Context-Aware Neural Confidence Estimation for Rare Word Speech Recognition
2022 IEEE Spoken Language Technology Workshop (SLT)(2023)
摘要
Confidence estimation for automatic speech recognition (ASR) is important for many downstream tasks. Recently, neural confidence estimation models (CEMs) have been shown to produce accurate confidence scores for predicting word-level errors. These models are built on top of an end-to-end (E2E) ASR and the acoustic embeddings are part of the input features. However, practical E2E ASR systems often incorporate contextual information in the decoder to improve rare word recognition. The CEM is not aware of this and underestimates the confidence of the rare words that have been corrected by the context. In this paper, we propose a context-aware CEM by incorporating context into the encoder using a neural associative memory (NAM) model. It uses attention to detect for presence of the biasing phrases and modify the encoder features. Experiments show that the proposed context-aware CEM using NAM augmented training can improve the AUC-ROC for word error prediction from 0.837 to 0.892.
更多查看译文
关键词
Confidence estimation,contextual biasing,end-to-end speech recognition,neural associative memory
AI 理解论文
溯源树
样例
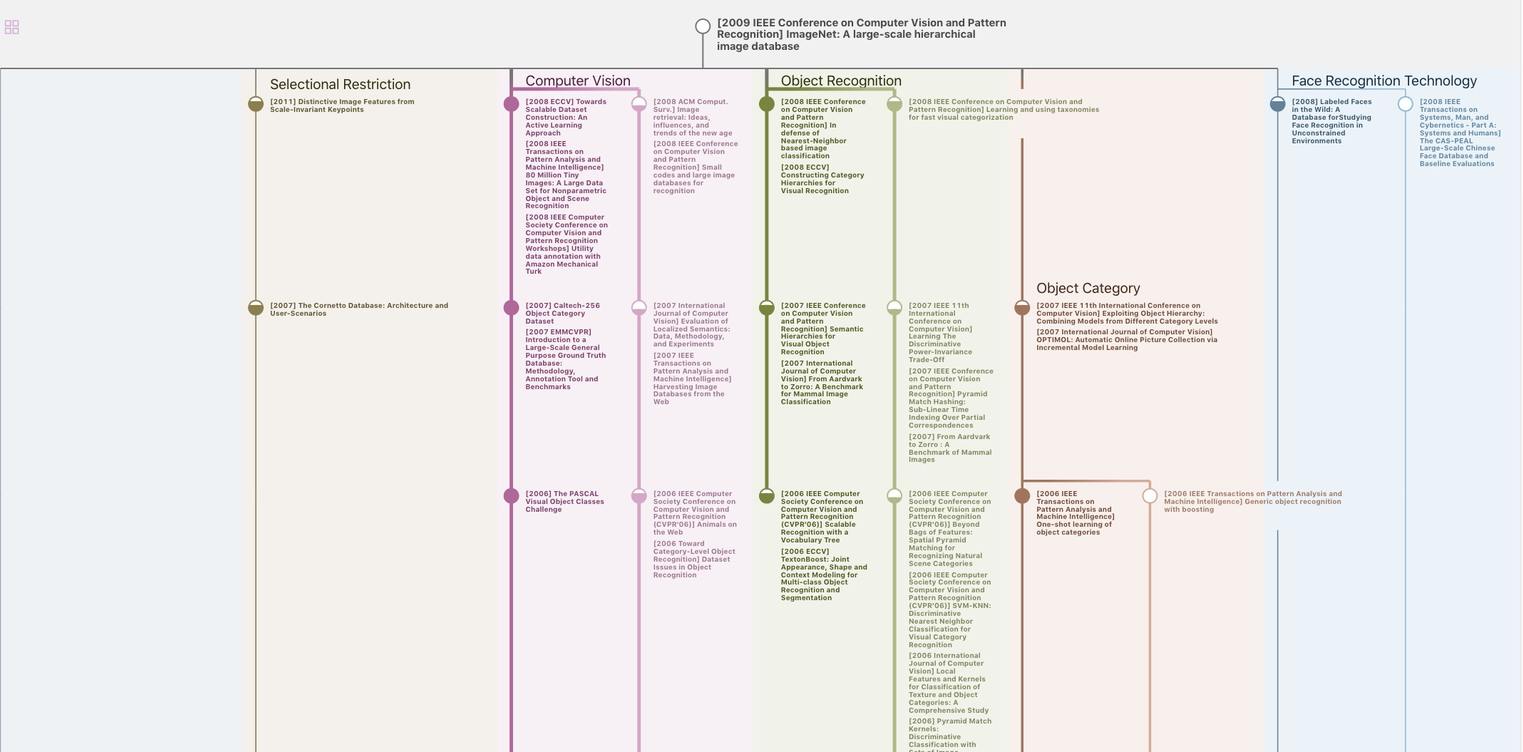
生成溯源树,研究论文发展脉络
Chat Paper
正在生成论文摘要