Deep Attributed Graph Embeddings.
Modeling Decisions for Artificial Intelligence (MDAI)(2022)
摘要
Graph Representation Learning aims to learn a rich and low-dimensional node embedding while preserving the graph properties. In this paper, we propose a novel Deep Attributed Graph Embedding (DAGE) that learns node representations based on both the topological structure and node attributes. DAGE a is able to capture, in a linear time and with a limited number of trainable parameters, the highly non-linear properties of attributed graphs. The proposed approach outperforms the current state-of-the-art approaches on node classification and node clustering tasks at a lower computational costs.
更多查看译文
关键词
Attributed Graph Embedding,Semantic proximity,Structural proximity
AI 理解论文
溯源树
样例
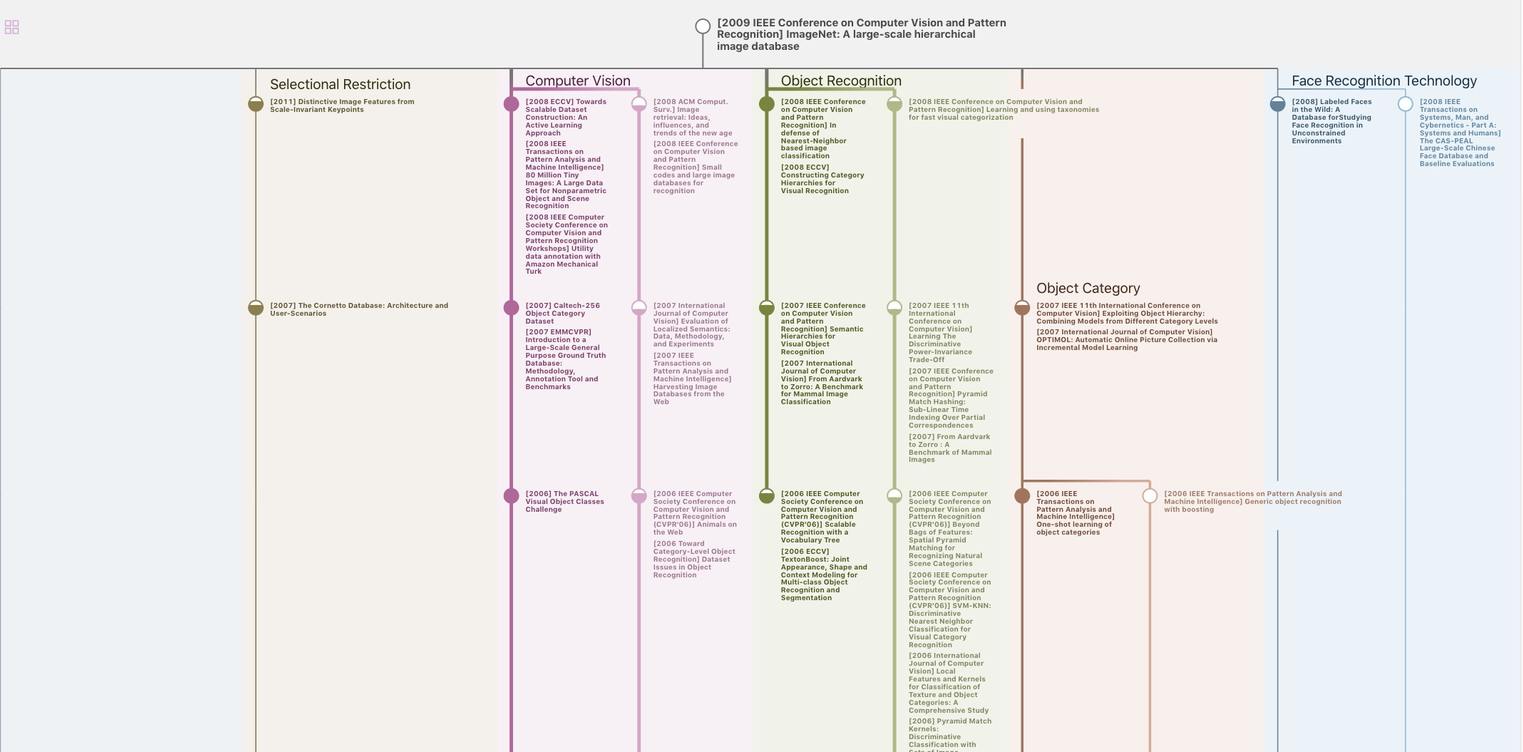
生成溯源树,研究论文发展脉络
Chat Paper
正在生成论文摘要