets, respectively.
更多查看译文
关键词
knowledge,gan-boosted,zero-shot
AI 理解论文
溯源树
样例
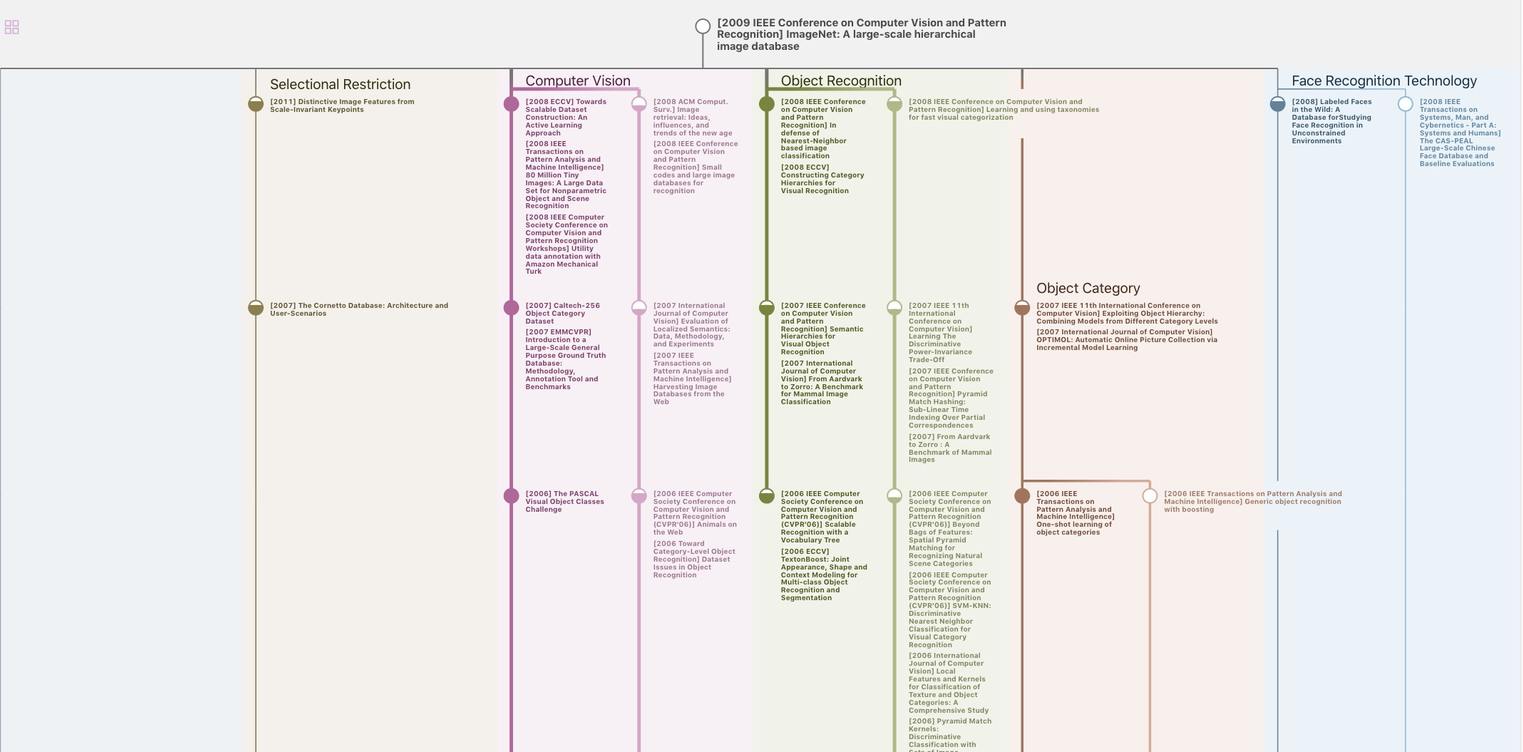
生成溯源树,研究论文发展脉络
Chat Paper
正在生成论文摘要