CausalDialogue: Modeling Utterance-level Causality in Conversations
conf_acl(2022)
摘要
Despite their widespread adoption, neural conversation models have yet to exhibit natural chat capabilities with humans. In this research, we examine user utterances as causes and generated responses as effects, recognizing that changes in a cause should produce a different effect. To further explore this concept, we have compiled and expanded upon a new dataset called CausalDialogue through crowd-sourcing. This dataset includes multiple cause-effect pairs within a directed acyclic graph (DAG) structure. Our analysis reveals that traditional loss functions can struggle to effectively incorporate the DAG structure, leading us to propose a causality-enhanced method called Exponential Maximum Average Treatment Effect (ExMATE) to enhance the impact of causality at the utterance level in training neural conversation models. To evaluate the effectiveness of this approach, we have built a comprehensive benchmark using the CausalDialogue dataset leveraging large-scale pre-trained language models, and have assessed the results through both human and automatic evaluation metrics for coherence, diversity, and agility. Our findings show that current techniques are still unable to effectively address conversational DAGs, and that the ExMATE method can improve the diversity and agility of conventional loss functions while maintaining coherence.
更多查看译文
关键词
utterance-level
AI 理解论文
溯源树
样例
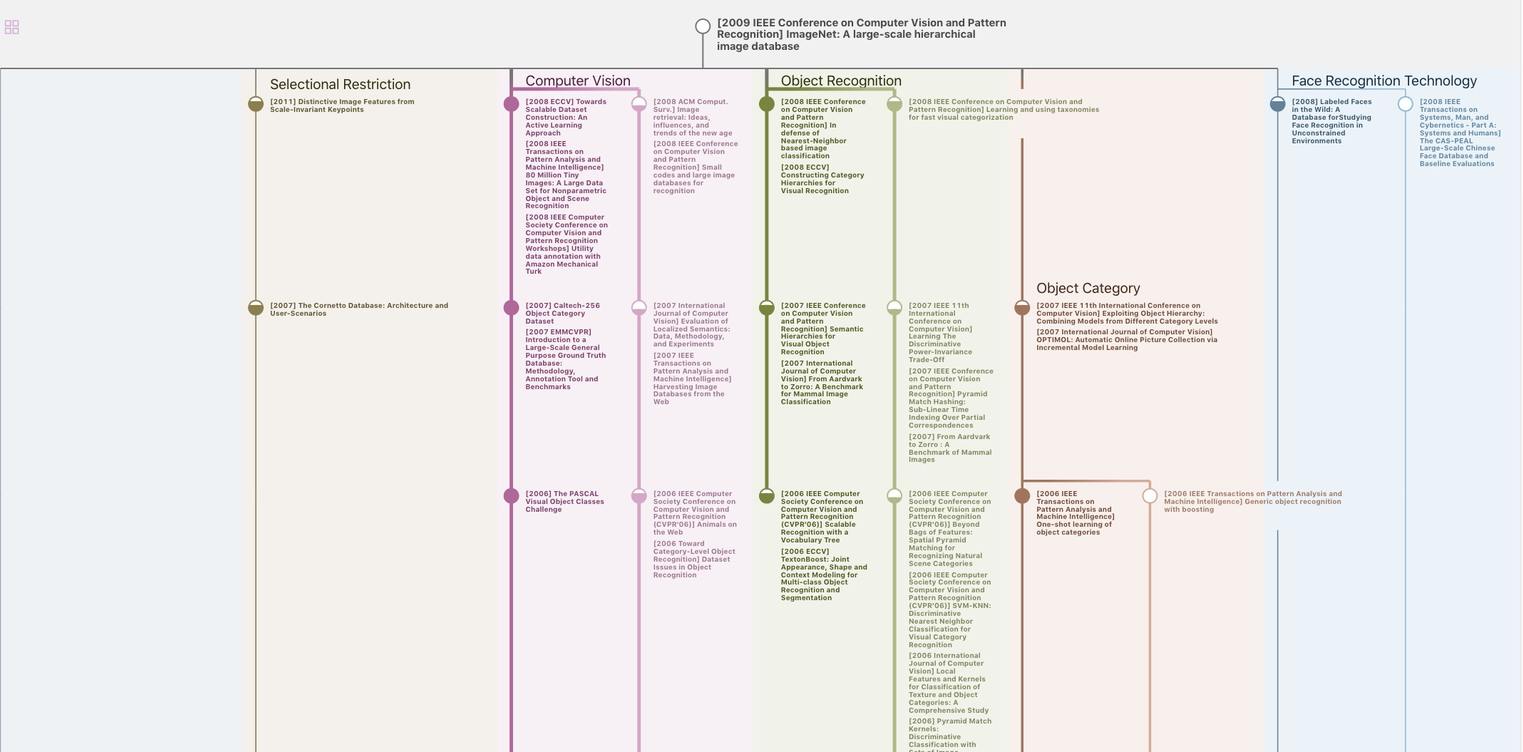
生成溯源树,研究论文发展脉络
Chat Paper
正在生成论文摘要