Dynamic provisioning of airport resources for inbound passenger flow using reinforcement learning
2022 IEEE 25th International Conference on Intelligent Transportation Systems (ITSC)(2022)
摘要
Correct provisioning of resources can help airports reduce cost of operations while ensuring quality of service seen by passengers. Specifically, dynamic provisioning of resources is needed to handle varying passenger loads that are subject to increased uncertainties due to the pandemic. Classical optimization methods could be computationally expensive; and rule-based heuristics that are well-tuned for normal operating conditions could be sub-optimal during periods of rapid change. We complement existing works with a Reinforcement Learning (RL)-based approach for dynamic provisioning of airport resources. Specifically, we consider the problem of jointly provisioning immigration and medical screening resources for inbound passenger flow. Our approach uses a state representation that encodes the supply and demand of the provisioned resources; and a reward engineered to capture the resource cost vs passenger delay trade-off. As baselines, we use two heuristics that statically and dynamically provision resources. We evaluate our approach on a simulated airport environment calibrated with real-world data. In scenarios that statistically significantly differ from the learning dataset, RL achieves up to ~20X decrease in passenger delay when compared to the heuristics for up to ~1.6X increase in resource cost.
更多查看译文
关键词
dynamic provisioning,airport resources,inbound passenger flow,reinforcement learning,varying passenger loads,optimization methods,immigration,medical screening resources,resource cost,passenger delay trade-off,simulated airport environment
AI 理解论文
溯源树
样例
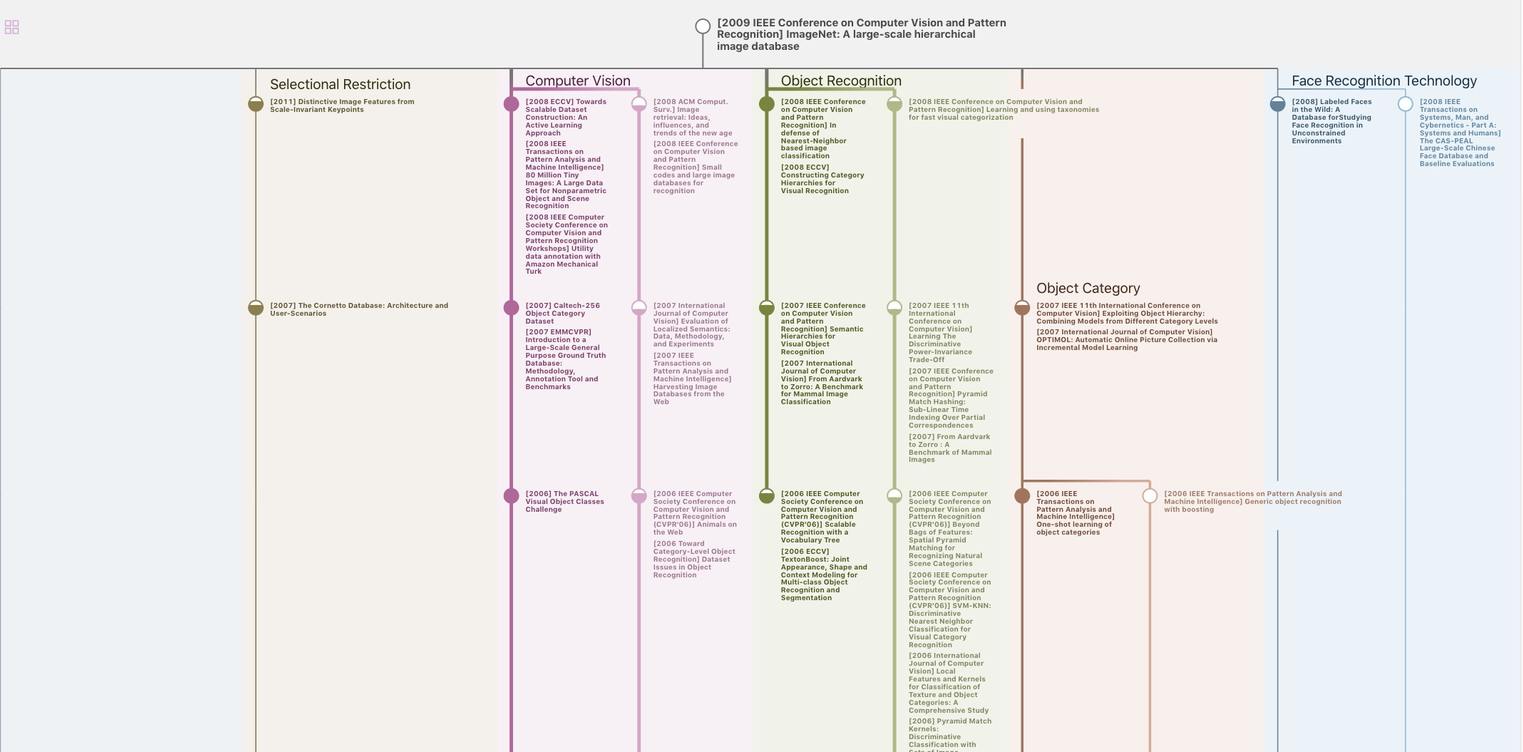
生成溯源树,研究论文发展脉络
Chat Paper
正在生成论文摘要