Sequential Short-Text Classification from Multiple Textual Representations with Weak Supervision.
BRACIS (1)(2022)
摘要
The amount of news generated on the internet has increased significantly in recent years. As a trend, text data has gained attention from industry, government, academia, and the financial market. This information is potentially valuable to assist domain experts in decision making. Therefore, related applications based on machine learning have been widely available in several areas of knowledge. However, for supervised learning tasks, the availability of annotated texts in quantity and quality is a recurring problem. This work proposes a time-series-driven approach to labeling chronologically arranged documents. Our proposal categorizes short texts for a particular domain according to the level and trend patterns of a given time series. We use the obtained weak labels with the understanding that they are imperfect but still useful for building predictive text models. Documents and agribusiness commodity price series were employed to assess performance in four classification scenarios. The experimental evaluation considered nine textual representations and different learning paradigms. Neural language-based models demonstrated better classification performance than traditional ones. The results indicate that the proposed approach can be an alternative for automatically labeling a large news volume.
更多查看译文
关键词
Data labeling, Machine learning, Text mining, Weak supervision
AI 理解论文
溯源树
样例
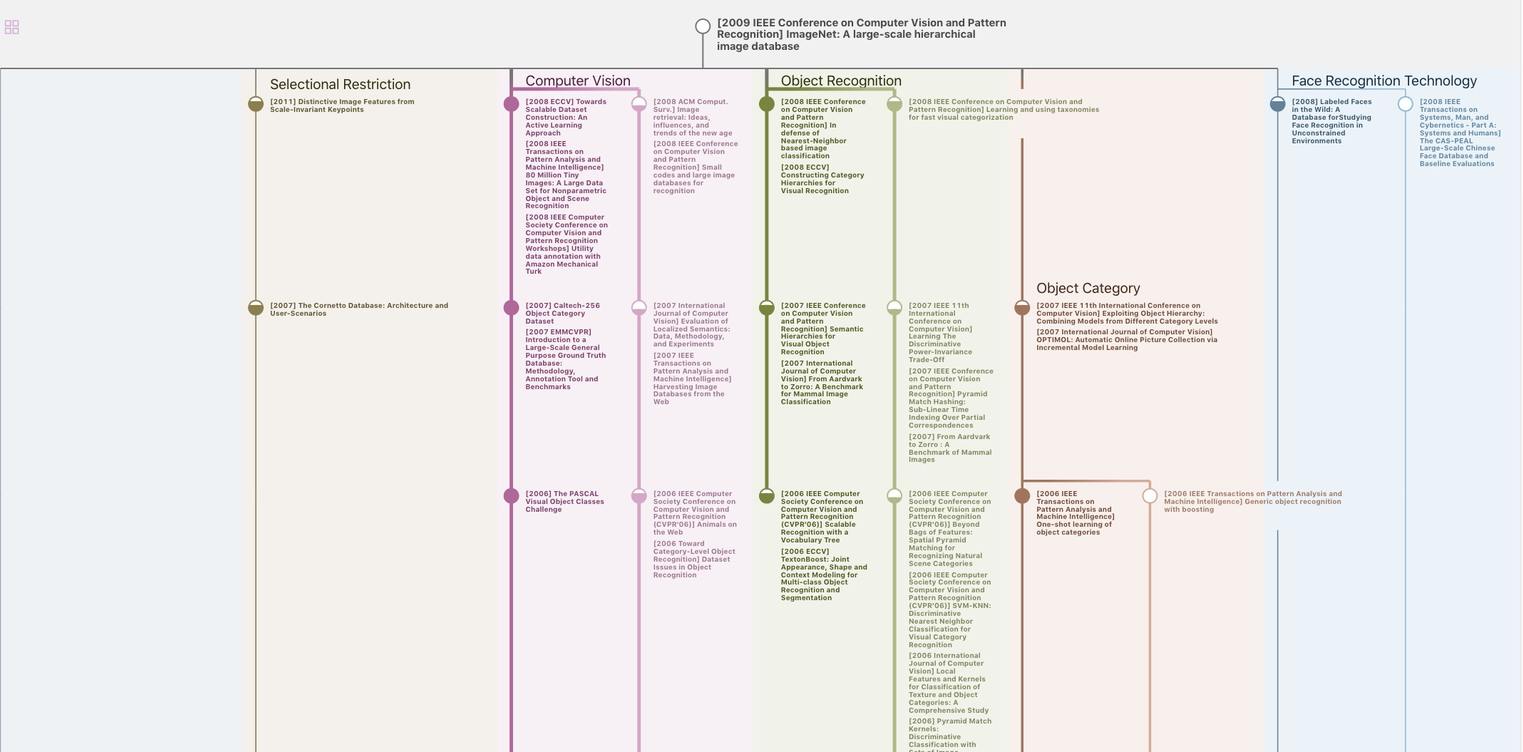
生成溯源树,研究论文发展脉络
Chat Paper
正在生成论文摘要