Multi-Layer Hybrid (MLH) balancing technique: A combined approach to remove data imbalance
Data & Knowledge Engineering(2023)
摘要
Data is one of the most important elements currently for business decisions as well as for scientific research. However, data imbalance is a critical issue that affects the outcome of business decisions or the performance of a model as the decision would be biased towards the majority class (MaC). Existing data balancing techniques have a major drawback: these create new artificial samples randomly which create outliers and hamper the potentiality of the original dataset. In this paper, we propose a Multi-Layer Hybrid (MLH) Balancing Scheme which combines three oversampling techniques in two layers. By combining the characteristics of ADASYN, SVM-SMOTE, and SMOTE+ENN with our data processing techniques, our scheme gives a distributed, noise-free output. It also creates new data points within the range of the original dataset, which keeps the originality of the new data points. Thus, the generated dataset is much suitable for machine learning models to achieve results with higher accuracy for highly imbalanced data. Experimental results on datasets with an imbalance ratio of up to 59 show that our proposed scheme can effectively generate a balanced dataset. We apply the resultant dataset to Random Forest and Artificial Neural Network algorithms; comparison with existing techniques shows that our scheme gives better results.
更多查看译文
关键词
Data imbalance learning,Oversampling,ADASYN,SVM-SMOTE,SMOTE,Data balancing technique
AI 理解论文
溯源树
样例
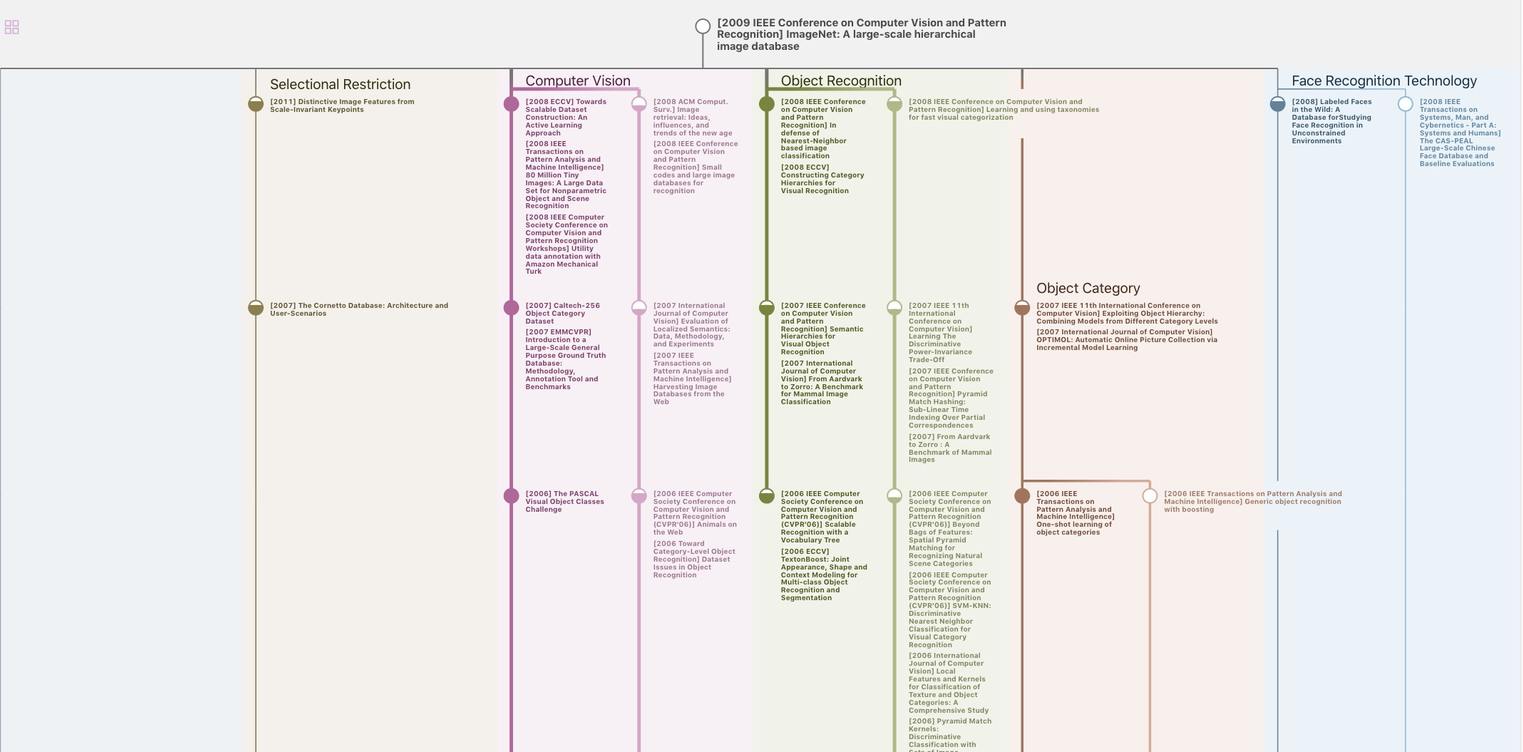
生成溯源树,研究论文发展脉络
Chat Paper
正在生成论文摘要