FSP: Towards Flexible Synchronous Parallel Frameworks for Distributed Machine Learning
IEEE Transactions on Parallel and Distributed Systems(2023)
摘要
Myriad of machine learning (ML) algorithms refine model parameters iteratively. Existing synchronous data-parallel frameworks can accelerate training with convergence guarantees. However, the pre-assigned workload-based synchronous design still poses great challenges, since fast workers must wait for slow, straggling ones, especially in a heterogeneous computing cluster. Asynchronous alternatives can bypass this performance bottleneck, but at expense of potentially losing convergence guarantees. This article proposes a new time-based flexible synchronous parallel framework (FSP). It provides strict convergence analysis by consistently updating parameters, as well as significant cost reduction by completely unleashing the power of fast workers. It identifies the optimal synchronization frequency, by online balancing costs of parameters' update and benefits brought by their freshness. Besides the basic goal of keeping all workers fully CPU-utilized, FSP also aims to keep data spread over the cluster fully utilized, so that they can contribute to convergence with equal opportunities. These proposals are all implemented in a prototype system Flegel, with additional engineering optimizations for further performance enhancement and programming facilitation. Experiments demonstrate that Flegel significantly outperforms recent studies.
更多查看译文
关键词
Machine learning,distributed computation,synchronous parallel model,straggler,workload balance
AI 理解论文
溯源树
样例
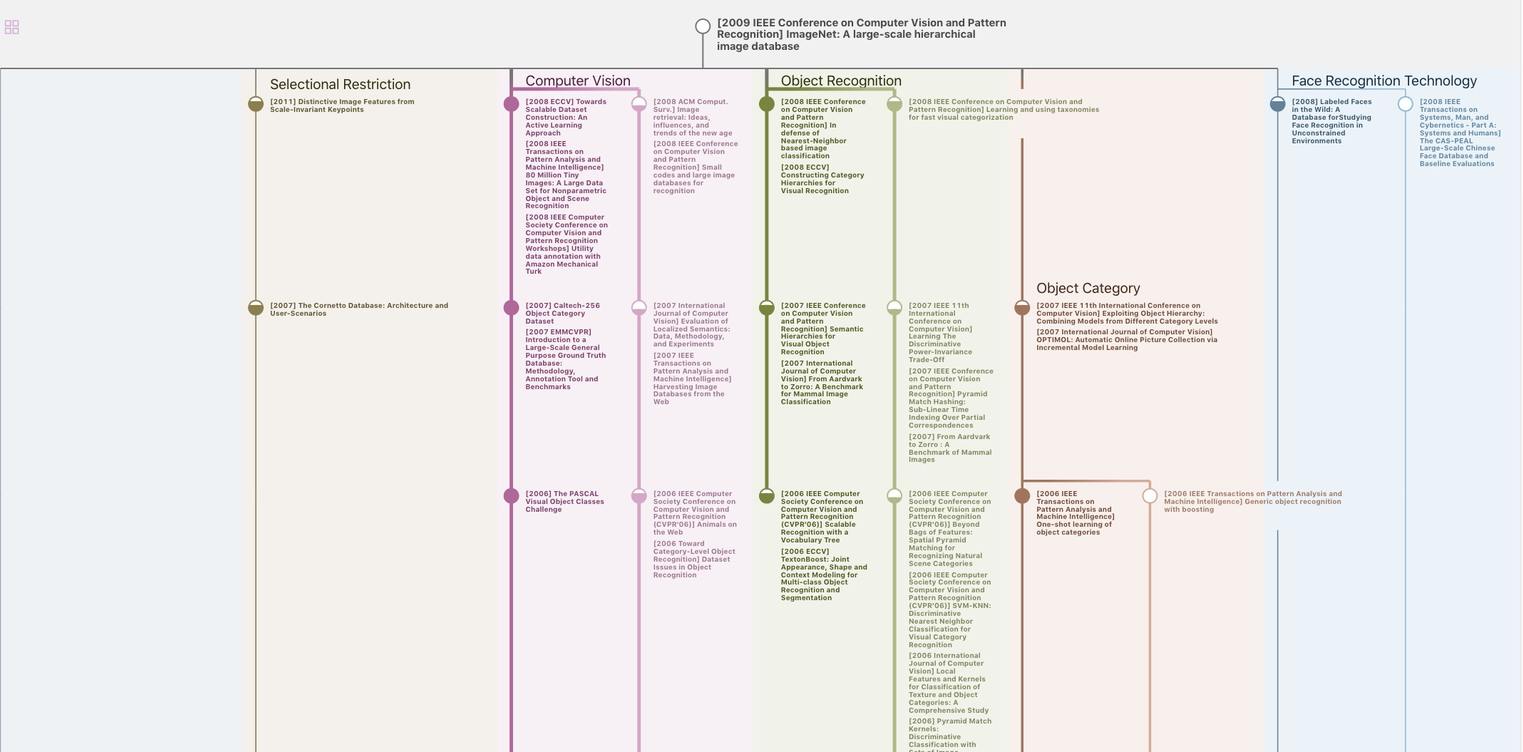
生成溯源树,研究论文发展脉络
Chat Paper
正在生成论文摘要