On the Foundations of Cycles in Bayesian Networks
arxiv(2023)
摘要
Bayesian networks (BNs) are a probabilistic graphical model widely used for representing expert knowledge and reasoning under uncertainty. Traditionally, they are based on directed acyclic graphs that capture dependencies between random variables. However, directed cycles can naturally arise when cross-dependencies between random variables exist, e.g., for modeling feedback loops. Existing methods to deal with such cross-dependencies usually rely on reductions to BNs without cycles. These approaches are fragile to generalize, since their justifications are intermingled with additional knowledge about the application context. In this paper, we present a foundational study regarding semantics for cyclic BNs that are generic and conservatively extend the cycle-free setting. First, we propose constraint-based semantics that specify requirements for full joint distributions over a BN to be consistent with the local conditional probabilities and independencies. Second, two kinds of limit semantics that formalize infinite unfolding approaches are introduced and shown to be computable by a Markov chain construction.
更多查看译文
关键词
cycles,networks
AI 理解论文
溯源树
样例
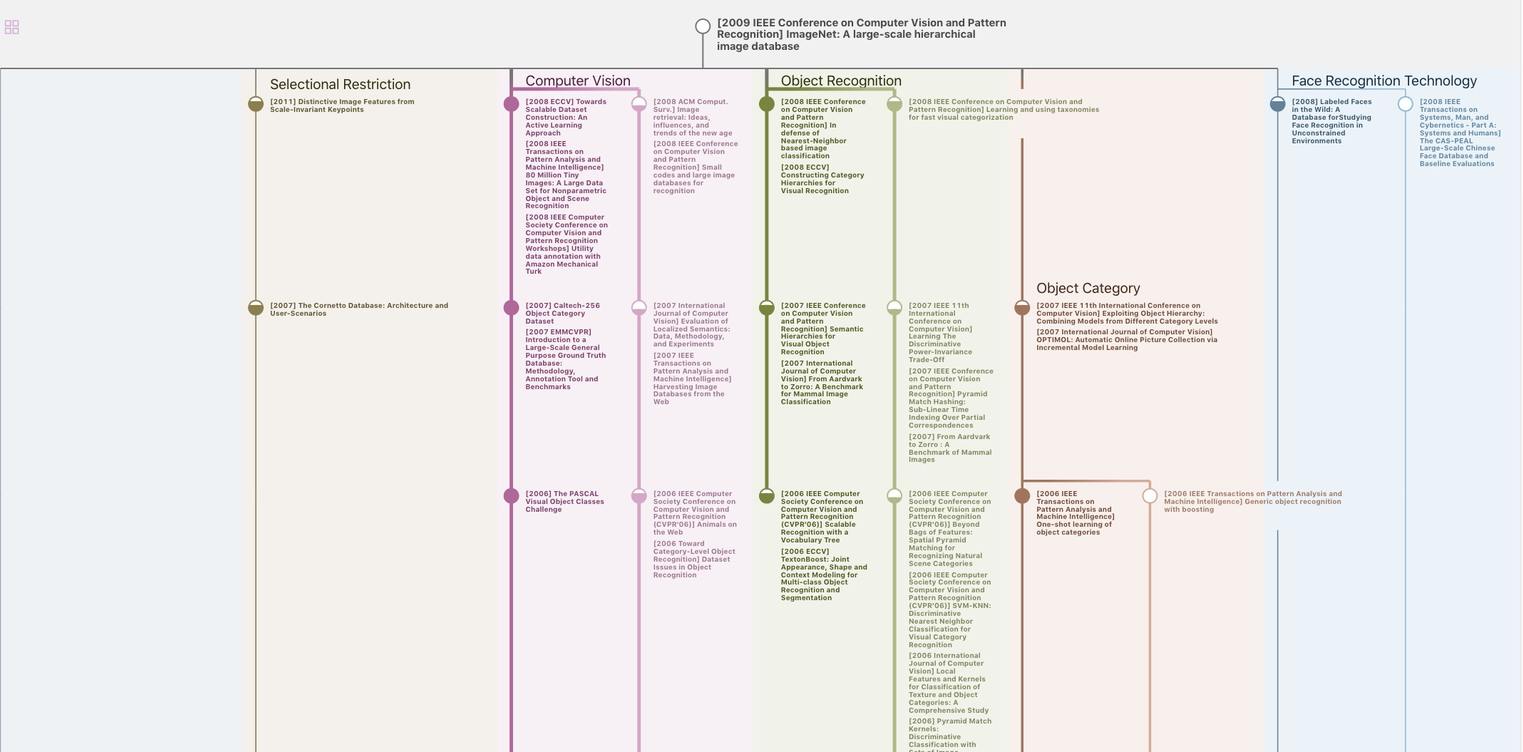
生成溯源树,研究论文发展脉络
Chat Paper
正在生成论文摘要