Privacy-Preserving Collaborative Learning Through Feature Extraction
IEEE TRANSACTIONS ON DEPENDABLE AND SECURE COMPUTING(2024)
摘要
We propose a framework in which multiple entities collaborate to build a machine learning model while preserving privacy of their data. The approach utilizes feature embeddings from shared/per-entity feature extractors transforming data into a feature space for cooperation between entities. We propose two specific methods and compare them with a baseline method. In Shared Feature Extractor (SFE) Learning, the entities use a shared feature extractor to compute feature embeddings of samples. In Locally Trained Feature Extractor (LTFE) Learning, each entity uses a separate feature extractor, and models are trained using concatenated features from all entities. As a baseline, in Cooperatively Trained Feature Extractor (CTFE) Learning, the entities train models by sharing raw data. Secure multi-party algorithms are utilized to train models without revealing data or features in plain text. We investigate the trade-offs among SFE, LTFE, and CTFE in regard to performance, privacy leakage (using an off-the-shelf membership inference attack), and computational cost. LTFE provides the most privacy, followed by SFE, and then CTFE. Computational cost is lowest for SFE and the relative speed of CTFE and LTFE depends on network architecture. CTFE and LTFE provide the best accuracy. We use three different datasets for evaluations.
更多查看译文
关键词
Feature extraction,Cryptography,Training,Servers,Computational modeling,Protocols,Data models,Collaborative learning,privacy-preserving training,secure multiparty computation,neural networks,feature extractor
AI 理解论文
溯源树
样例
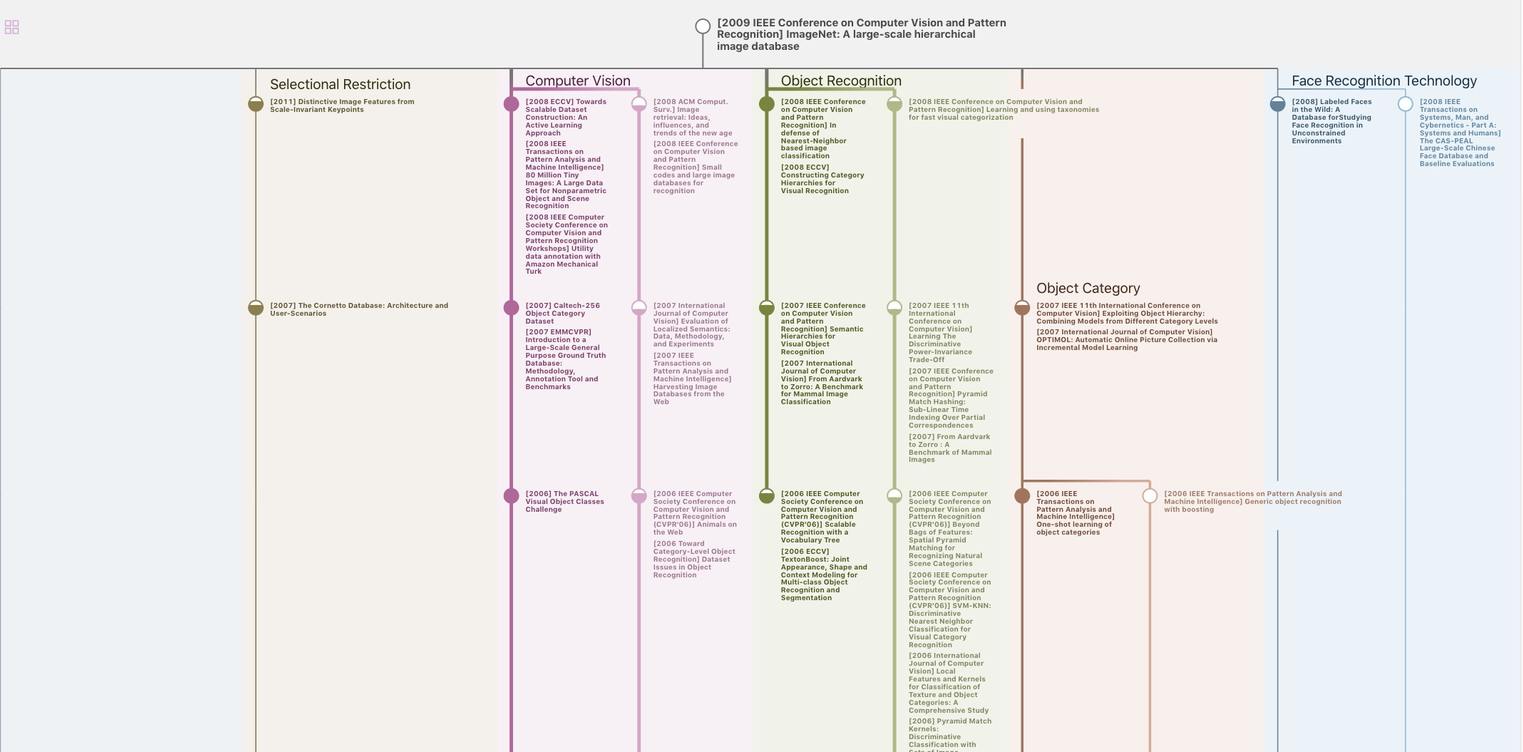
生成溯源树,研究论文发展脉络
Chat Paper
正在生成论文摘要