ShaSTA: Modeling Shape and Spatio-Temporal Affinities for 3D Multi-Object Tracking
IEEE ROBOTICS AND AUTOMATION LETTERS(2024)
摘要
Multi-object tracking (MOT) is a cornerstone capability of any robotic system. Tracking quality is largely dependent on the quality of input detections. In many applications, such as autonomous driving, it is preferable to over-detect objects to avoid catastrophic outcomes due to missed detections. As a result, current state-of-the-art 3D detectors produce high rates of false-positives to ensure a low number of false-negatives. This can negatively affect tracking by making data association and track lifecycle management more challenging. Additionally, occasional false-negative detections due to difficult scenarios like occlusions can harm tracking performance. To address these issues in a unified framework, we propose ShaSTA which learns shape and spatio-temporal affinities between tracks and detections in consecutive frames. The affinity is a probabilistic matching that leads to robust data association, track lifecycle management, false-positive elimination, false-negative propagation, and sequential track confidence refinement. We offer the first self-contained framework that addresses all aspects of the 3D MOT problem. We quantitatively evaluate ShaSTA on the nuScenes tracking benchmark with 5 metrics, including the most common tracking accuracy metric called AMOTA, to demonstrate how ShaSTA may impact the ultimate goal of an autonomous mobile agent. ShaSTA achieves 1st place amongst LiDAR-only trackers that use CenterPoint detections.
更多查看译文
关键词
Computer vision for transportation,deep learning for visual perception,visual tracking
AI 理解论文
溯源树
样例
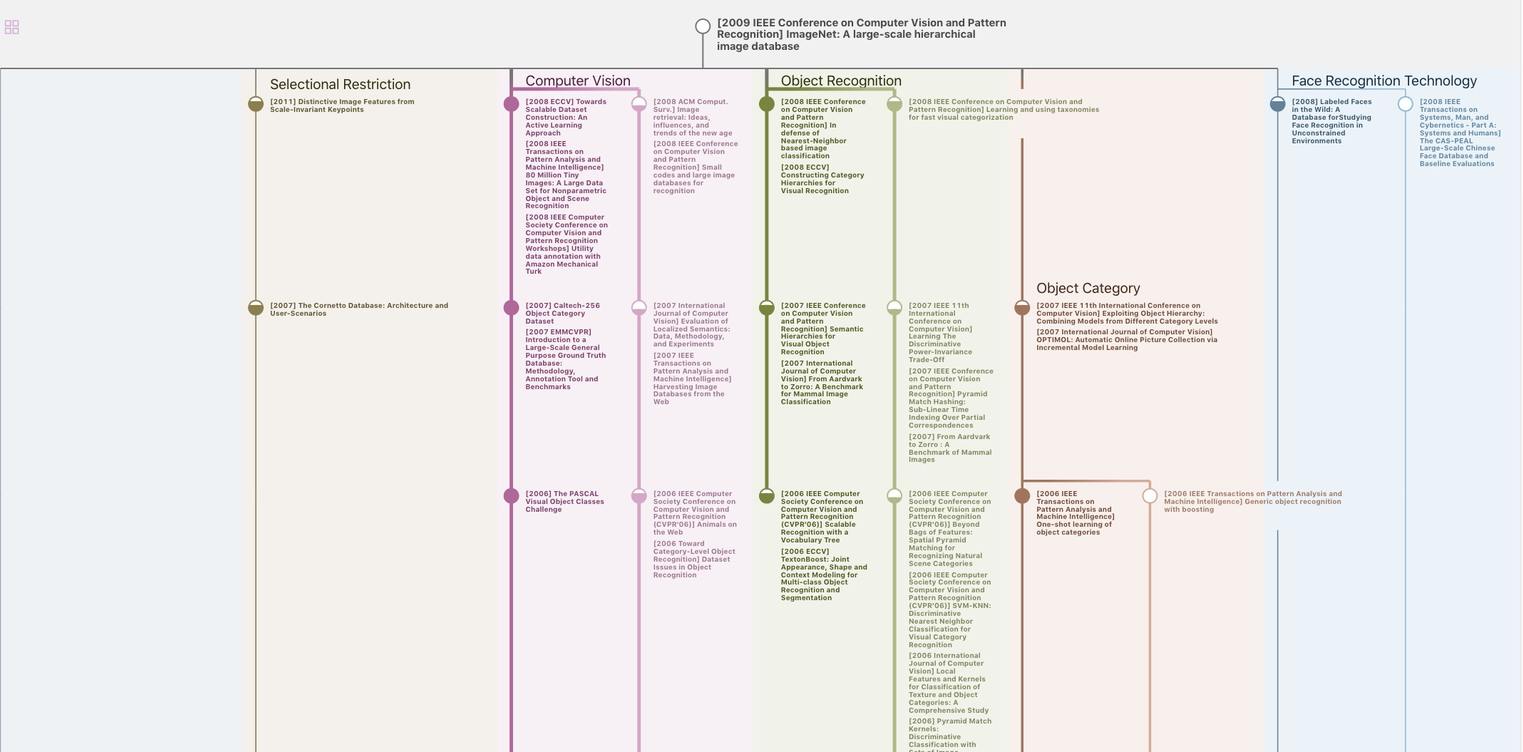
生成溯源树,研究论文发展脉络
Chat Paper
正在生成论文摘要