"It's a Match!" -- A Benchmark of Task Affinity Scores for Joint Learning
arxiv(2023)
摘要
While the promises of Multi-Task Learning (MTL) are attractive, characterizing the conditions of its success is still an open problem in Deep Learning. Some tasks may benefit from being learned together while others may be detrimental to one another. From a task perspective, grouping cooperative tasks while separating competing tasks is paramount to reap the benefits of MTL, i.e., reducing training and inference costs. Therefore, estimating task affinity for joint learning is a key endeavor. Recent work suggests that the training conditions themselves have a significant impact on the outcomes of MTL. Yet, the literature is lacking of a benchmark to assess the effectiveness of tasks affinity estimation techniques and their relation with actual MTL performance. In this paper, we take a first step in recovering this gap by (i) defining a set of affinity scores by both revisiting contributions from previous literature as well presenting new ones and (ii) benchmarking them on the Taskonomy dataset. Our empirical campaign reveals how, even in a small-scale scenario, task affinity scoring does not correlate well with actual MTL performance. Yet, some metrics can be more indicative than others.
更多查看译文
关键词
task affinity scores,benchmark,learning,match!
AI 理解论文
溯源树
样例
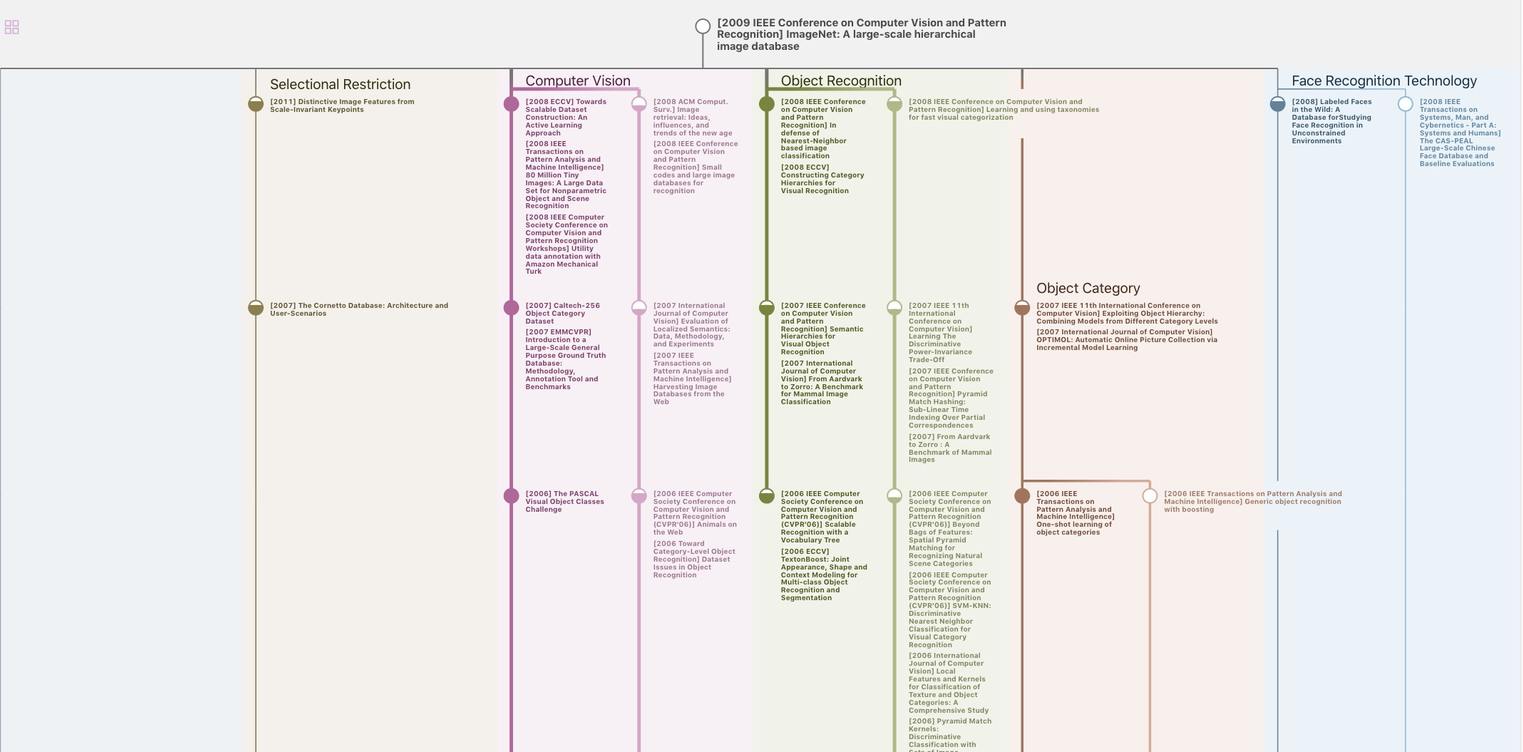
生成溯源树,研究论文发展脉络
Chat Paper
正在生成论文摘要