A Data-Driven Framework for Driving Style Classification.
ADMA (2)(2022)
摘要
Traditional driving behaviour recognition algorithms leverage hand-crafted features extracted from raw driving data and then apply user-defined machine learning models to identify driving behaviours. However, such solutions are limited by the set of selected features and by the chosen model. In this work, we present a data-driven driving behaviour recognition framework that utilizes an unsupervised feature extraction and feature selection algorithm and a deep neural network architecture obtained using an Automated Machine Learning (AutoML) approach. To validate the feasibility of this solution, numerical evaluations were performed on a unique real-world driving datasets collected from 29 professional truck drivers in uncontrolled environments, including supervisor's scoring of driver behavior that is used as ground truth data. Our experimental results show that the proposed deep neural network model achieves up to 95% accuracy for multi-class classification, significantly outperforming five other popular machine learning models.
更多查看译文
关键词
classification,data-driven
AI 理解论文
溯源树
样例
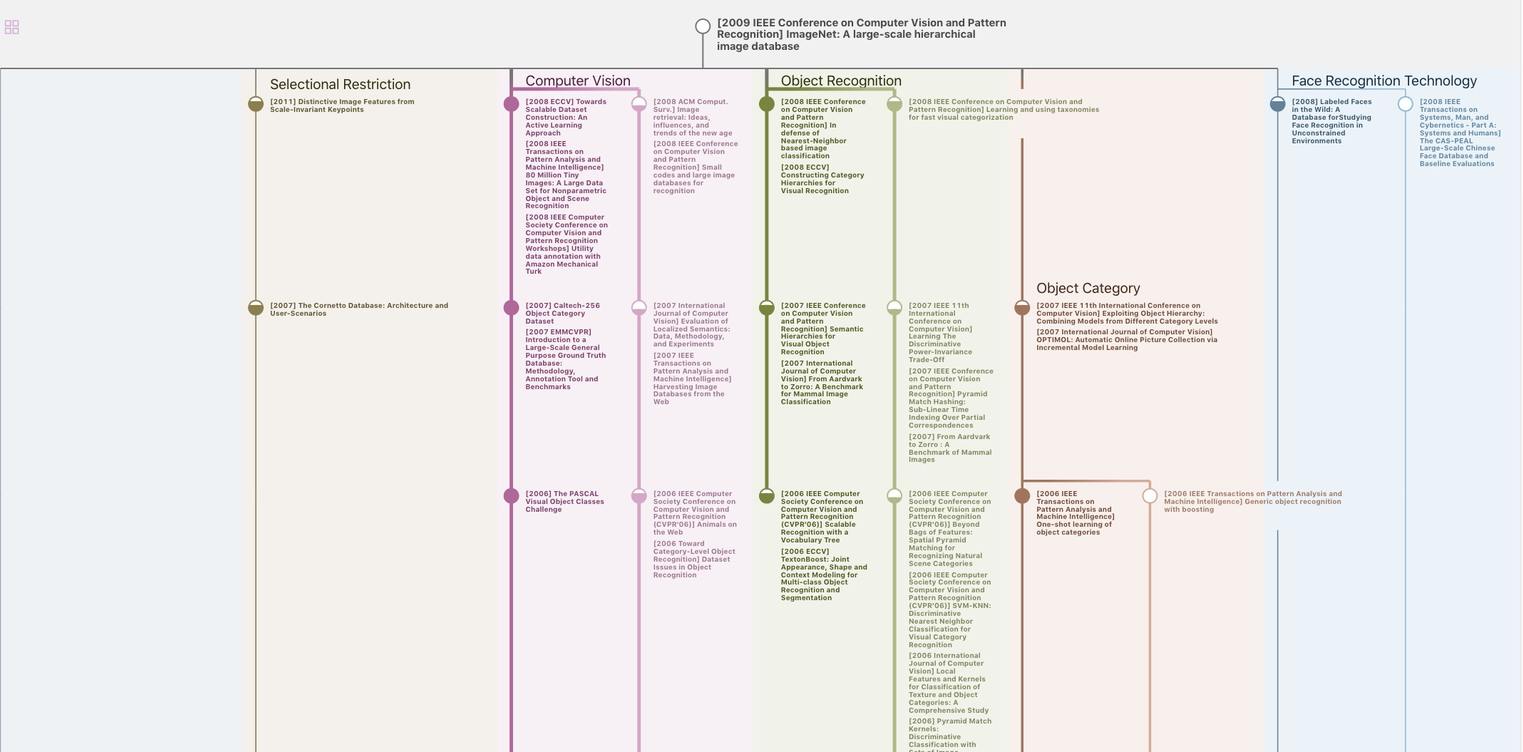
生成溯源树,研究论文发展脉络
Chat Paper
正在生成论文摘要