Attacking Image Splicing Detection and Localization Algorithms Using Synthetic Traces
IEEE TRANSACTIONS ON INFORMATION FORENSICS AND SECURITY(2024)
摘要
Recent advances in deep learning have enabled forensics researchers to develop a new class of image splicing detection and localization algorithms. These algorithms identify spliced content by detecting localized inconsistencies in forensic traces using Siamese neural networks, either explicitly during analysis or implicitly during training. At the same time, deep learning has enabled new forms of anti-forensic attacks, such as adversarial examples and generative adversarial network (GAN) based attacks. Thus far, however, no anti-forensic attack has been demonstrated against image splicing detection and localization algorithms. In this paper, we propose a new GAN-based anti-forensic attack that is able to fool state-of-the-art splicing detection and localization algorithms such as EXIF-Net, Noiseprint, and Forensic Similarity Graphs. This attack operates by adversarially training an anti-forensic generator against a set of Siamese neural networks so that it is able to create synthetic forensic traces. Under analysis, these synthetic traces appear authentic and are self-consistent throughout an image. Through a series of experiments, we demonstrate that our attack is capable of fooling forensic splicing detection and localization algorithms without introducing visually detectable artifacts into an attacked image. Additionally, we demonstrate that our attack outperforms existing alternative attack approaches.
更多查看译文
关键词
Forensics,Splicing,Location awareness,Training,Feature extraction,Detectors,Generators,Anti-forensics,adversarial attacks,generative adversarial networks,splicing detection and localization
AI 理解论文
溯源树
样例
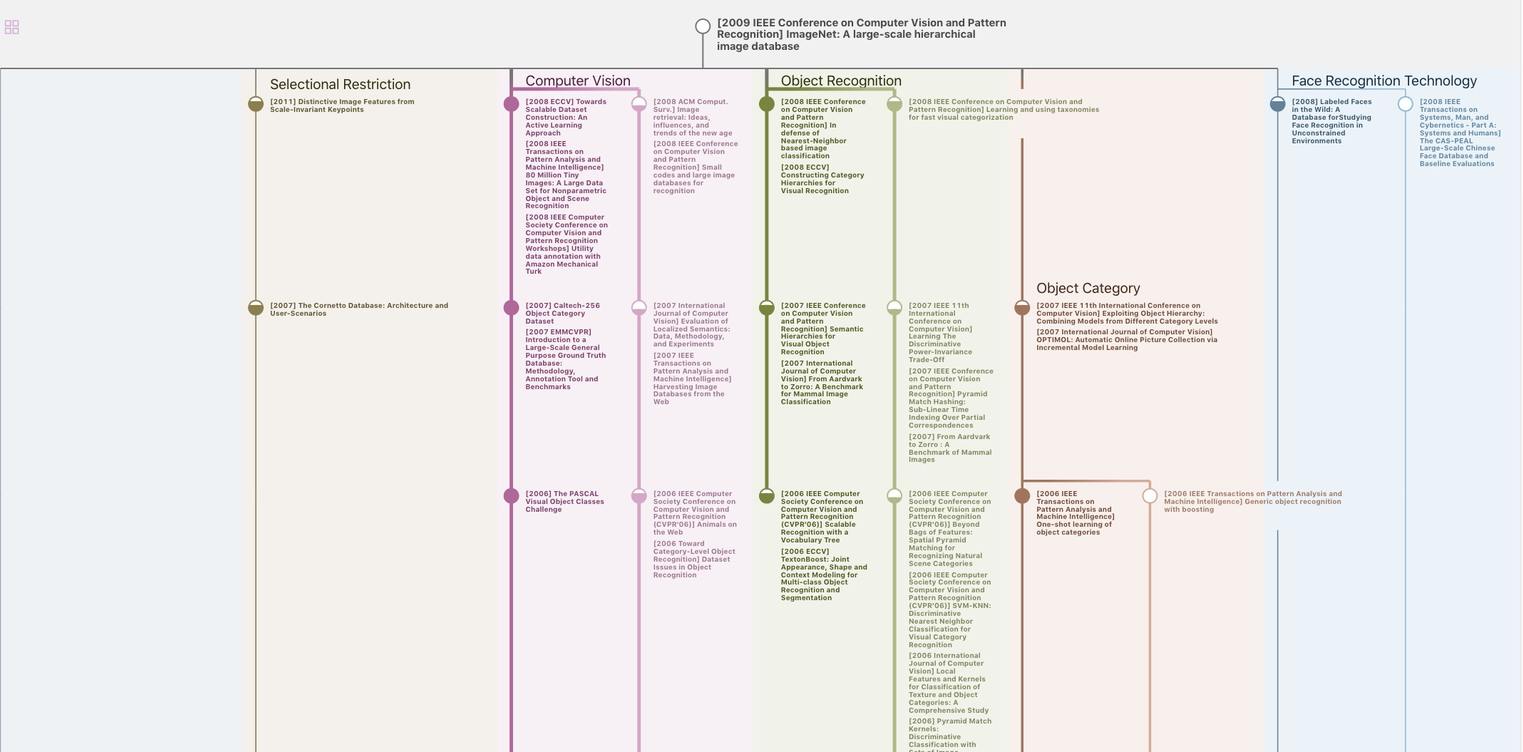
生成溯源树,研究论文发展脉络
Chat Paper
正在生成论文摘要