Accurate, Low-latency, Efficient SAR Automatic Target Recognition on FPGA
2022 32nd International Conference on Field-Programmable Logic and Applications (FPL)(2022)
摘要
Synthetic aperture radar (SAR) automatic target recognition (ATR) is the key technique for remote-sensing image recognition. The state-of-the-art convolutional neural networks (CNNs) for SAR ATR suffer from high computation cost and large memory footprint, making them unsuitable to be deployed on resource-limited platforms, such as small/micro satellites. In this paper, we propose a comprehensive GNN-based model-architecture co-design on FPGA to address the above issues. Model design: we design a novel graph neural network (GNN) for SAR ATR. The proposed GNN model incorporates GraphSAGE layer operators and attention mechanism, achieving comparable accuracy as the state-of-the-art work with near 1/100 computation cost. Then, we propose a pruning approach including weight pruning and input pruning. While weight pruning through lasso regression reduces most parameters without accuracy drop, input pruning eliminates most input pixels with negligible accuracy drop. Architecture design: to fully unleash the computation parallelism within the proposed model, we develop a novel unified hardware architecture that can execute various computation kernels (feature aggregation, feature transformation, graph pooling). The proposed hardware design adopts the Scatter-Gather paradigm to efficiently handle the irregular computation patterns of various computation kernels. We deploy the proposed design on an embedded FPGA (AMD Xilinx ZCU104) and evaluate the performance using MSTAR dataset. Compared with the state-of-the-art CNNs, the proposed GNN achieves comparable accuracy with 1/3258 computation cost and 1/83 model size. Compared with the state-of-the-art CPU/GPU, our FPGA accelerator achieves 14.8×/2.5× speedup (latency) and is 62×/39× more energy efficient.
更多查看译文
关键词
SAR ATR,graph neural network (GNN),hard-ware architecture
AI 理解论文
溯源树
样例
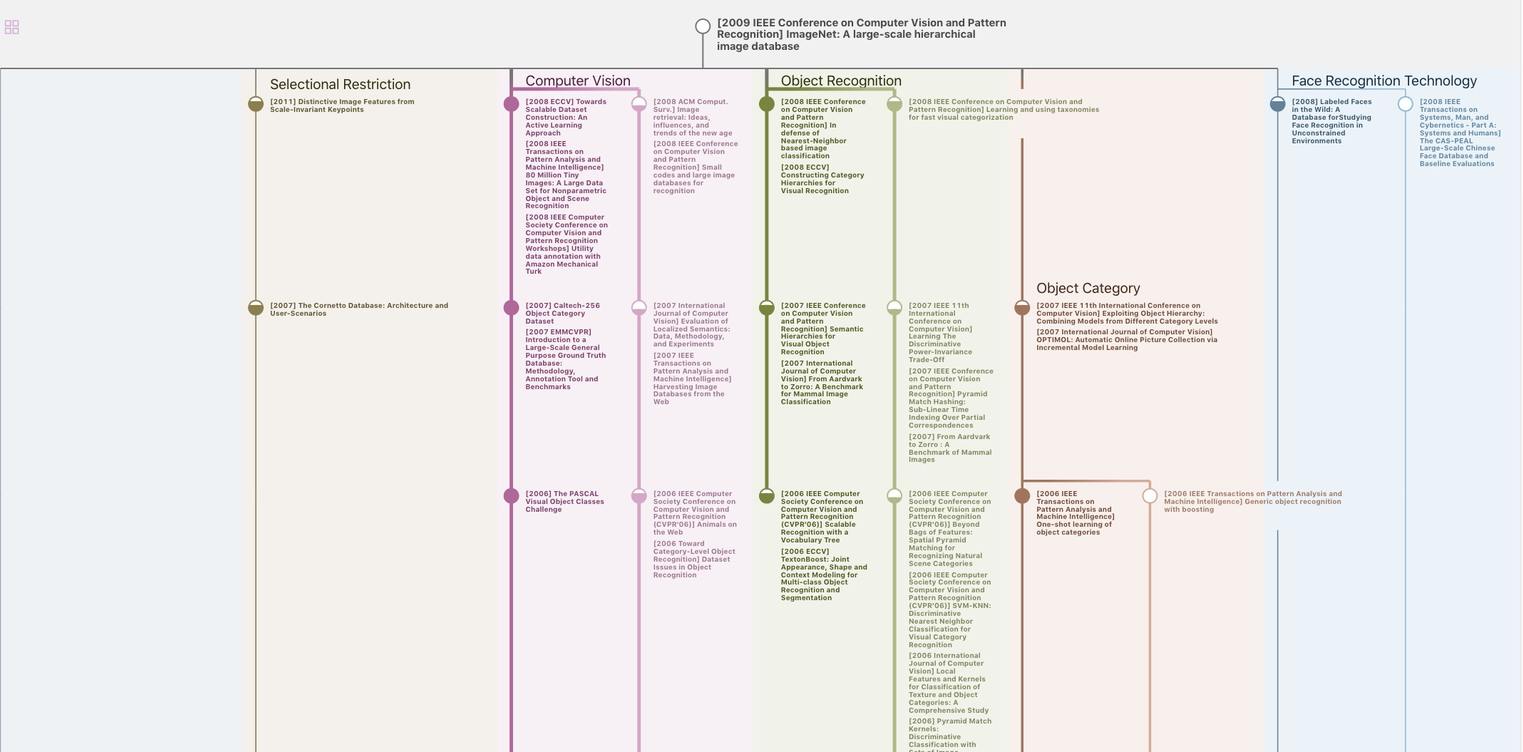
生成溯源树,研究论文发展脉络
Chat Paper
正在生成论文摘要