Classifying Anomalous Members in a Collection of Multivariate Time Series Data Using Large Deviations Principle: An Application to COVID-19 Data
COMPUTATIONAL SCIENCE - ICCS 2022, PT I(2022)
摘要
Anomaly detection for time series data is often aimed at identifying extreme behaviors within an individual time series. However, identifying extreme trends relative to a collection of other time series is of significant interest, like in the fields of public health policy, social justice and pandemic propagation. We propose an algorithm that can scale to large collections of time series data using the concepts from the theory of large deviations. Exploiting the ability of the algorithm to scale to high-dimensional data, we propose an online anomaly detection method to identify anomalies in a collection of multivariate time series. We demonstrate the applicability of the proposed Large Deviations Anomaly Detection (LAD) algorithm in identifying counties in the United States with anomalous trends in terms of COVID-19 related cases and deaths. Several of the identified anomalous counties correlate with counties with documented poor response to the COVID pandemic.
更多查看译文
关键词
Large deviations, Anomaly detection, High-dimensional data, Multivariate time series, Time series database
AI 理解论文
溯源树
样例
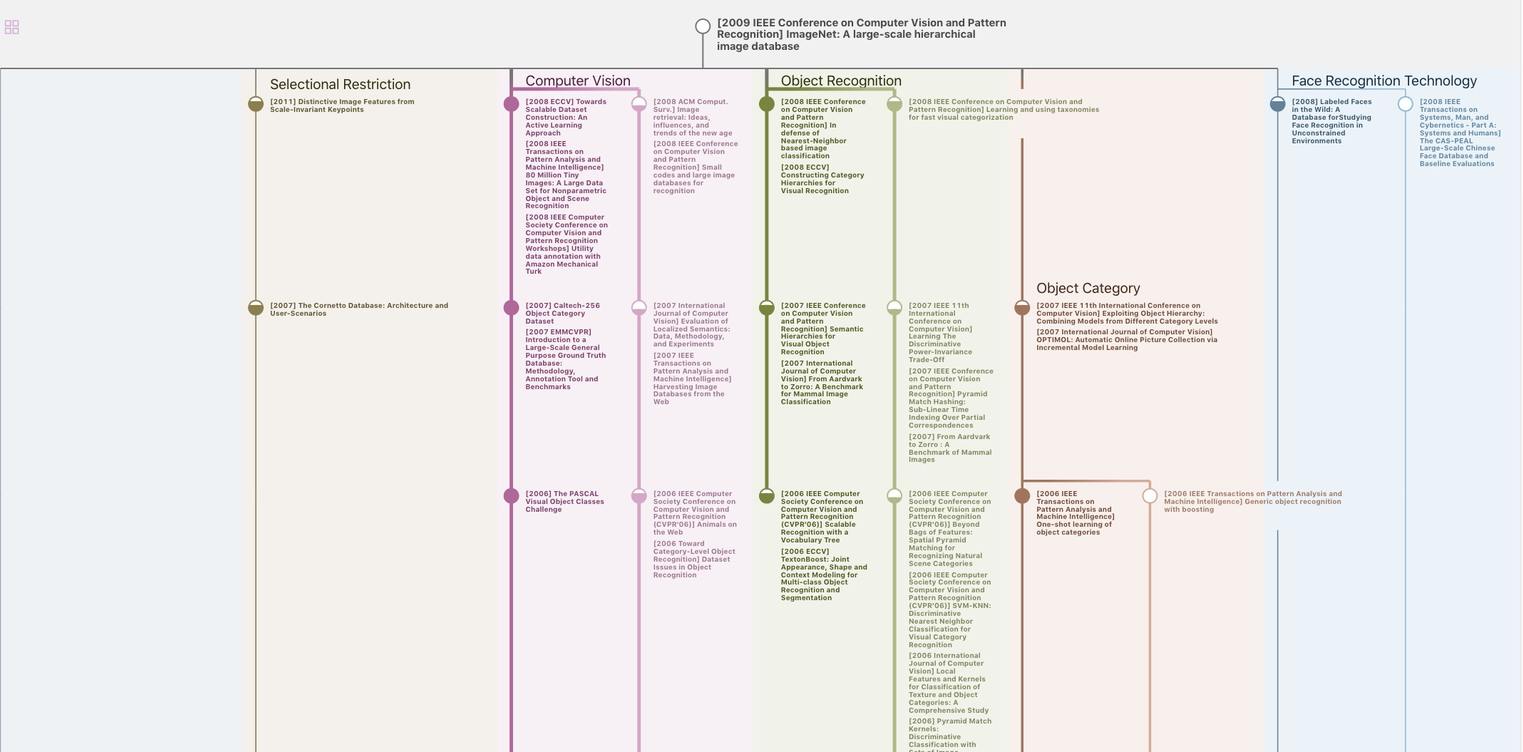
生成溯源树,研究论文发展脉络
Chat Paper
正在生成论文摘要