Padding Module: Learning the Padding in Deep Neural Networks.
IEEE Access(2023)
摘要
During the last decades, many studies have been dedicated to improving the performance of neural networks, for example, the network architectures, initialization, and activation. However, investigating the importance and effects of learnable padding methods in deep learning remains relatively open. To mitigate the gap, this paper proposes a novel trainable Padding Module that can be placed in a deep learning model. The Padding Module can optimize itself without requiring or influencing the model's entire loss function. To train itself, the Padding Module constructs a ground truth and a predictor from the inputs by leveraging the underlying structure in the input data for supervision. As a result, the Padding Module can learn automatically to pad pixels to the border of its input images or feature maps. The padding contents are realistic extensions to its input data and simultaneously facilitate the deep learning model's downstream task. Experiments have shown that the proposed Padding Module outperforms the state-of-the-art competitors and the baseline methods. For example, the Padding Module has 1.23% and 0.44% more classification accuracy than the zero padding when tested on the VGG16 and ResNet50.
更多查看译文
关键词
Deep learning,Neural networks,Convolutional neural networks,Reflection,Filtering algorithms,Feature extraction,Padding module,deep learning,neural networks,trainable padding
AI 理解论文
溯源树
样例
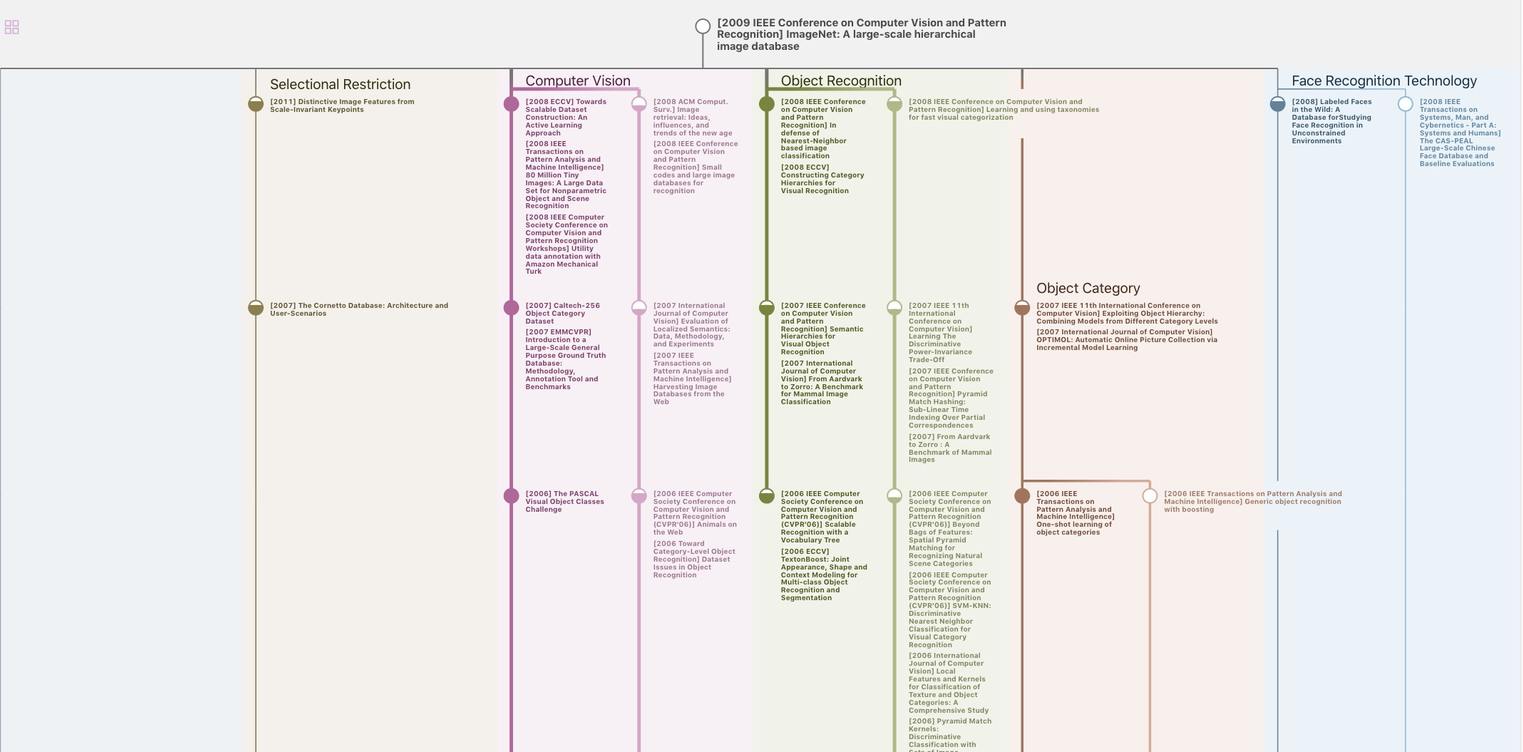
生成溯源树,研究论文发展脉络
Chat Paper
正在生成论文摘要