Joint Optimization of Diffusion Probabilistic-Based Multichannel Speech Enhancement with Far-Field Speaker Verification
2022 IEEE Spoken Language Technology Workshop (SLT)(2023)
摘要
Smart devices using speaker verification are getting equipped with multiple microphones, improving spatial ambiguity and directivity. However, unlike other speech-based applications, the performance of speaker verification degrades in far-field scenarios due to the adverse effects of a noisy environment and room reverberation. This paper presents a novel diffusion probabilistic models-based multichannel speech enhancement as a front-end for the ECAPA-TDNN speaker verification system in a far-field noisy-reverberant scenario. The proposed approach incorporates a two-stage training approach. In the first stage, we individually train the speech enhancement and speaker verification modules. In the second stage, we combined both modules and trained them jointly. We use similarity-preserving knowledge distillation loss that guides the network to produce similar activation for enhanced signals like clean signals. Joint optimization achieved the best results on synthetic and VOiCES datasets.
更多查看译文
关键词
multichannel speech enhancement,far-field speaker verification,deep neural network
AI 理解论文
溯源树
样例
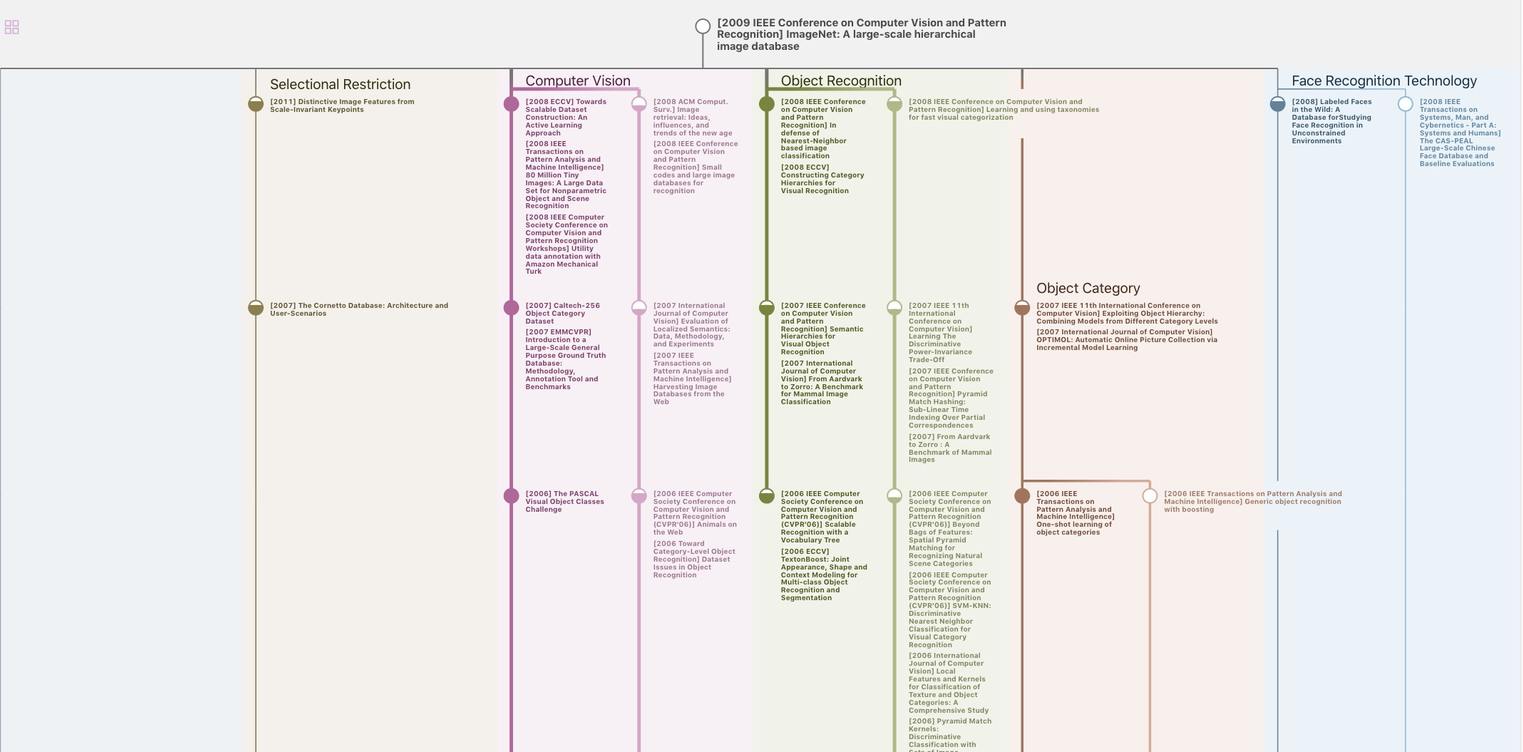
生成溯源树,研究论文发展脉络
Chat Paper
正在生成论文摘要