A3T: accuracy aware adversarial training
arxiv(2023)
摘要
Adversarial training has been empirically shown to be more prone to overfitting than standard training. The exact underlying reasons are still not fully understood. In this paper, we identify one cause of overfitting related to current practices of generating adversarial examples from misclassified samples. We show that, following current practice, adversarial examples from misclassified samples results in harder-to-classify samples than the original ones. This leads to a complex adjustment of the decision boundary during training and hence overfitting. To mitigate this issue, we propose A3T, an accuracy aware AT method that generate adversarial example differently for misclassified and correctly classified samples. We show that our approach achieves better generalization while maintaining comparable robustness to state-of-the-art AT methods on a wide range of computer vision, natural language processing, and tabular tasks.
更多查看译文
关键词
Adversarial training,Overfitting in adversarial training,Accuracy aware adversarial training
AI 理解论文
溯源树
样例
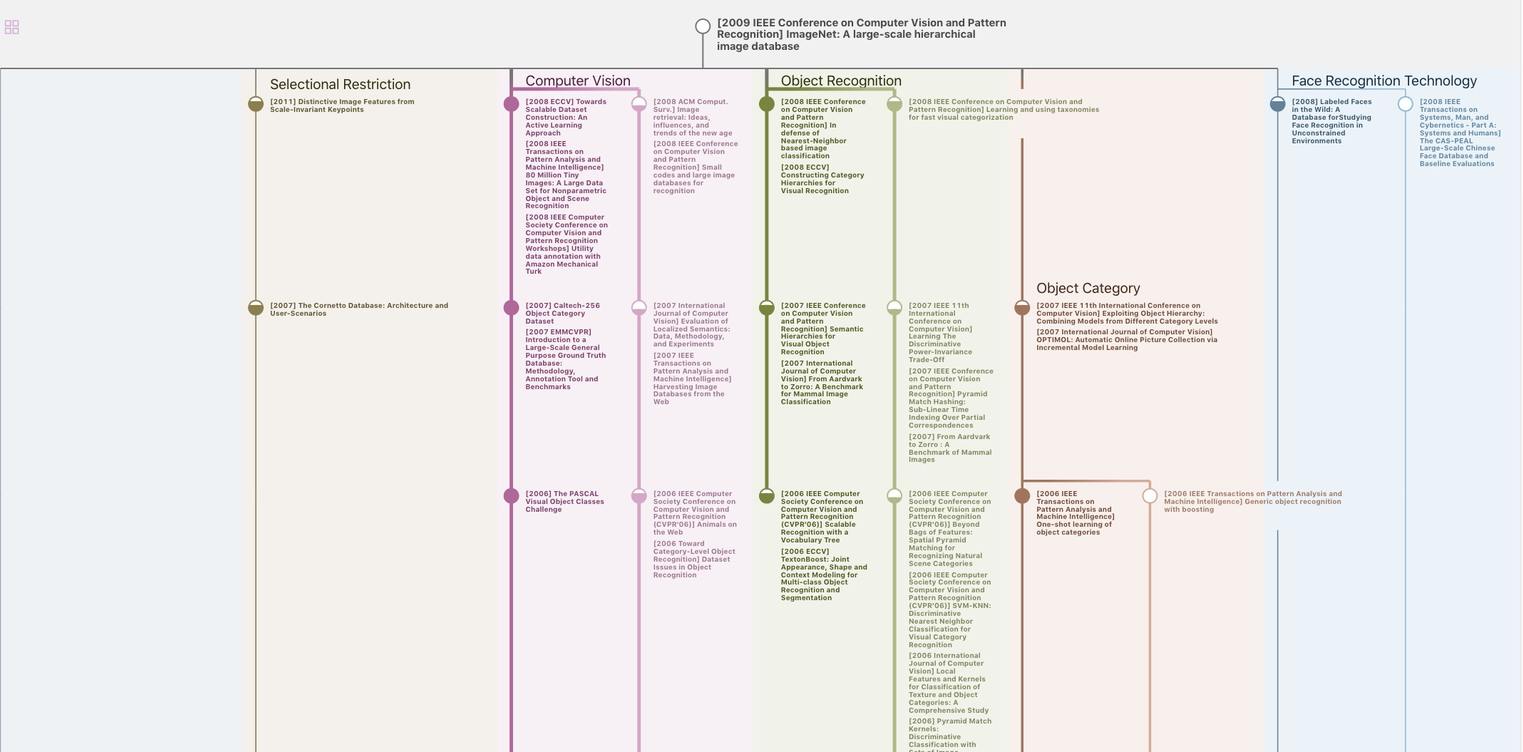
生成溯源树,研究论文发展脉络
Chat Paper
正在生成论文摘要