Multi-Head Uncertainty Inference for Adversarial Attack Detection
arxiv(2023)
摘要
Deep neural networks (DNNs) are sensitive and susceptible to tiny perturbations by adversarial attacks which cause erroneous predictions. Various methods, including adversarial defense and uncertainty inference (UI), have been developed to overcome adversarial attacks in recent years. In this paper, we propose a multi-head uncertainty inference (MH-UI) framework for detecting adversarial attack examples. We adopt a multi-head architecture with multiple prediction heads (i.e., classifiers) to obtain predictions from different depths in the DNNs and introduce shallow information for the UI. Using independent heads at different depths, the normalized predictions are assumed to follow the same Dirichlet distribution, and we estimate the distribution parameter of it by moment matching. Cognitive uncertainty brought by the adversarial attacks will be reflected and amplified in the distribution. Experimental results show that the proposed MH-UI framework has good performance in different settings of adversarial attack detection tasks.
更多查看译文
关键词
Uncertainty inference,adversarial attack detection,image recognition,Dirichlet distribution
AI 理解论文
溯源树
样例
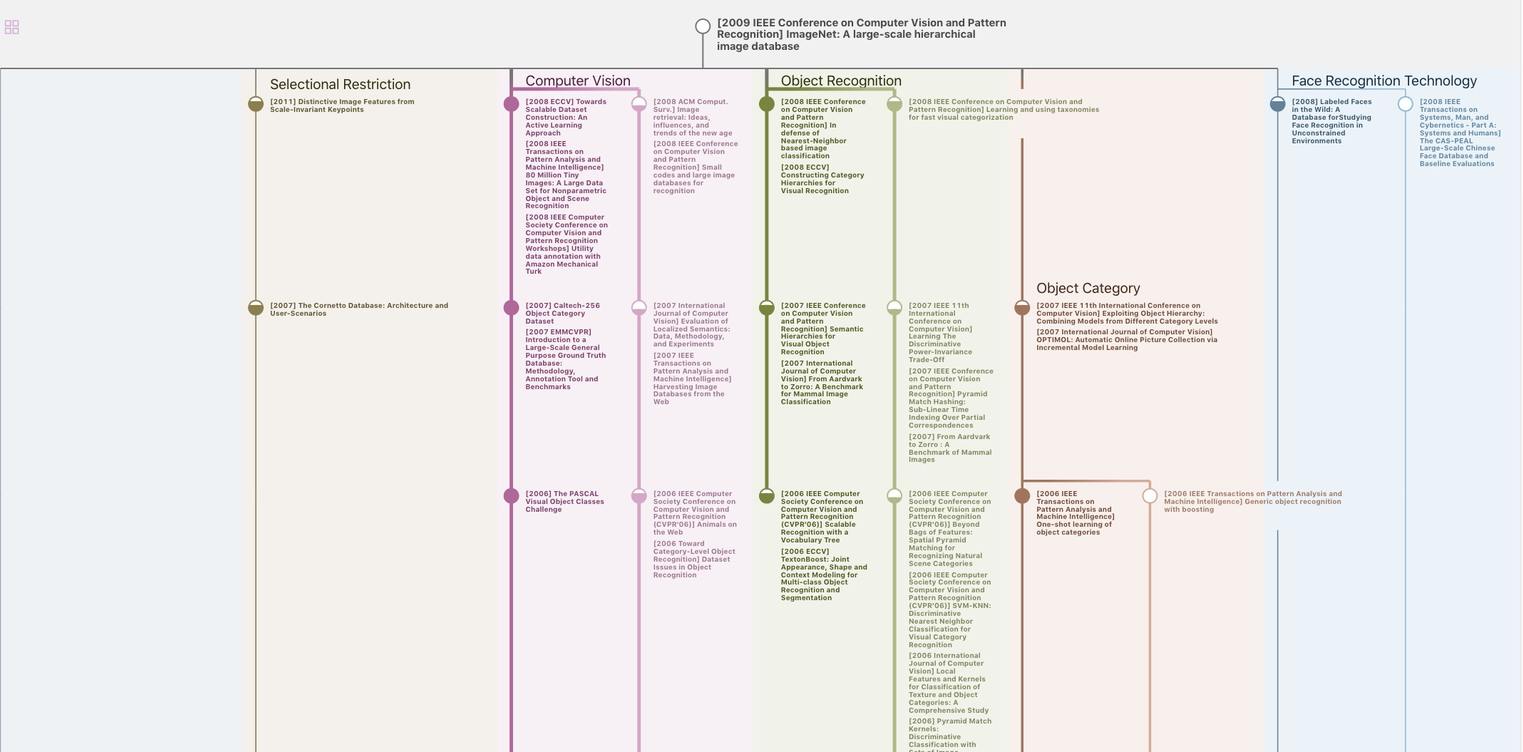
生成溯源树,研究论文发展脉络
Chat Paper
正在生成论文摘要